AI Security: How to Trust AI Models Better (5/17)
As we become more reliant on AI, concerns about AI security increase, especially in today’s data-driven world, where AI models are substantially employed to make predictions that impact everything from individual credit scores to medical diagnoses. In response, two significant countermeasures have been gaining popularity: homomorphic encryption and differential privacy.
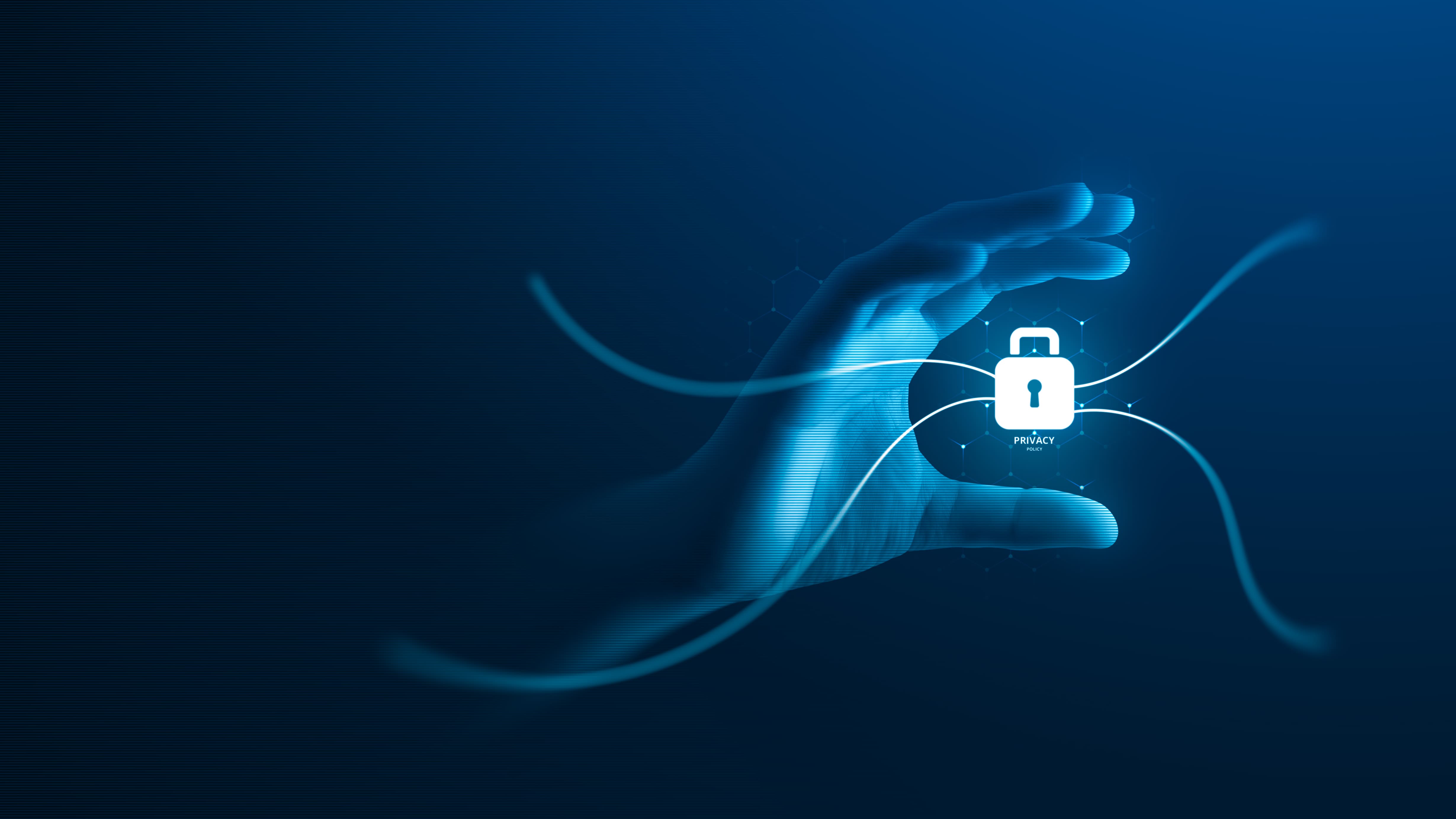
Homomorphic Encryption: Robust but Complex
The idea of homomorphic encryption is simple: process data in encrypted form. This is crucial to ensure that the confidentiality of data is not compromised while being processed. In other words, an AI model can perform operations on encrypted data without ever seeing the actual data. However, it may sound paradoxical to achieve meaningful results when the meaning of inputs are unknown, which makes homomorphic encryption complicated to implement and computationally expensive.
