Opening the Black Box of AI: The Importance of Explainable AI (06/05)
Table of Contents
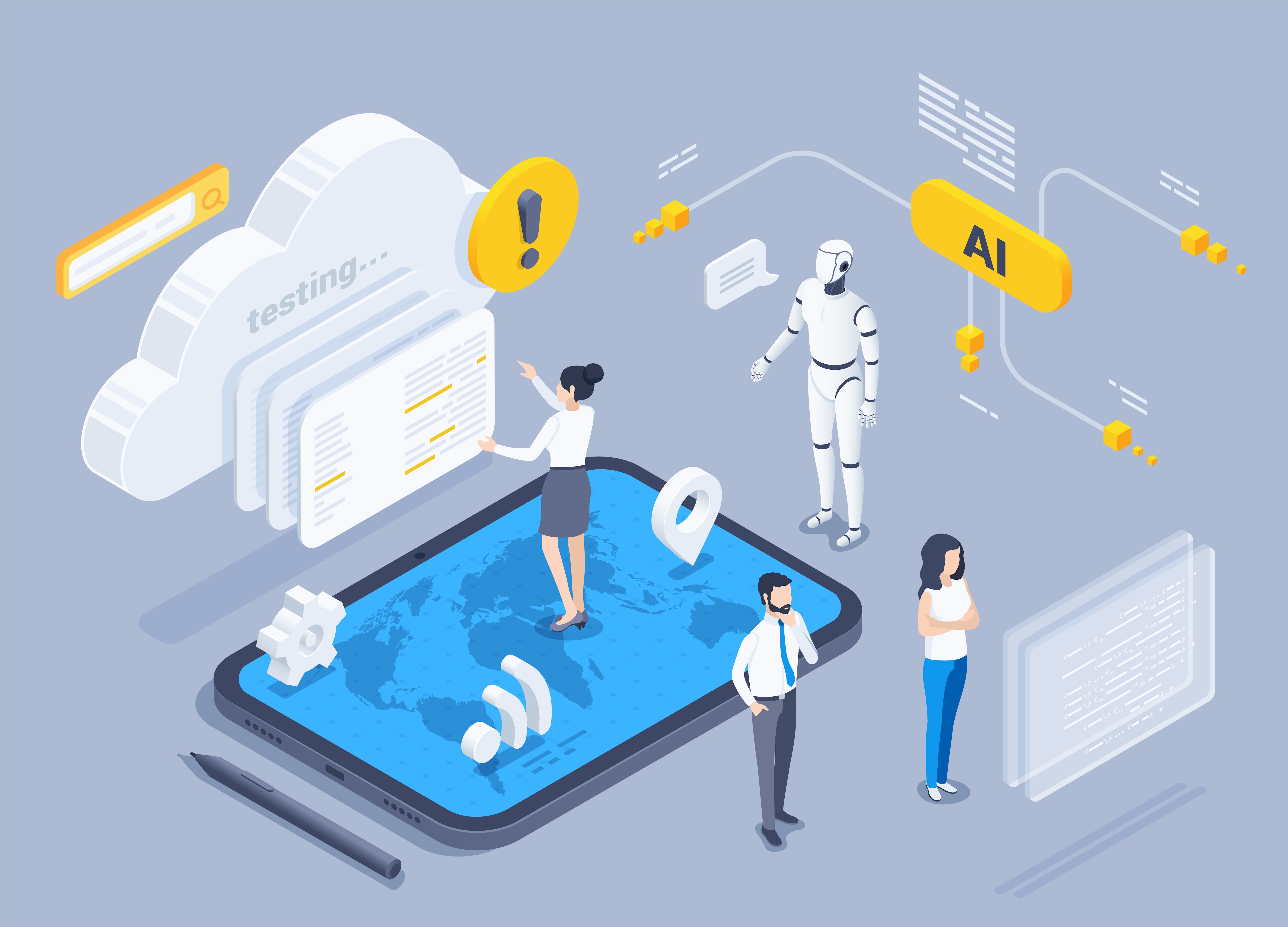
What is Explainable AI?
As artificial intelligence (AI) becomes deeply embedded in our daily lives and industries, the need to understand and explain AI systems’ decision-making processes is growing. “Explainable AI” (XAI) is a concept developed to meet this demand, playing a crucial role in enhancing AI’s transparency and trustworthiness.
AI systems, especially deep learning models, are often referred to as ‘black boxes.’ This term highlights the difficulty in clearly understanding how these models make decisions. The complex mathematical processes and multi-layered neural networks involved in making decisions are not easily understood by users. This lack of transparency can be a significant barrier to the adoption and utilization of AI. For instance, consider an AI model diagnosing a specific disease in the medical field. In a ‘black box’ situation, users may not understand the rationale behind the model’s diagnosis, making it hard for doctors or patients to trust the results.
