Harnessing User-Level Differential Privacy: Strengthening User-Centric Security (9/15)
Table of Contents
As privacy concerns grow in the data world, differential privacy (DP) is becoming a key tool for ensuring that sensitive information is protected while still enabling valuable analysis. A newer concept within this space is User-Level Differential Privacy (DP), which offers an alternative approach to protecting privacy when users contribute multiple data points to a dataset.
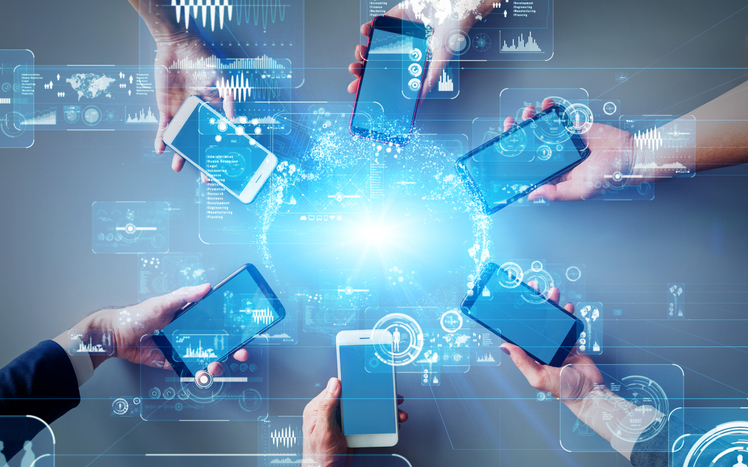
What is User-Level Differential Privacy?
In traditional differential privacy, each individual data point is protected. However, User-Level DP focuses on ensuring the privacy of the entire set of data points contributed by a single user. This approach is especially relevant when users provide multiple entries to a dataset over time, such as fitness tracker data or transaction histories.
For instance, letโs say a fitness tracking app collects a userโs step count, heart rate, and workout details over several weeks. User-level DP would ensure that all of this data, taken together, is protected, making it harder to infer details about the user even if someone had access to the dataset.
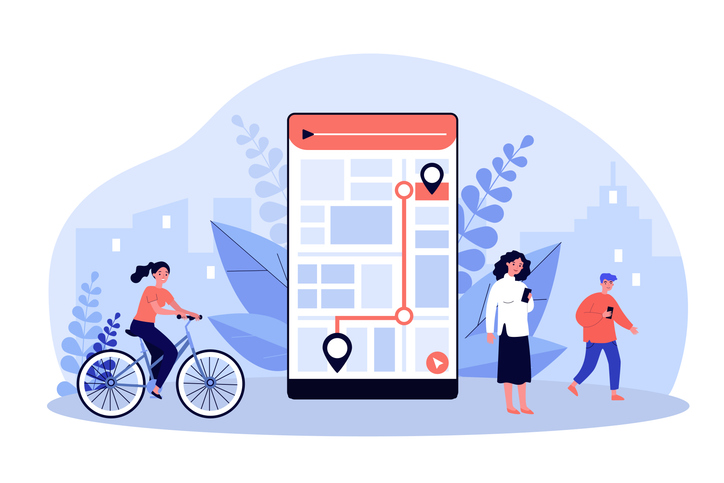
How Does User-Level DP Differ from Standard DP?
- Data Aggregation Focus: While traditional DP protects individual entries, user-level DP looks at the user as a whole. This can be useful in cases where users contribute multiple records, as it offers protection across their entire dataset.
- Use Case Sensitivity: User-level DP doesnโt necessarily replace item-level DP but complements it depending on the use case. Itโs particularly beneficial for scenarios where datasets contain correlated data points from the same individual, such as in health monitoring or financial transactions.
- No One-Size-Fits-All: There isn’t a definitive answer to whether user-level DP is better or worse than item-level DPโit all depends on the nature of the data and the desired privacy guarantees. In some situations, item-level DP provides sufficient protection, while in others, user-level DP may offer a more suitable approach to safeguarding personal information.
Why Is User-Level DP Interesting?
User-level DP brings a different perspective to privacy protection that researchers and practitioners find compelling. For datasets where users contribute multiple, related data points, user-level DP ensures that privacy is maintained across the board, even when those records are linked.
However, like all privacy mechanisms, there are trade-offs. User-level DP may require more careful tuning to balance privacy and data utility, especially when working with smaller datasets. The amount of noise added to the data still depends on the privacy guarantees needed, and maintaining the balance between noise and data accuracy remains a central challenge.
The Role of Differential Privacy at Cubig
At Cubig, we are dedicated to pushing the boundaries of privacy-preserving technologies. One key tool in our toolbox is differential privacy, which we incorporate into our synthetic data solutions, such as our DTS (Data Transformation System). This allows us to help clients achieve the highest levels of data privacy, ensuring that sensitive information remains protected while still allowing meaningful insights and analysis.
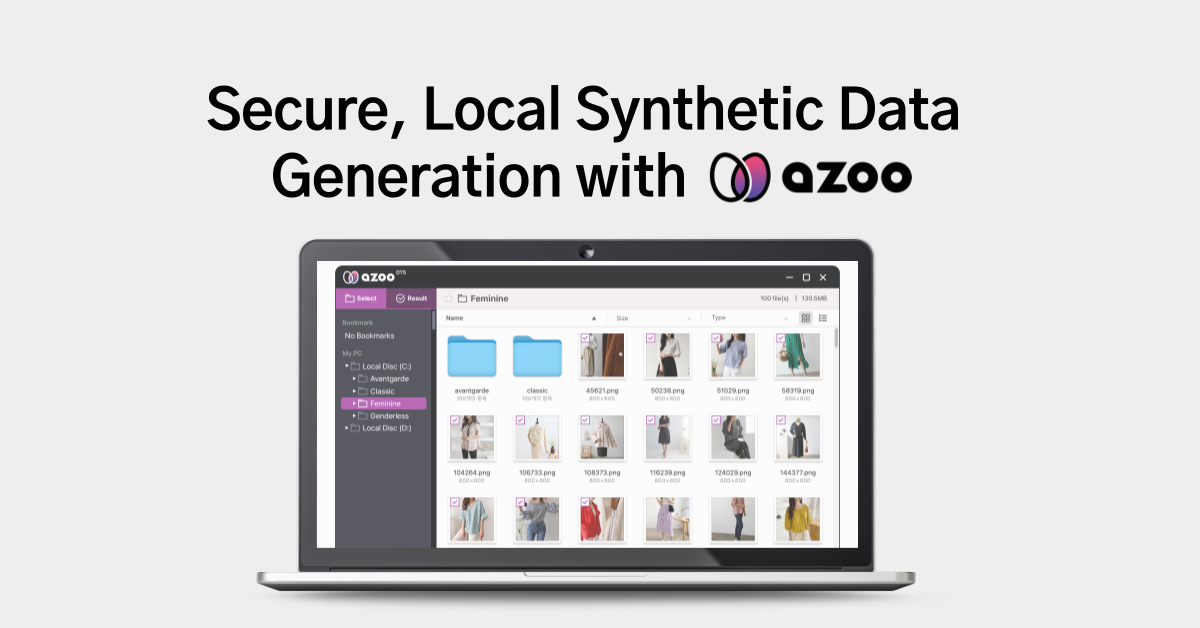
Looking Ahead
Differential privacy continues to evolve rapidly, with innovations like user-level DP offering new possibilities for enhanced privacy protection. Whether itโs applied in healthcare, finance, or research, understanding the wide range of privacy tools available can help organizations protect sensitive data without compromising on the quality of their analysis.