Science Research: Achieving Great Breakthroughs with Synthetic Data (7/29)
Table of Contents
1. Introduction
In the field of natural sciences, obtaining large volumes of high-quality experimental data is crucial. However, this can be a significant challenge in terms of time and cost. Moreover, the data collection process may involve ethical issues. To overcome these limitations, synthetic data can be utilized. Although synthetic data is not real, it is generated based on real data, maintaining statistical properties while excluding sensitive information or personal data. Additionally, synthetic data can be easily produced in large quantities from a small amount of existing real data. In this post, we will discuss whether synthetic data can truly be used in natural science research and how it can be effectively utilized.
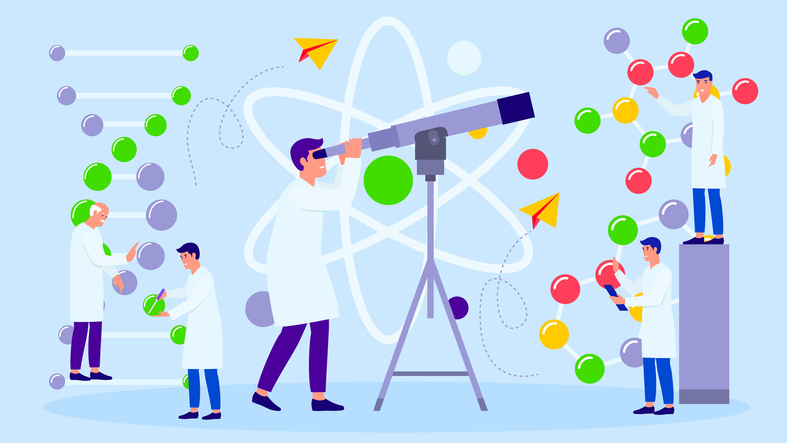
2. Assessing the Reliability of Synthetic Data for Natural Science Research
At this point, you might be wondering, “Isn’t data for natural science experiments supposed to be accurate? Is it okay to use fake data for experiments?” The answer to this question can be both yes and no. The accuracy of data is crucial in research, and the reliability of synthetic data depends on how it is generated. Therefore, we first introduce various methods to evaluate the reliability of synthetic data.
Since synthetic data is generated based on real data, it retains the statistical properties and patterns of the original data. Therefore, most synthetic data is reliable. However, many people might still have the bias that synthetic data is inferior to real data. Here are some methods to prove the reliability of synthetic data.
2.1. Comparison with Real Data Distribution
One way to assess the reliability of synthetic data is by comparing its distribution with that of real data. By ensuring that the statistical distributions of both data sets align closely, we can verify that synthetic data accurately reflects the properties of real data.
2.2. Classification Experiments
Another method involves conducting classification experiments. By training machine learning models on synthetic data and testing them on real data (or vice versa), we can evaluate how well the synthetic data represents the real-world scenarios.
2.3. Modeling and Validation
Synthetic data should be modeled and validated rigorously. The models used to generate synthetic data should be trained on real data, and the generated data should undergo extensive validation to ensure it aligns with the characteristics of the original data.
By using these methods, we can determine whether synthetic data is inferior, similar, or even superior in quality to real data. For instance, the DTS synthetic data generation solution from the service AZOO has undergone extensive validation across numerous data sets and is continually tested and improved.
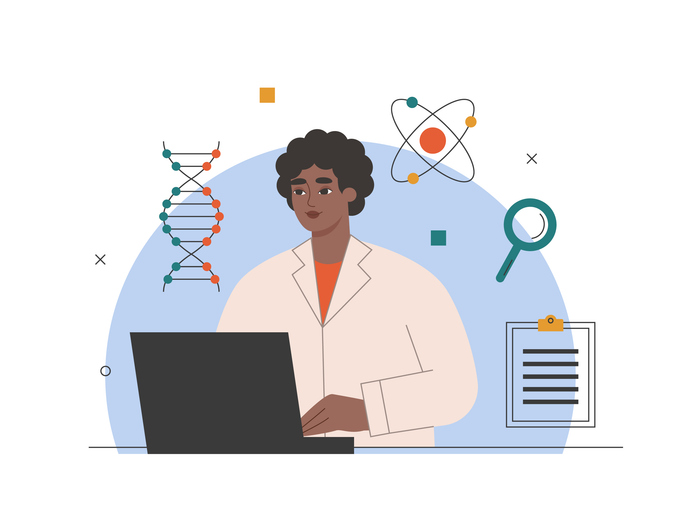
3. Case Studies of Synthetic Data in Real Research
3.1. Genetic Research (Science Research)
Synthetic genetic data can be used to test various hypotheses related to gene expression, contributing to the development of new gene therapies based on real experimental data.
3.2. Astrophysics
Synthetic data generated from astronomical observation data can be used to study various cosmic phenomena. This is particularly useful for simulating scenarios that are difficult to observe in reality.
3.3. Drug Development
Synthetic data for various compounds can help in screening potential drug candidates and simulating chemical reactions, thus enhancing the efficiency of drug development.
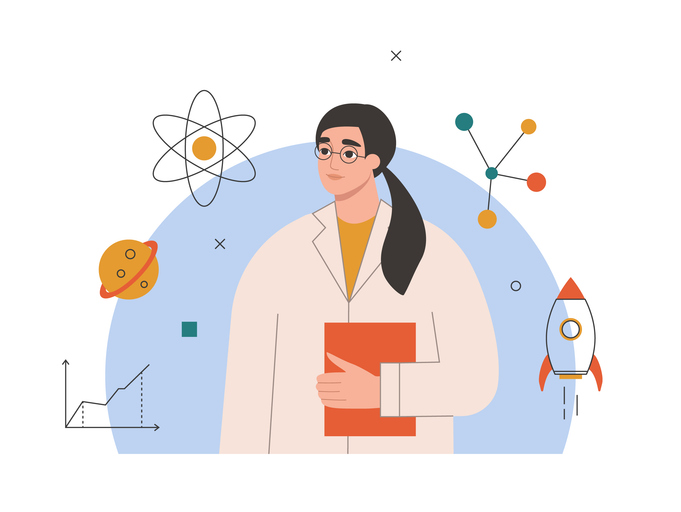
4. Conclusion
Synthetic data has become an essential tool in natural science research. It helps maintain the accuracy and reliability of data while reducing costs and time. It also offers a way to avoid ethical issues associated with real data collection. Moreover, synthetic data can be generated in any desired quantity, making it useful for reducing biases related to race, gender, etc. The use of synthetic data is expected to continue expanding, opening up new possibilities that go beyond the limitations of traditional natural science research.
If you are interested in synthetic data generation solutions like DTS provided by AZOO, visit their website.
For more information on synthetic data, AI, security, and other related topics, feel free to explore our blog.