Safeguard Your Secrets While Training AI: Differentially Private Synthetic Data to the Rescue (6/7)
Table of Contents
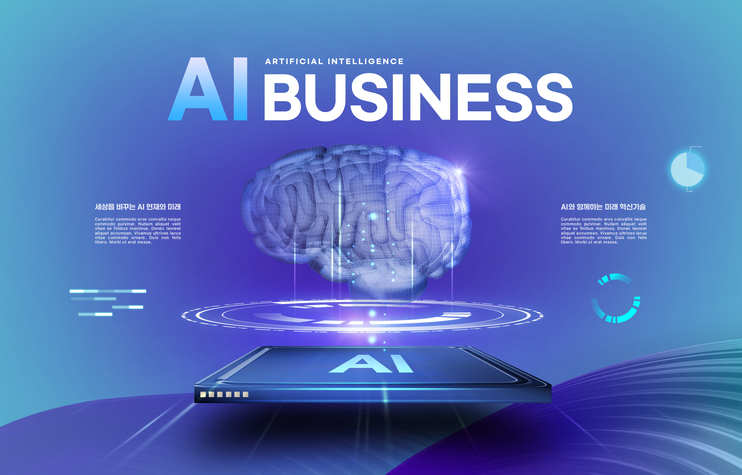
The Paradox of Data Utilization
Companies across various sectors, including healthcare, finance, and legal services, recognize the potential of their data to drive innovation and improve decision-making through machine learning (ML) and artificial intelligence (AI). Yet, the necessity to use real, sensitive data for training these models often leads to a paradox: the desire to leverage data effectively while simultaneously ensuring its security and confidentiality.
Differential Privacy: A Protective Shield
Differential privacy (DP) is a groundbreaking approach that provides robust privacy guarantees. It ensures that the inclusion or exclusion of any single data point does not significantly affect the outcome of any analysis, thereby protecting individual privacy. When used to generate synthetic data, differential privacy enables the creation of datasets that reflect the statistical properties of the original data without revealing any actual confidential information.
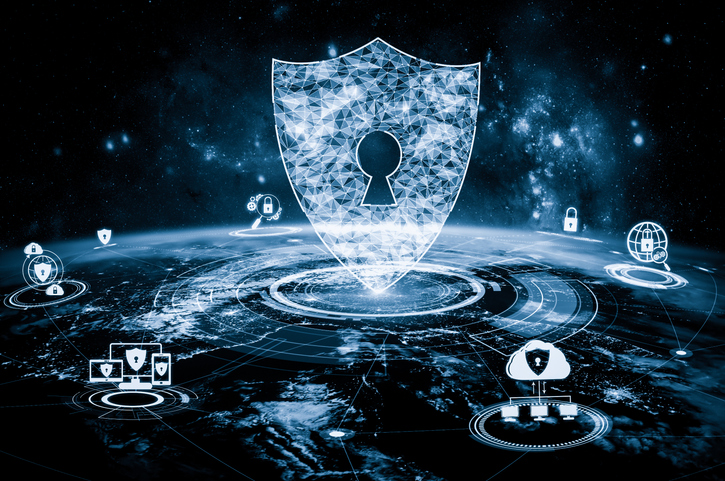
Why Choose Differentially Private Synthetic Data?
- Uncompromised Privacy: Differentially private synthetic data ensures that no real personal information is included, drastically reducing the risk of data breaches and ensuring compliance with regulations like GDPR and CCPA.
- Enhanced Collaboration: Synthetic data can be freely shared within the organization or with third parties, facilitating collaboration without complex data-sharing agreements or anonymization processes.
- Bias Mitigation: By balancing out biases present in real datasets, synthetic data helps in developing fairer and more equitable AI models.
- Efficiency: Generating synthetic data is often quicker and more cost-effective than collecting and labeling new datasets, especially for rare or difficult-to-capture scenarios which is called tailed-data.
Conclusion
Differentially private synthetic data empowers organizations to harness the full potential of their data for machine learning without compromising on privacy. This approach not only enhances data security but also promotes the development of robust and unbiased AI models. Embrace differentially private synthetic data to protect your valuable insights while maintaining the highest standards of data confidentiality.
In azoo, you can make your own differential privacy synthetic data without process of uploading your confidential data to our server. You can safely make your own secure data which can treat as public data because of the differential privacy . If you have an interest, please visit our blogs or contact us please.
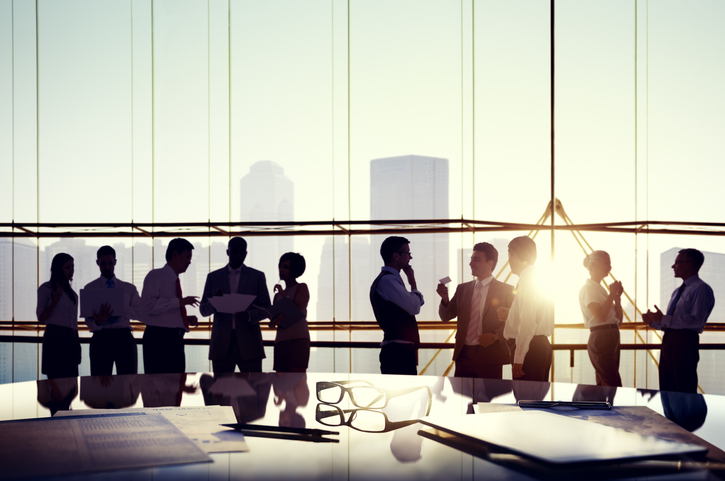
azoo Platform
cubig website
By adopting differentially private synthetic data, your organization can confidently advance its data initiatives, ensuring the security and confidentiality of sensitive information while still driving innovation.
Thank you!