Explainable AI includes technologies and methodologies designed to address these issues. The goal of XAI is to explain the reasons behind AI model decisions in a way that humans can understand. This approach enhances AI transparency, helps users trust AI decisions, and provides opportunities to correct or improve erroneous judgments. Moreover, it encourages more proactive use of AI.
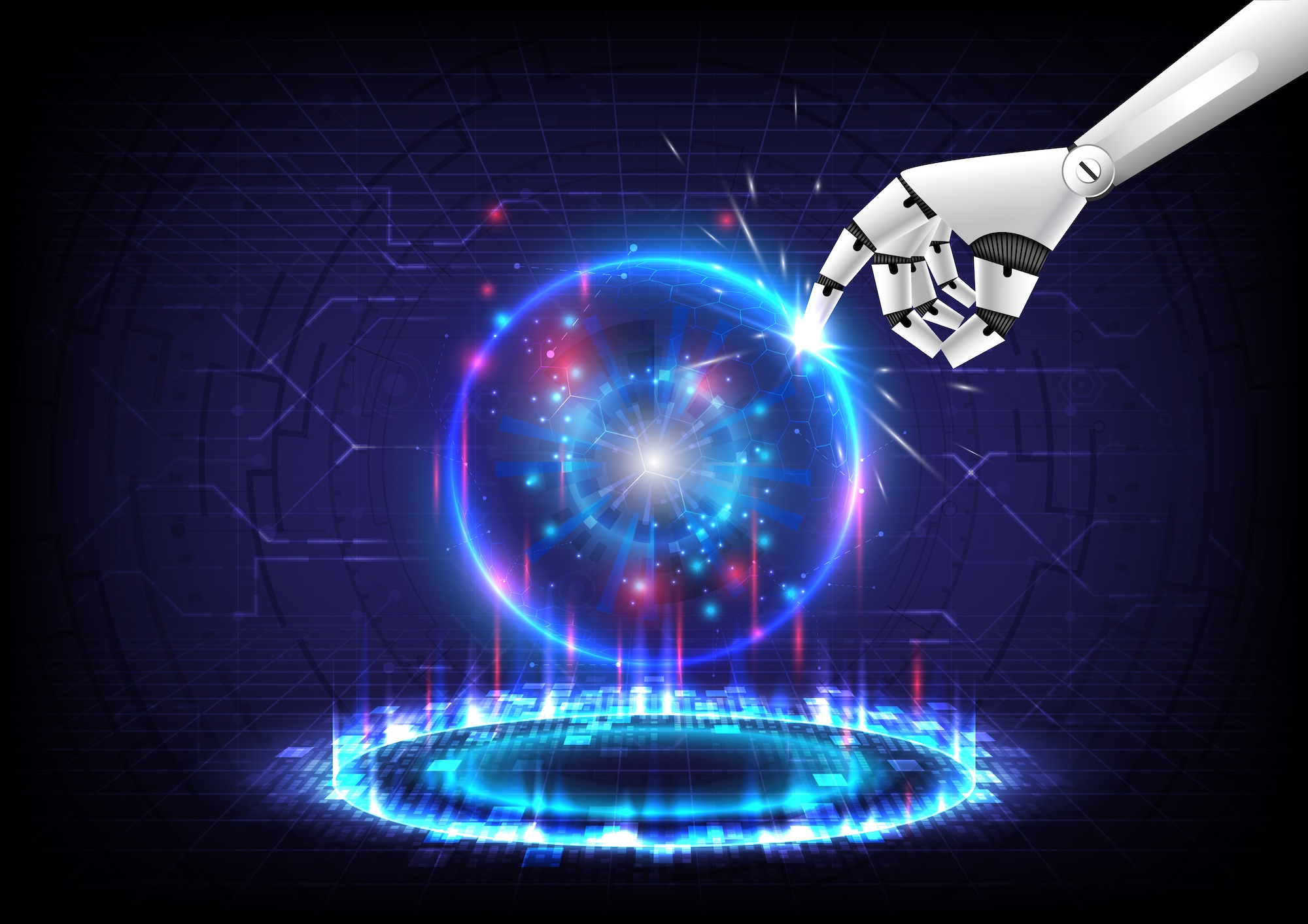
The importance of explainable AI is highlighted in several aspects:
1. Ethical Aspects
In ethical terms, explainable AI helps prevent AI systems from making biased or discriminatory decisions. Understanding why AI made a particular decision allows for checking if the decision was based on biased data and making necessary corrections. This is essential for building fair AI systems.
2. Regulatory Compliance
Explainable AI is also crucial for regulatory compliance. Many countries and organizations have introduced legal regulations demanding AI systems to be transparent and accountable. For example, the European Union’s GDPR specifies the right to an explanation for automated decisions. Therefore, XAI is essential for meeting these regulatory requirements.
