Enhancing Data Security with Synthetic Data: Overcoming the Limitations of De-Identification (5/30)

Ensuring privacy while maintaining data utility is a significant challenge. Traditional de-identification methods have been used to protect personal information, but they often fall short. Synthetic data can be greate alternative that addresses these shortcomings effectively. This article explores the limitations of de-identification and highlights the benefits of synthetic data.
The Limitations of De-Identification
De-identification involves modifying personal data to prevent the identification of individuals. This is typically achieved by removing or masking direct and indirect identifiers. However, this approach has several significant limitations:
- Residual Risk of Re-Identification: Despite efforts to anonymize data, there is always a risk that de-identified data can be re-identified through sophisticated techniques. For instance, when combined with other datasets, even data that has been de-identified can sometimes be traced back to individuals. Appropriately de-identified pseudonymized data may still carry identification risks and remain subject to data protection regulations.
- Reduced Data Utility: High levels of de-identification can severely reduce the utility of the data. The more de-identified the data, the less useful it becomes for meaningful analysis and decision-making. Anonymized data, while safer, often lacks the detail necessary for comprehensive data analysis, thus limiting its effectiveness and applicability.
- Compliance Challenges: De-identified data still needs to comply with various legal and regulatory frameworks, which can be complex and burdensome. Ensuring that data is sufficiently de-identified to meet all relevant regulations while retaining its utility is a challenging balancing act for organizations.
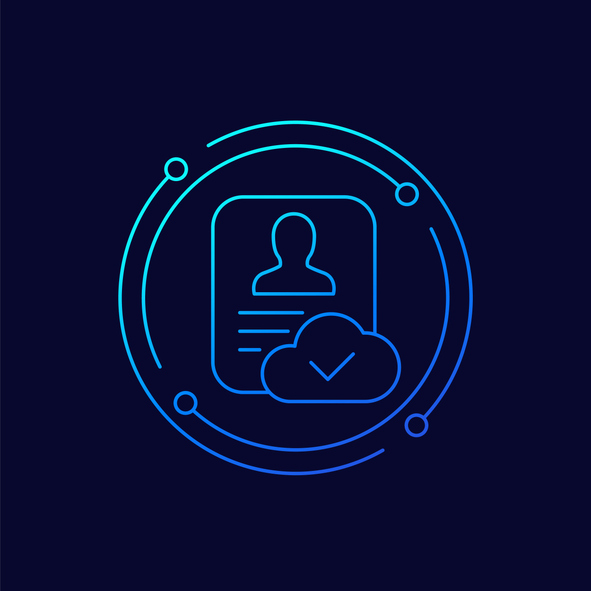
The Promise of Synthetic Data
Synthetic data is created artificially to mirror the statistical properties of real data without containing any actual personal information. This innovative approach offers several key advantages:
- Enhanced Privacy: Since synthetic data does not include real personal information, it inherently eliminates the risk of re-identification. This makes it a safer option for a variety of applications where privacy is a critical concern. Properly generated synthetic data ensures that individuals cannot be identified, even if the data is analyzed alongside other datasets.
- Maintaining Data Utility: Synthetic data retains the essential statistical properties of the original dataset, ensuring that it remains useful for analysis, machine learning, and other data-driven tasks. This balance between privacy and utility makes it extremely valuable for organizations seeking to maximize the usefulness of their data while protecting privacy.
- Cost-Effectiveness: Generating synthetic data can be significantly more cost-effective than collecting and de-identifying real data. For example, creating synthetic images or other data types is often much cheaper than acquiring real ones, which can substantially reduce costs for large-scale data projects.
- Regulatory Compliance: Synthetic data is generally not subject to the same stringent regulations as real personal data, simplifying compliance and enabling more flexible use across different jurisdictions. This ease of compliance facilitates data sharing and collaboration without the legal complexities typically associated with real data.
Applications and Future Prospects
Synthetic data has a wide range of applications across various domains, including healthcare, public safety, retail, and finance. For instance, in healthcare, synthetic data can be used to develop AI models for disease diagnosis without compromising patient privacy. Similarly, in the finance sector, it can help create robust credit scoring models while protecting sensitive customer information.

Conclusion
While de-identification has been a standard approach to data privacy, its limitations necessitate alternative solutions. Synthetic data offers a promising way to enhance data security and utility, ensuring privacy without sacrificing the richness of information needed for advanced analytics. As technology continues to evolve, it is set to become a cornerstone of secure and effective data management practices.
Azoo’s Commitment to Quality and Security
At Azoo, we are at the forefront of synthetic data innovation. By applying differential privacy techniques, we ensure our synthetic data is both highly secure and of the highest quality. This allows organizations to confidently use and share data without compromising privacy or utility. Explore Azoo’s synthetic data solutions to see how we can help you meet your data needs securely and efficiently.