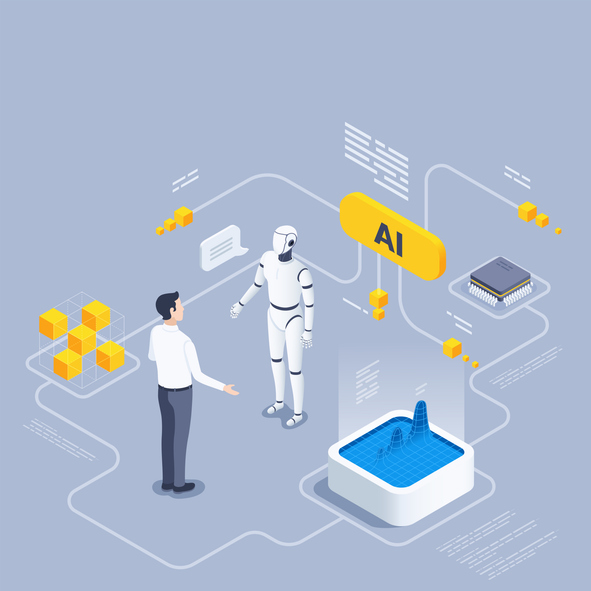
Differential Privacy: Balancing Utility and Privacy
On the other hand, differential privacy offers a more accessible approach to safeguarding data. This technique adds controlled noise to the dataset or to the AI’s outputs so that adversaries cannot analyze the output and extract sensitive information out of it. In short, differential privacy obscures the details of individual data points.
For example, if you observe that a medical record of a man increased the success probability of a certain disease’s treatment, then you can easily infer that the man had that disease, underwent treatment, and survived. Differential privacy prevent this kind of inference, ensuring