3. Business Aspects
In terms of business trustworthiness, explainable AI plays a vital role in gaining customer trust. In fields such as financial services or healthcare, where AI decisions can have significant impacts, the ability to clearly explain the reasoning behind these decisions is crucial for earning customer trust.
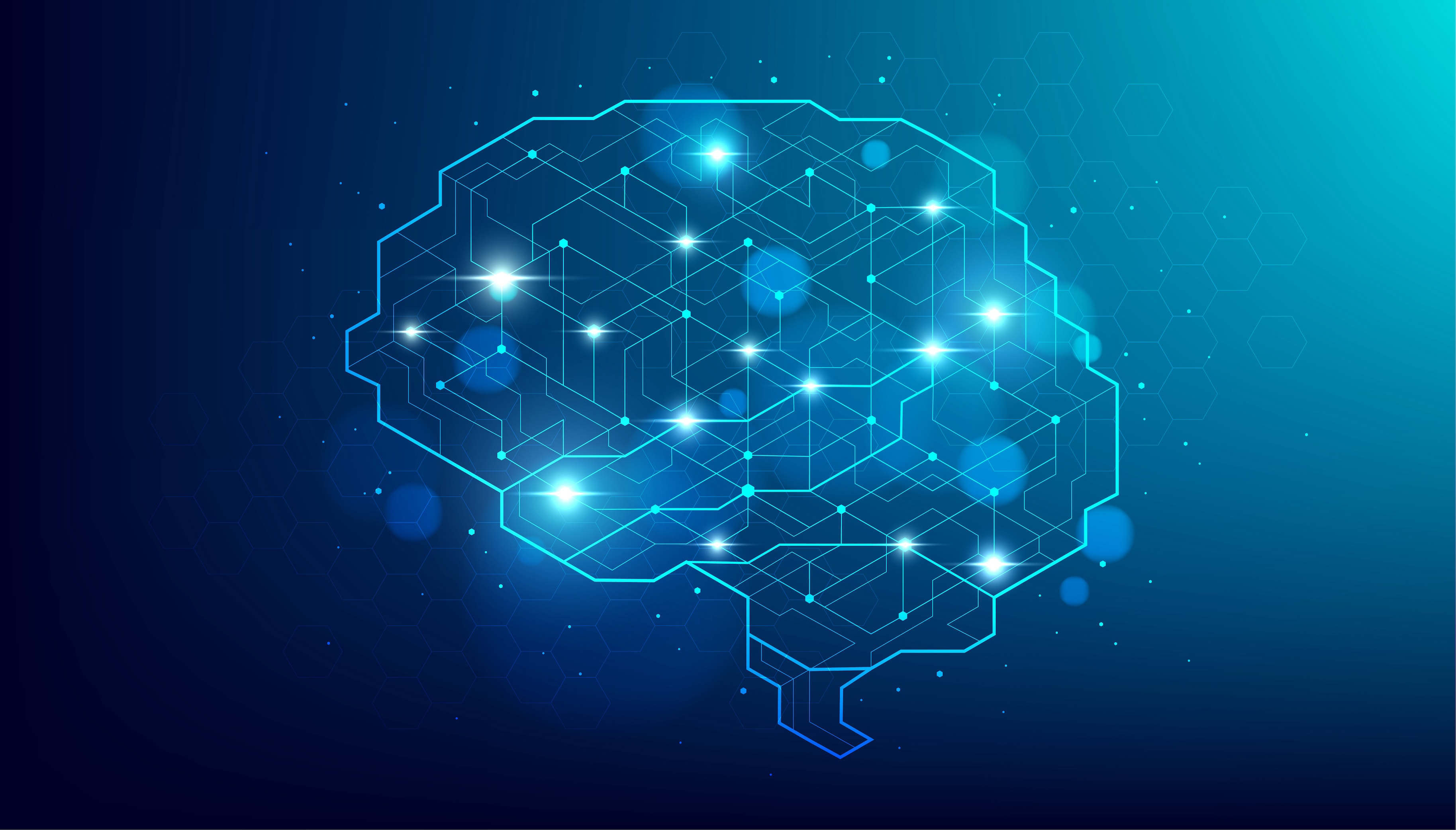
Conclusion
There are various methods to implement Explainable AI. A typical approach is simplifying the model structure to make it easier for humans to understand. However, since complex models are often used in practice, auxiliary models to explain decisions are also employed. For example, decision trees or regression analysis, which are relatively easier to understand, can be used to explain complex model decisions. Other methods include using visualizations to explain data or highlighting key features that underpin specific decisions.
As AI technology continues to advance, the importance of explainable AI will grow even more. With AI systems becoming more complex and applied across various fields, building transparent and trustworthy AI is essential. Explainable AI is a crucial tool for achieving this goal, and its significance will only increase alongside AI technology’s progression. Opening the black box of AI to create understandable and trustworthy AI for everyone is our challenge.
If you want to learn more, click the links: