For Large language model surpassing GPT-4(05/14)
Table of Contents
In the past two years, if you were to name the most talked-about generative model, many would point to ChatGPT. Initially, its outstanding performance captivated both users and researchers. However, the lack of detailed disclosures limited in-depth analysis.
The Rise of Open Source Large Language Models
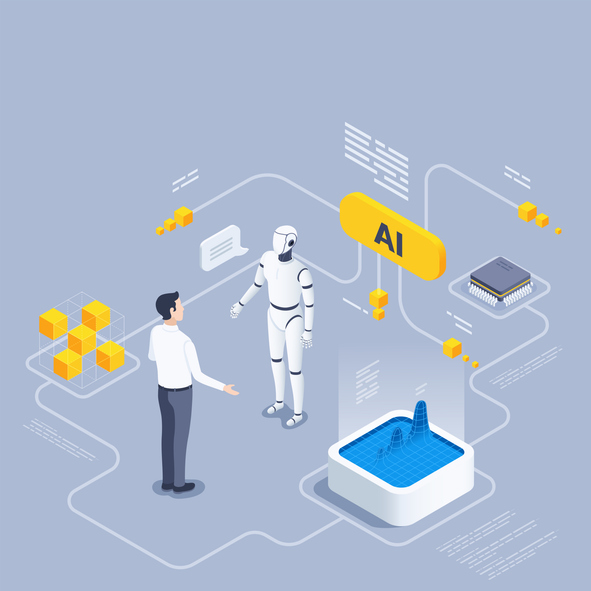
With the advent of open-source LLMs like LLaMA2, BLOOM, and Falcon, understanding and researching large language models have significantly advanced. Despite this progress, there remains a performance gap between these open-source models, which are primarily trained on publicly available data, and the proprietary commercial models in the market. Consequently, there is ongoing research aimed at bridging this performance gap using these foundational models.
Need More Varied Data
Several limitations have been identified in existing large language models. One major issue is the performance drop in languages other than English. This trend is also observed in commercial language models. The recently introduced open-source LLM, LLaMA3, underscores the importance of high-quality data in improving model performance. LLaMA3 invested substantial time in training with vast amounts of data, using over 15 trillion tokens collected from publicly available sources for its pre-training. This model also incorporates data in various languages, addressing a significant weakness of previous LLMs.
more details on LLaMA3: [Meta AI’s blog]
The quality and diversity of data are crucial to enhancing model performance. However, open-source models are often constrained by the limited availability of open-source data, which can lead to out-of-distribution issues when deployed in the market. There are several approaches to mitigate this, one of which includes using synthetic data generation services to obtain a wide variety of training data.
Link: CUBIG Azoo