Free AI Datasets: The Power of Use and Dangers of Unverified Sources (9/12)
AI research and development heavily rely on vast amounts of training data, and free AI datasets play a crucial role in facilitating advancements in the field. These open-source datasets enables researchers and developers to experiment, prototype, and refine their models without incurring significant costs. However, while the availability of free datasets is essential for AI’s growth, there are substantial risks when using datasets from unverified or unknown sources. These risks range from security threats like poisoning attacks to ethical concerns around bias and fairness.
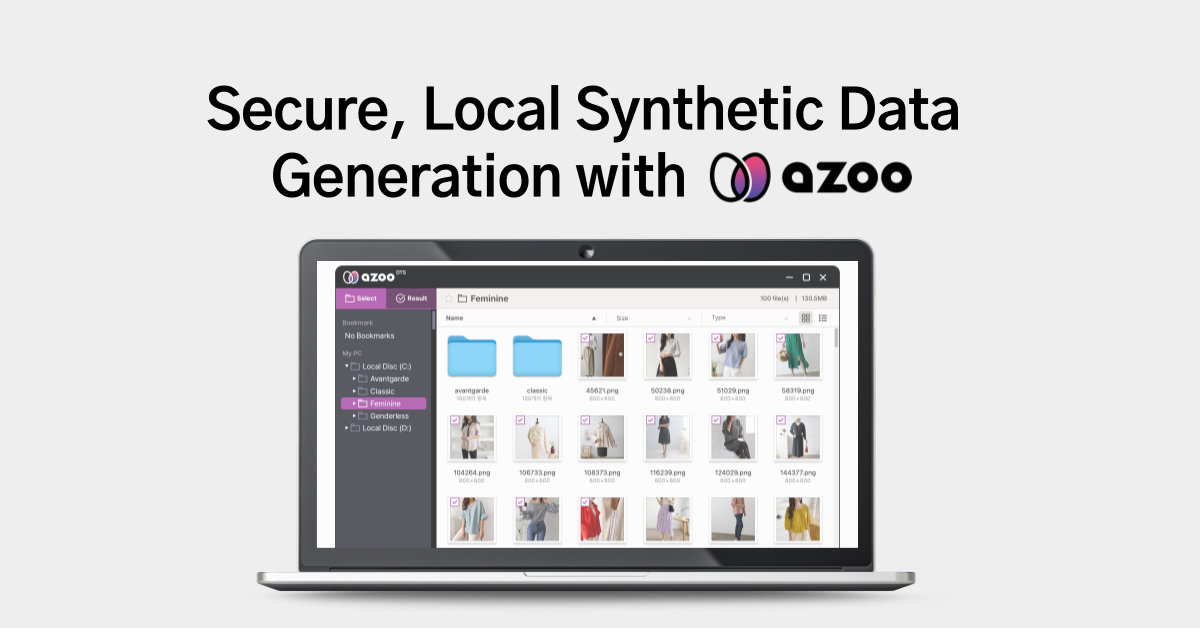
Free AI Datasets Might Corrupt Models
Poisoning Attacks
One of the most pressing dangers of using unverified datasets is the potential for poisoning attacks. In a poisoning attack, a malicious actor intentionally injects harmful or misleading data into a training dataset. When a model is trained on this compromised data, its ability to perform correctly is undermined. The model might produce inaccurate predictions, make biased decisions, or even behave unpredictably under certain conditions.
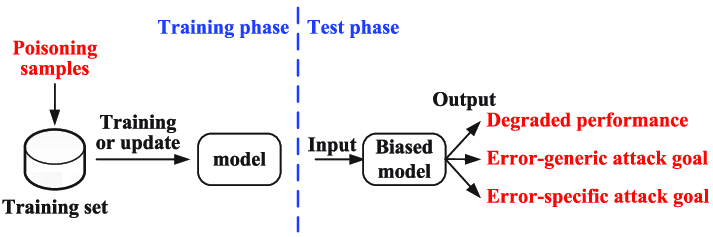
For instance, a facial recognition model trained on poisoned data might wrongly classify certain individuals or fail to recognize them altogether. This could have severe consequences in security, healthcare, or legal applications where accuracy is paramount. Poisoning attacks are difficult to detect and can cause long-lasting damage to the integrity of an AI system.
Data Bias
Another major concern with using unverified datasets is the possibility of data bias. AI systems learn from the data they’re given, and if that data is biased, the resulting models will be biased as well. Many free datasets available online may not have undergone proper vetting to ensure they are diverse, inclusive, or representative of the real-world populations they aim to model.

For example, a machine learning model trained on biased data in a hiring algorithm could end up favoring certain demographics while discriminating against others. This could reinforce societal inequalities and perpetuate systemic discrimination, going against the ethical standards that AI development should strive for.
Quality Control
Free AI datasets often lack the rigorous quality control found in proprietary datasets. Data quality is critical for training reliable models, and poor-quality data can severely impair model performance. Unverified datasets may contain errors, missing values, or inconsistencies that can negatively affect training outcomes.
