How Prompt Tuning Elevates Generative Masterpieces! (4/11)
Table of Contents
Introduction
In the field of AI particularly in generative models, the technique known as “prompt tuning” has emerged as a key handle. This approach manipulates the prompts (short texts or instructions) fed into models, significantly influencing their output without altering the underlying model architecture. The importance of prompt tuning lies in its ability to fine-tune model responses, leading to enhanced performance and more accurate, contextually relevant outputs. It can divides into two main types: soft prompt tuning and discrete prompt tuning.

Soft Prompt Tuning
Soft prompt tuning, also known as “continuous prompt tuning”, involves embedding trainable parameters which don’t look like natural language. Unlike traditional methods that train the model’s weights, soft prompt tuning introduces a layer of abstraction. The model learns optimal embeddings for the prompts during the training process. These embeddings are not fixed but adapt based on the task making the model more flexible and capable of handling a wide array of scenarios. Soft prompt tuning is particularly beneficial for tasks where direct manipulation of model weights is impractical or where the goal is to maintain the original model’s integrity while still customizing the outputs.
https://huggingface.co/docs/peft/conceptual_guides/prompting
Discrete Prompt Tuning
On the other hand discrete prompt tuning focuses on modifying the prompt’s content or structure without altering the model’s learned parameters. This approach needs to make prompts to guide the model towards the desired output. By carefully selecting words, phrases, or even the format of the prompt, we can steer the model’s attention and improve its performance on specific tasks. Discrete prompt tuning is especially useful when working with black-box api model like GPT, where modifying the internal architecture or weights is not an option.
Impact of prompt-tuning on Generative Model Quality
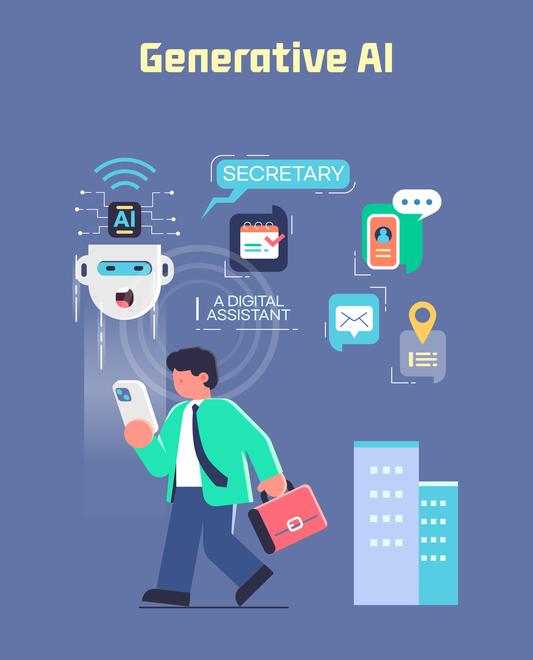
The quality of outputs from generative models can be dramatically improved through effective prompt tuning. By refining prompts, it’s possible to achieve outputs that are not only more accurate and contextually relevant but also more creative and diverse. We can write down below details.
1. Enhanced Contextual Relevance
Prompt tuning allows for more nuanced control over the generative process, enabling models to produce outputs that are closely aligned with the given context. This is especially important in tasks that require a deep understanding of context, such as generating text that fits a particular narrative or style.
2. Improved Accuracy and Performance
Through the careful design of prompts, models can be guided to focus on the most relevant aspects of a task thereby improving accuracy and overall performance. This fine-tuning process helps in minimizing errors and producing outputs that closely match human expectations.
3. Increased Creativity and Diversity
Finally, prompt tuning can expand creativity and diversity in model outputs. By experimenting with different prompt styles and structures, it’s possible to upgrade models to handle a wider range of possibilities, leading to varied outputs.
conclusion
In conclusion, prompt tuning is one of the most important thing to handle generative models. Whether through soft or discrete methods, the careful tuning of prompts can significantly enhance the quality of generative models.

CUBIG is crafting sensational synthetic data that seem magically geared with mind-blowing prompts. Interested? Click below!