Supercharge Your Business with LLMs: Smart Privacy Solutions with DP-synthetic data (4/25)
Table of Contents
Introduction

Today, LLMs (Large Language Models) are frequently used to enhance company operations. LLMs can be invaluable tool for company staff, enabling them to quickly find and use necessary operational data. Also, refined response by LLMs can be used to provide accurate information to customers in contact with the company. Such capabilities of LLMs can be a significant force in enhancing company operations and growth.
However, great power comes with great responsibility. LLMs have the risk of outputting confidential data from the input without filtering it. If confidential data is scattered throughout company data, this would be a crucial issue.
This blog will explore the privacy concerns associated with language models and suggest what can you do to solve that privacy problems.
Understanding the Privacy Issues with LLMs
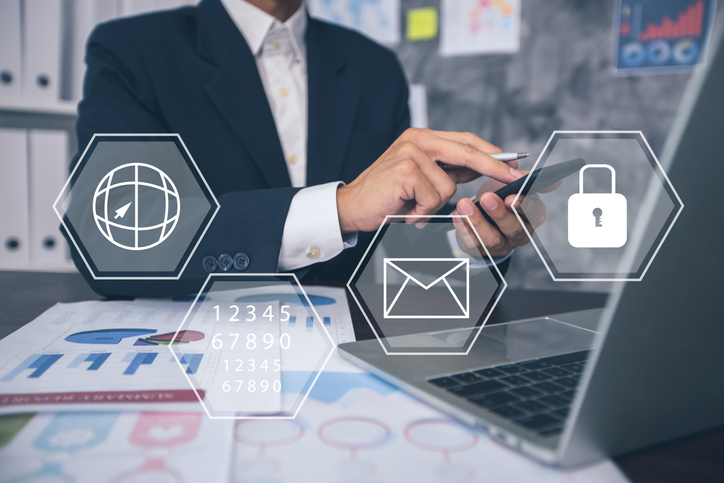
Language models are trained on vast amounts of data, some of which may contain personal information or confidential contents. This can lead to unintended consequences, such as:
- Data Leakage: Models might reproduce snippets of training data, potentially revealing sensitive information.
- Inference Attacks: Skilled individuals could manipulate the model to extract private data or infer characteristics about individuals or information.
- Bias and Fairness: Models might amplify biases present in the training data, affecting privacy and fairness.
Commonly, we can choose from the following options to solve this problems.
- Removing confidential information in Data: You can remove privacy contents in the dataset and ensure that the data used for training LLMs is free of confidential information.
- Model Filtering and Monitoring: You can continuously monitor your LLMs for any signs of privacy breaches and apply filtering techniques to prevent sensitive data leakage.
However, applying such methods requires significant time and labor resources. Also, it is challenging to implement these methods across all confidential data.
Enhancing Privacy with Technology
To further enhance LLM privacy with ease, you can apply below techniques.
- Apply Differential Privacy(DP) to LLMs: By integrating differential privacy techniques into LLMs, you can train your business data without capturing business specific data that can be traced back to an individual or confidential data.
- Use differential privacy synthetic data: You can implement differential privacy into the original business data to create synthetic data. You can train your LLMs with this data, then it will satisfy differential privacy, thus it will become safe from the risk of privacy leakage.
Both of ways can be great solution, but first way has to implement differential privacy to your LLMS internal architecture. It can be annoying and tough process to have. So, it will be more comfortable to use second way with well-made DP-synthetic data.
Conclusion
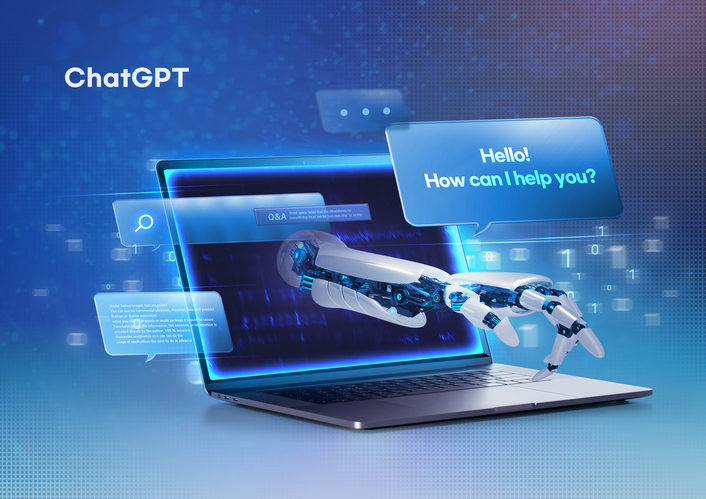
While LLMs offer hugs potential, it is imperative to address privacy concerns with the utmost seriousness. Our company can offer well-made synthetic data to train your LLM with privacy, and can eliminate your privacy concerns.
Contact to us anytime you need synthetic data with DP.
Thank you π
Do you want to get custom synthetic data with DP?
Please visit our homepage!!
Do you want to train you business own chatbot??
Visit the below link!
Do you want to know what is DP(differential privacy)?
There is great reference.