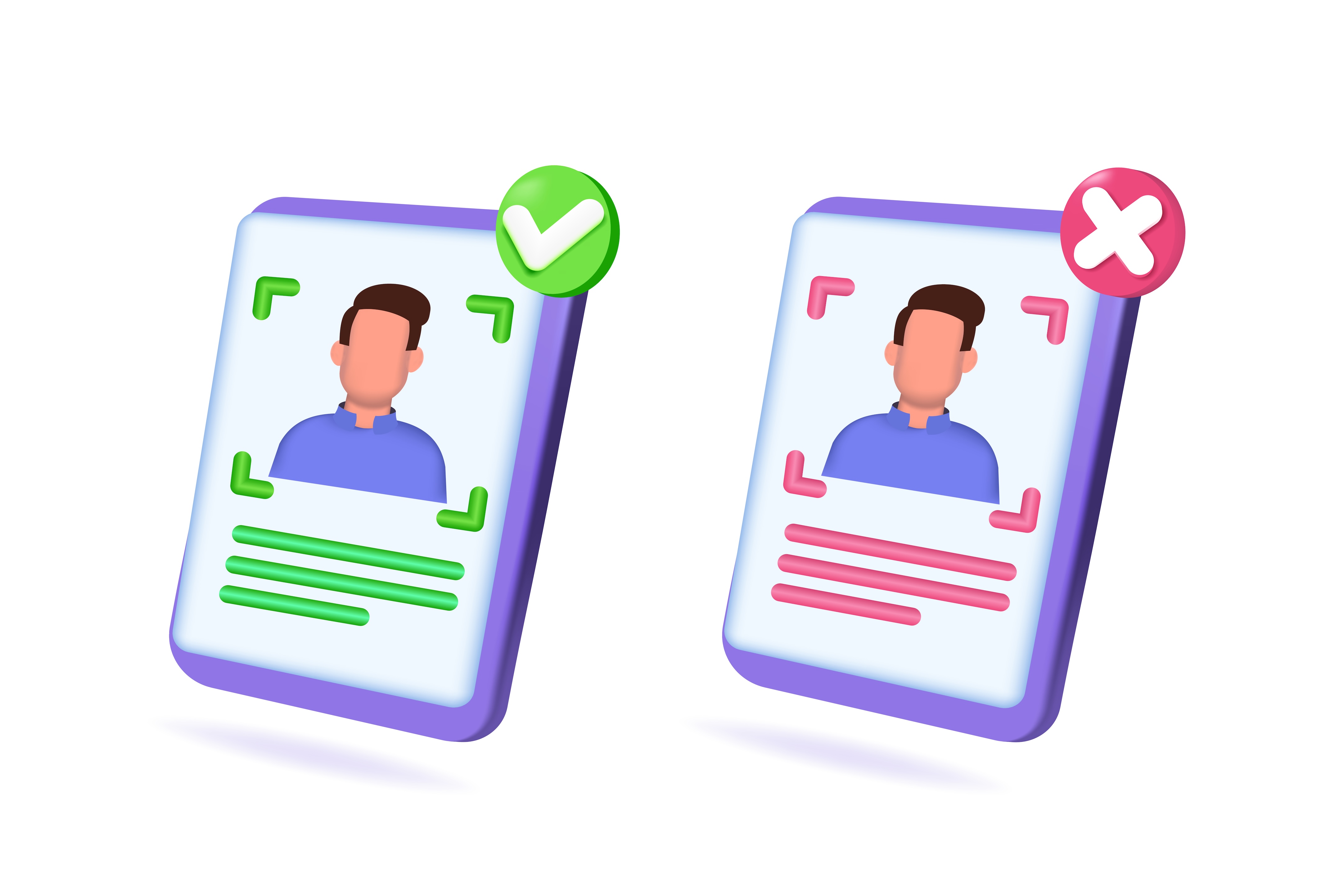
Without proper validation, a dataset might include irrelevant or redundant information, which can introduce noise and reduce the effectiveness of the model. Models trained on poor-quality data will struggle to generalize, leading to incorrect predictions when applied to real-world scenarios.
Are All Free AI Datasets Bad?
No. Absolutely not all free AI datasets are bad. Many reputable organizations, research institutions, and universities provided high-quality, well-curated datasets that are essential for advancing AI research. These datasets often undergo rigorous vetting processes to ensure they are diverse, ethically sourced, and reliable.

Particularly, platforms like azoo, which function as a data marketplace, which function as a trusted data marketplace, provide high-quality and vetted datasets. Azoo ensures that the datasets available on its platform are reliable, ethically sourced, and free from the risks often associated with unverified data. By maintaining rigorous standards for data integrity and transparency, azoo allows researchers and developers to confidently utilize its resources without the typical concerns related to data quality, bias, or security vulnerabilities.