Why Synthetic Data Is Essential for RAG AI Training? (01/08)
Intro: The Need for Data in RAG AI Models
Retrieval-Augmented Generation (RAG) AI models are at the forefront of innovation, combining retrieval-based and generative AI capabilities to deliver highly contextual responses. However, like all AI models, their performance is directly tied to the quality and diversity of the datasets used for training. Unfortunately, acquiring such datasets is easier said than done due to privacy regulations, ethical concerns, and the sheer cost of obtaining and annotating real-world data.
Challenges: A Case Study in Healthcare
Consider the case of a healthcare provider attempting to train a RAG model to assist doctors in generating patient-specific recommendations. Despite the potential benefits, the institution faced a significant roadblock: compliance with the Health Insurance Portability and Accountability Act (HIPAA). Strict regulations around patient data limited access to the real-world information needed to train the model effectively. Without a way to bridge this gap, the organization risked deploying a model that was either undertrained or biased.
Enter Synthetic Data: The Game-Changer
Synthetic data offers a practical and scalable solution to these challenges. By generating realistic yet entirely artificial datasets, organizations can train their models without compromising privacy or compliance. Unlike traditional anonymization techniques, which may still carry risks of re-identification, synthetic data ensures no actual personal information is included, aligning perfectly with regulations like HIPAA, GDPR, and beyond.
How Cubig’s Solutions Lead the Way
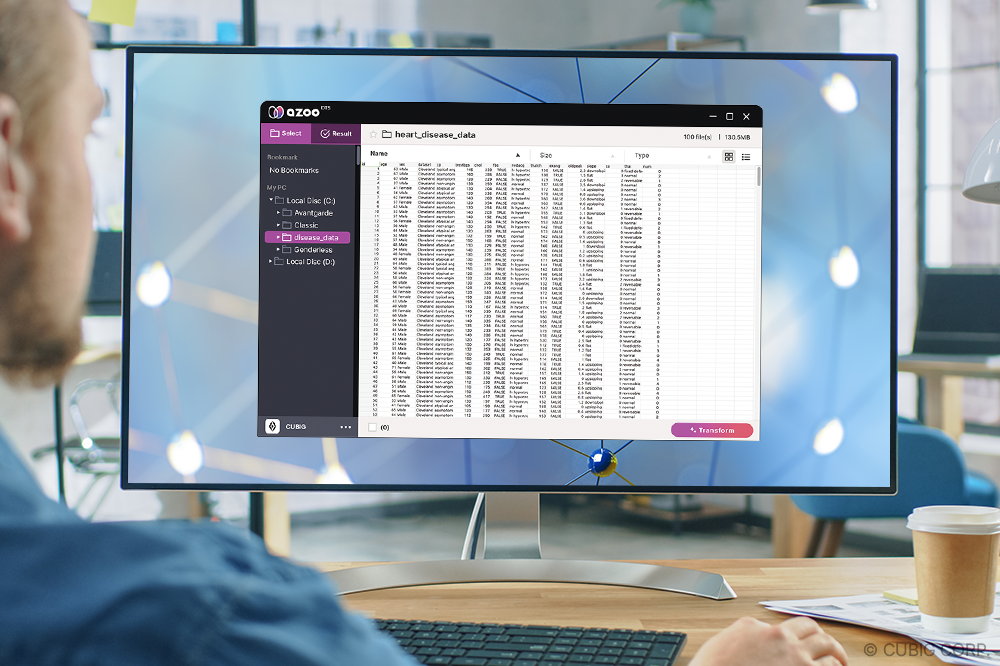
Cubig, a pioneer in synthetic data solutions, provides tools specifically designed to address the needs of industries like healthcare, finance, and public services. Their DTS (Data Transform System) and SynFlow platforms allow users to create high-fidelity synthetic datasets that mirror the statistical properties of real-world data while maintaining complete privacy compliance.
For instance, a healthcare organization can leverage Cubig’s solutions to:
- Generate patient-like data reflecting real-world distributions.
- Incorporate differential privacy techniques to ensure robust data security.
- Train RAG models effectively without regulatory concerns.
The result? A high-performing RAG AI system capable of delivering personalized recommendations or insights, all while safeguarding sensitive information.
Applications Across Industries
Synthetic data isn’t just for healthcare. Industries such as finance, retail, and manufacturing can benefit immensely. In financial services, synthetic transaction data can enhance fraud detection systems. In retail, synthetic consumer behavior data can drive better personalization strategies. The applications are as broad as they are impactful.
RAG AI models hold tremendous potential to transform industries, but they require a strong foundation of high-quality data. Synthetic data provides that foundation while overcoming the traditional limitations of data access and privacy concerns. Companies like Cubig are leading the charge, enabling businesses to harness the full power of AI without compromising ethical or regulatory standards.
By embracing synthetic data, we can build AI systems that are not only powerful and efficient but also responsible and compliant, paving the way for a smarter, safer future.
CUBIG's Service Line
Recommended Posts