Synthetic Data for CLIP Training (08/20)
Quality and Legal Issues
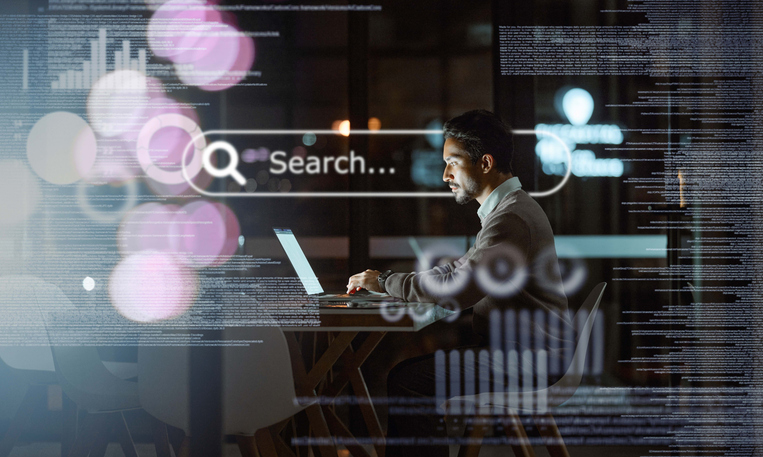
Recent advancements in AI technology, particularly with multimodal models like CLIP, have highlighted the need for large-scale image-text datasets. Traditionally, these datasets are sourced from the web, but this approach comes with several issues.
Firstly, data quality is a major concern. Web-sourced data often suffers from poor alignment between images and captions. For instance, captions may describe something entirely unrelated to the image, causing noise in model training and negatively affecting performance.
Legal issues are also becoming increasingly important. In 2023, several artists filed lawsuits claiming that their copyrighted works were used without permission for training AI models. This highlights ethical and legal concerns associated with using web data. Additionally, there is a risk of including inappropriate or illegal content, necessitating rigorous filtering to ensure the creation of safe and reliable AI models.
Scalability and Flexibility with Synthetic Data
Synthetic data allows for flexible generation of data tailored to specific domains or scenarios, enabling rapid training of AI models for various applications. For example, data can be quickly generated for new technologies or industry environments, facilitating the development of specialized models.
Recent advancements in large language models like ChatGPT have demonstrated the capability to generate natural, contextually relevant text. This, combined with image generation models, opens up the possibility of creating comprehensive synthetic datasets. These datasets can effectively replace traditional web-sourced data, offering a controlled and adaptable solution for AI training.
CLIP Training with Synthetic Data
The process of training CLIP using synthetic data involves several key steps. Initially, large language models (LLMs) are used to generate captions based on specific concepts. For instance, a caption might describe “a person relaxing in a mountain setting.” These captions are then paired with images generated through models like Stable Diffusion.
The resulting synthetic image-text pairs are used to train the CLIP model, refining both image and text encoders through contrastive learning. This approach has shown that CLIP models can be effectively trained using entirely synthetic data. Several studies have already demonstrated that synthetic data can achieve performance levels similar to those of models trained on real-world data.
Applications and Future Directions
AI models powered by synthetic data hold significant potential across various fields. In the healthcare sector, where data privacy is crucial, synthetic data offers a promising solution. By generating realistic yet anonymized medical data, AI models can be trained without compromising patient confidentiality. This could advance diagnostic tools and personalized treatment plans.
In social media and content creation, synthetic data can transform targeted marketing and user experience customization. Many companies are exploring the use of AI to generate social media ads and marketing content. Brands can automatically create content that aligns with their image and tone, enhancing marketing efficiency.
Future research will focus on improving the quality and developing models that perform comparably to real-world data across diverse domains. As synthetic data generation technologies advance, the performance gap between synthetic and real data will continue to narrow, unlocking the potential for more ethical, scalable, and adaptable AI systems.