Should Generative AI Development and Usage Proceed Without Any Security Measures? The Answer is “No.” (6/13)
Table of Contents
In recent years, Generative AI, especially those utilizing deep learning techniques, has achieved remarkable innovations in various fields such as image generation, text writing, and music composition. However, since these Generative models ultimately learn from actual data and generate content based on it, they can cause significant issues like privacy violations, copyright infringements, and identity theft. Differential Privacy (DP) has emerged as a solution to these challenges. In this blog post, we will discuss why applying Differential Privacy to Generative AI is necessary and the benefits it brings.
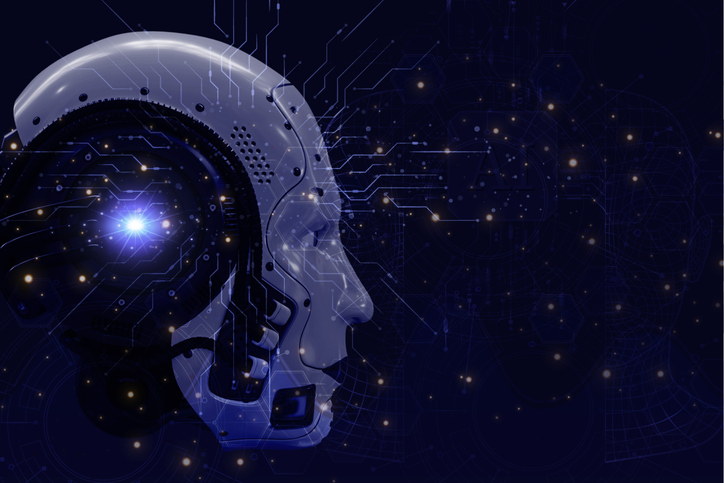
1. Privacy Risks of Generative AI
AI models learn from large datasets to create new content. During this process, the models often memorize sensitive information from the training data, which can unintentionally be included in the generated output. For instance, a large text generation model might reproduce parts of personal emails or chat logs used in training, leading to severe privacy breaches.
2. What is Differential Privacy?
Differential Privacy is a robust mathematical framework designed to protect individual data during data analysis or machine learning model training. The core concept is to minimize the impact of individual data items on the analysis results, making it nearly impossible to determine whether a specific individualβs information is included in the dataset. This ensures that even if a model has learned from specific personal data, it cannot expose that individual’s information.
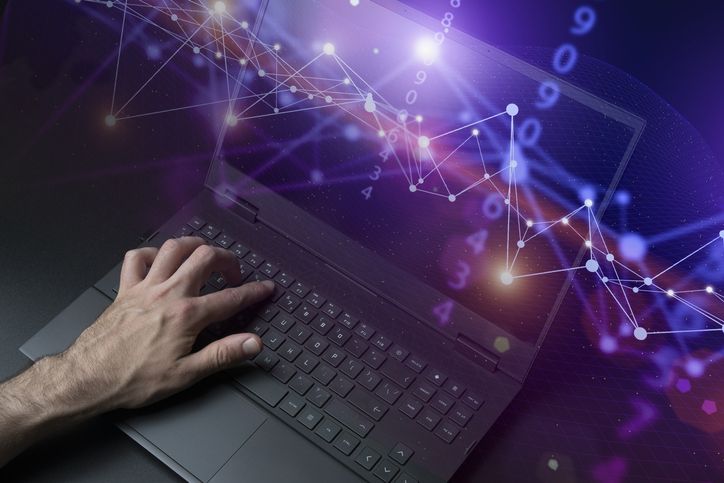
3. The Need for Applying Differential Privacy to Generative AI
3.1. Enhanced Privacy Protection
Applying Differential Privacy to Generative AI models significantly reduces the risk of sensitive information being included in the generated content. This guarantees the safety of user data and helps AI service providers comply with privacy regulations.
3.2. Increased Trust
Generative models with Differential Privacy gain higher trust from users. Users can be assured that their data is securely protected and that the generated content will not infringe on their privacy. This increased trust can lead to higher adoption rates of AI services.
3.3. Compliance with Regulations
Many countries enforce strict regulations to protect personal data. Differential Privacy is an effective method to comply with these regulations. For example, the General Data Protection Regulation (GDPR) demands high levels of data protection from companies handling personal data. By applying Differential Privacy to Generative AI models, these legal requirements can be met.
3.4. Improved Generative AI Model
Differential Privacy prevents model overfitting, ensuring that models rely less on the training data and generalize better to new data. This enhances the quality of generated content, making it more useful across various contexts.
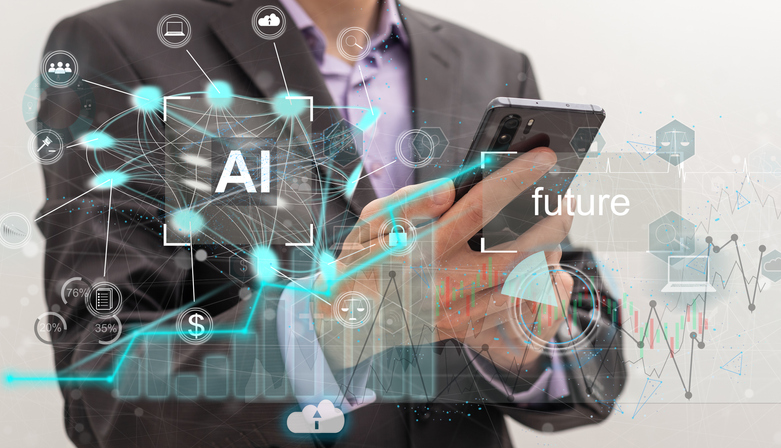
Conclusion
Generative AI is a powerful tool that can transform our lives, but it also raises critical privacy and security concerns. Differential Privacy offers an effective solution to these issues. By integrating Differential Privacy into Generative AI models, we can enjoy benefits such as enhanced privacy protection, increased trust, regulatory compliance, and improved model performance. We look forward to seeing more Generative AI models adopting Differential Privacy, providing users with safe and trustworthy AI services.
We generate data safely and there are sites that sell such data. If you’re interested, please visit.
AZOO Link: https://azoo.ai/
If you are curious about CUBIG, the company that created AZOO and runs this blog, click the link below to find out more.
Company Link: https://cubig.ai/
If you are interested in various topics about AI and its security, we would appreciate it if you explore our blog further.
Blog Link: https://azoo.ai/blogs/