that one cannot determine whether any individual data was included in the dataset based on the output. In this way, organizations can share findings from their data without exposing sensitive information.
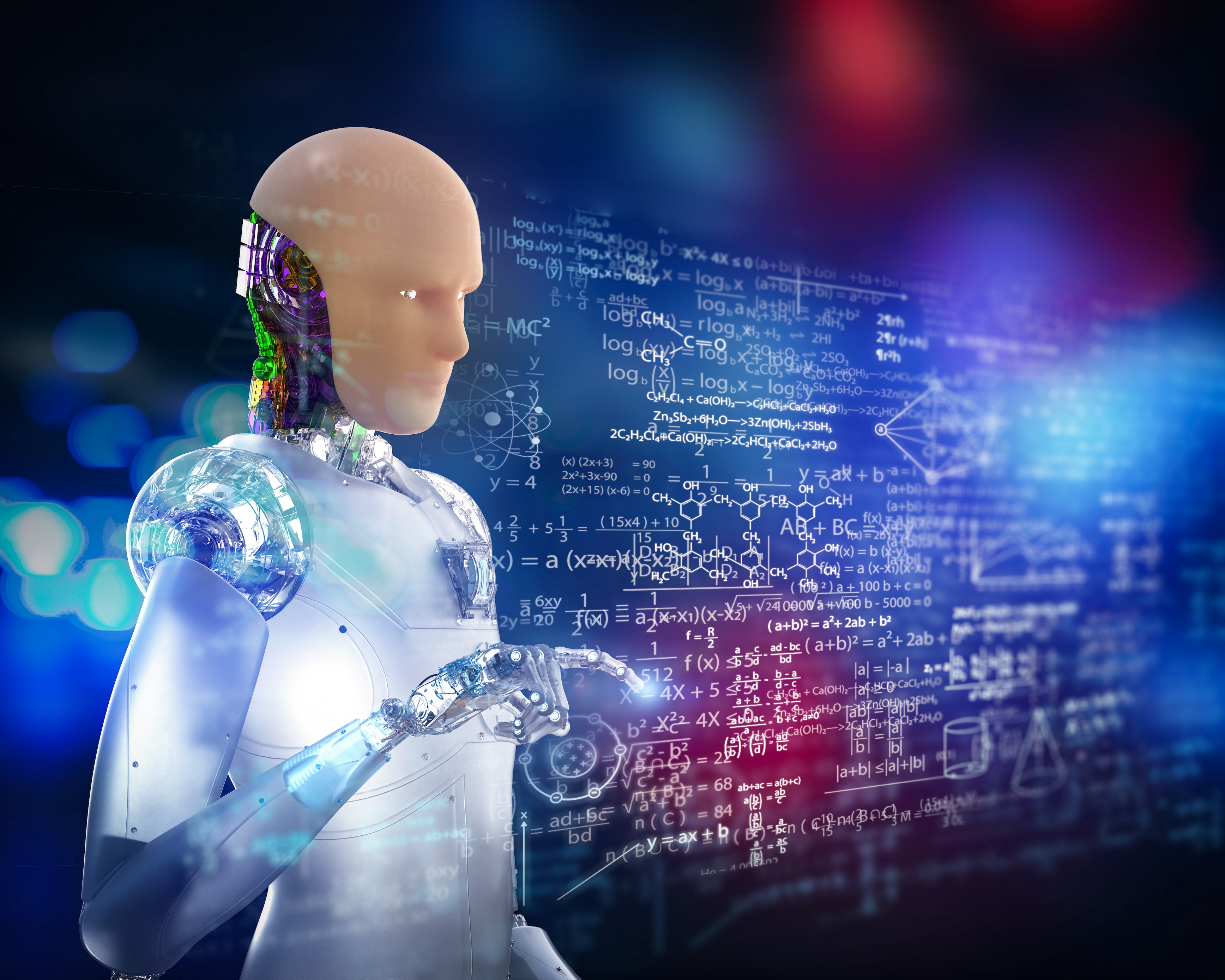
Practical Consideration for AI Security
In summary, while both homomorphic encryption and differential privacy offer effective ways to protect data privacy in AI applications, differential privacy often provides a more balanced solution. This subtle yet significant advantages often makes differential privacy the preferred choice for organizations aiming to build trust and ensure the reliability of their AI security.
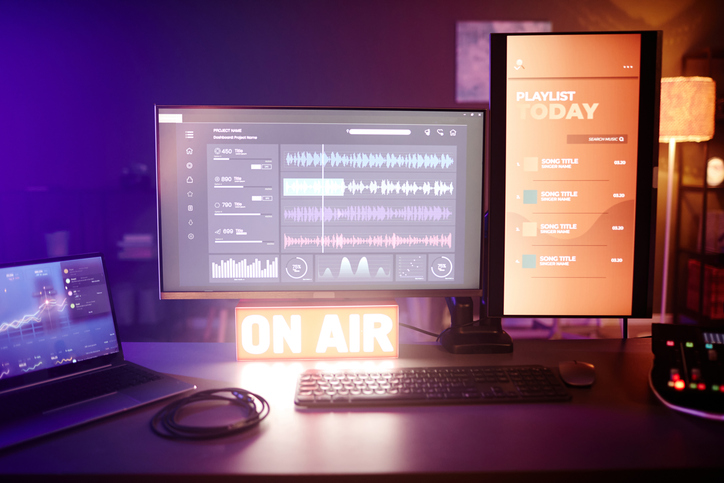