Generative AI: 2 Things You Should Know to Be Safer
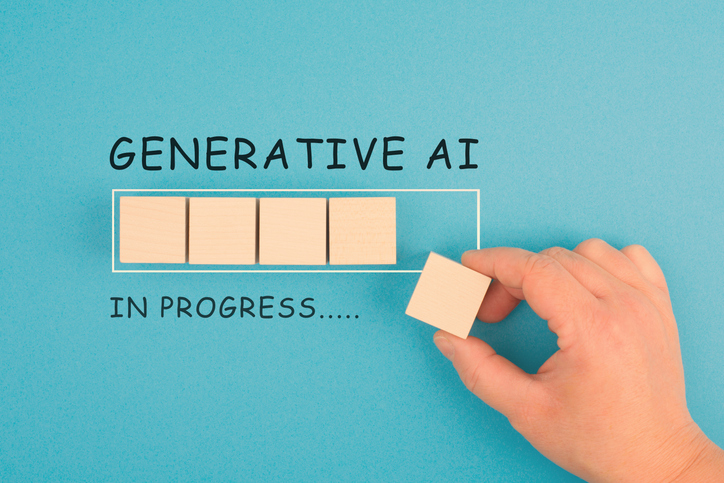
It is quite straightforward to understand what generative AI means — AI models that generate data. But you may wonder why it is more special than other AI models. If so, you should pay close attention to the story I’m going to tell here.
Understanding Generative AI
Generative AI refers to a subset of AI technologies that can generate new content, including text, images, and even music, based on the data they’ve been trained on. These models, such as GPT (Generative Pre-trained Transformer) for text and DALL-E for images, leverage vast amounts of data to learn patterns, styles, and structures, enabling them to produce original outputs when prompted.
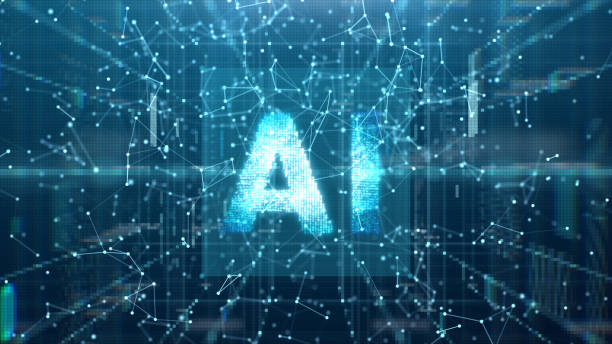
Why So Special?
Typical AI architectures focus mainly on prediction. It learns the implicit knowledge underneath a set of data it’s trained on and uses it to predict unseen data, assuming that the same rules may be applied. On the other hand, generative AI goes further than that: it generates new data itself. This requires more meticulously detailed understanding of the data distribution. You could roll a dice to potentially get a good score on multiple-choice questions. But relying on such luck won’t work for subjective questions such as writing an essay. The exact same logic holds true for AI models as well. In summary, it’s special because it requires a more complex approach.
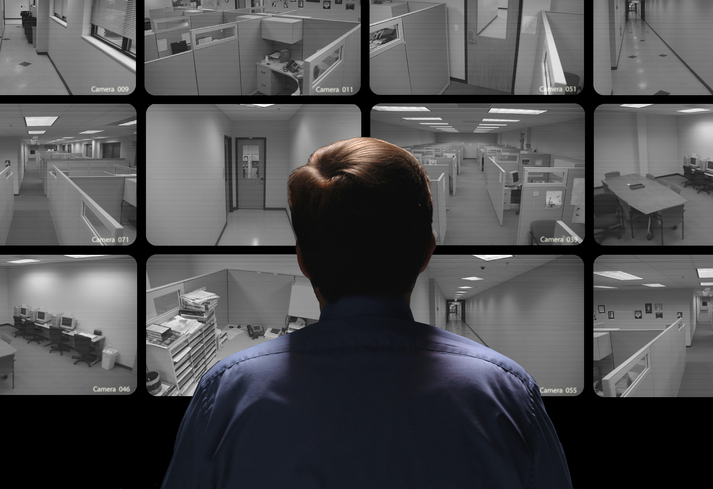
The Safety Conundrum
The thing is that, the smarter AI model is, the riskier it is. It can now even mimic your data including your credit card usage pattern, medical history, the way you walk, where you live and work, and so on and on. That’s pretty much everything needed to create your virtual self that acts exactly like you on the Internet. Even worse, it could differ from you in only a few, subtle ways that are significant enough to harm your reputation.
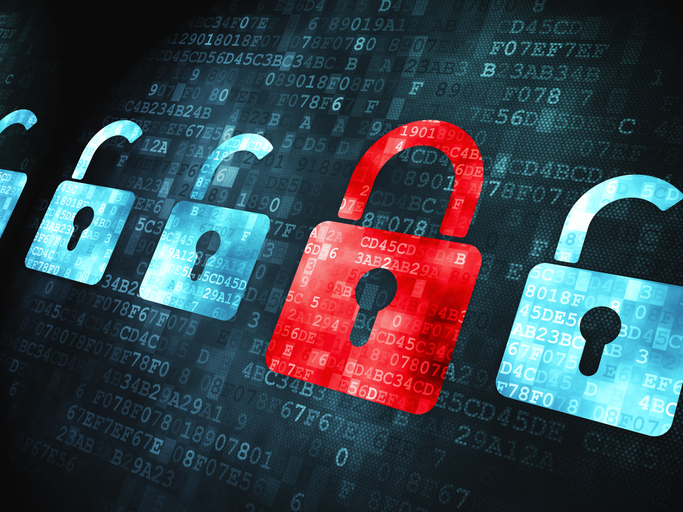
Toward Safer AI
Addressing the safety and data privacy concerns associated with generative AI requires a multi-faceted approach, involving developers, regulators, and users. Nevertheless, all solutions stem from just two main motivations: Limit the model’s access to private data and remove all unnecessary details. How is that even possible? The answer is synthetic data.