3 Best Datasets for Computer Vision
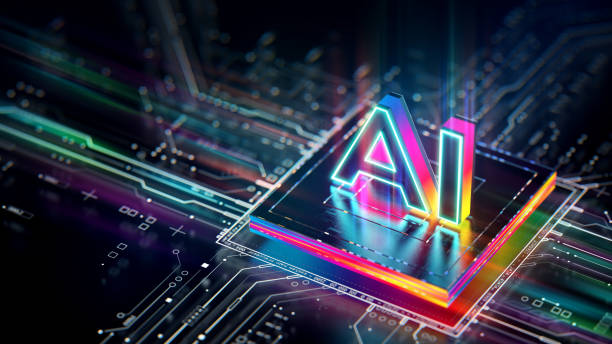
Even if you’re not experienced in AI, you may still understand that data is crucial. Datasets for AI not only provide a consistent framework for comparison but also push the boundaries of what AI can achieve by presenting challenging problems to solve. In this article, we delve into five benchmark datasets that are critical for researchers and practitioners looking to stay at the forefront of AI developments.
ImageNet
ImageNet is one of the most influential datasets in the field of computer vision. It consists of over 14 million images categorized into more than 20,000 classes. The dataset has been instrumental in the development and benchmarking of image recognition algorithms. The annual ImageNet Large Scale Visual Recognition Challenge (ILSVRC) has spurred significant advancements in deep learning and convolutional neural networks (CNNs), making it a cornerstone for evaluating the performance of AI models in image classification and object detection tasks.
CIFAR
The CIFAR dataset is a collection of images used for machine learning and computer vision research. The most commonly used versions are CIFAR-10 and CIFAR-100, which contain 60,000 32×32 color images each, divided into 10 and 100 classes, respectively. CIFAR-10’s dataset is split into 50,000 training images and 10,000 testing images, with each class (such as cars, birds, cats, etc.) represented equally in the dataset. CIFAR-100 follows a similar structure but with 100 classes grouped into 20 superclasses, providing a more fine-grained classification challenge. These datasets are widely used for training and testing algorithms in image recognition tasks.
CoCo
The COCO dataset is another cornerstone in the field of computer vision, focusing on image recognition, segmentation, and captioning. It features over 330,000 images with 80 object categories, providing more than 200,000 labeled instances. This dataset is unique because of its emphasis on object detection in the context of scene understanding, making it more challenging and closer to real-world applications than datasets that focus solely on object classification.
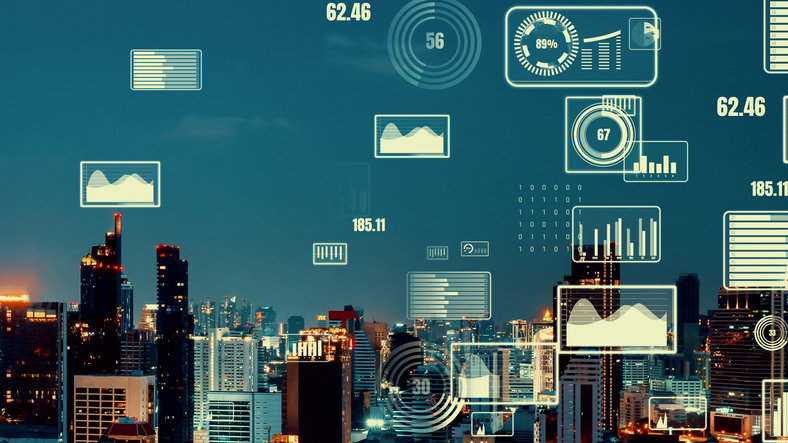
Can benchmark datasets accurately represent the real world?
The answer is simple. No, they don’t. Benchmark datasets are typically created by researchers with great care, in contrast to the unpredictable nature of real-world data, which can feel as random as rolling a dice. They tend to be harder than the carefully crafted benchmark dataset. As a result, you may see undesirable performance out of an AI model if you train it with benchmark datasets only.
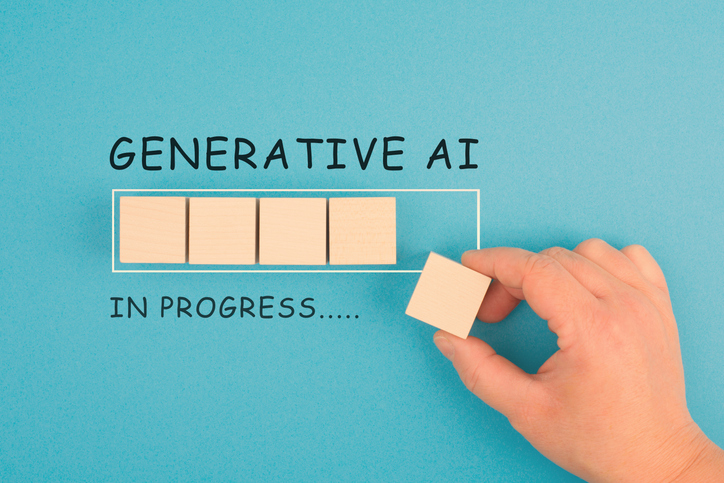
Synthetic Data: the Breakthrough
You may ask then, what should I use to train my model if the benchmarks are not enough? There are datasets that are intentionally made to resemble the real world and therefore random-looking on purpose. The thing is, they are only the minority. What we need to do is increase their number and size. How so? Generative AI is the key.
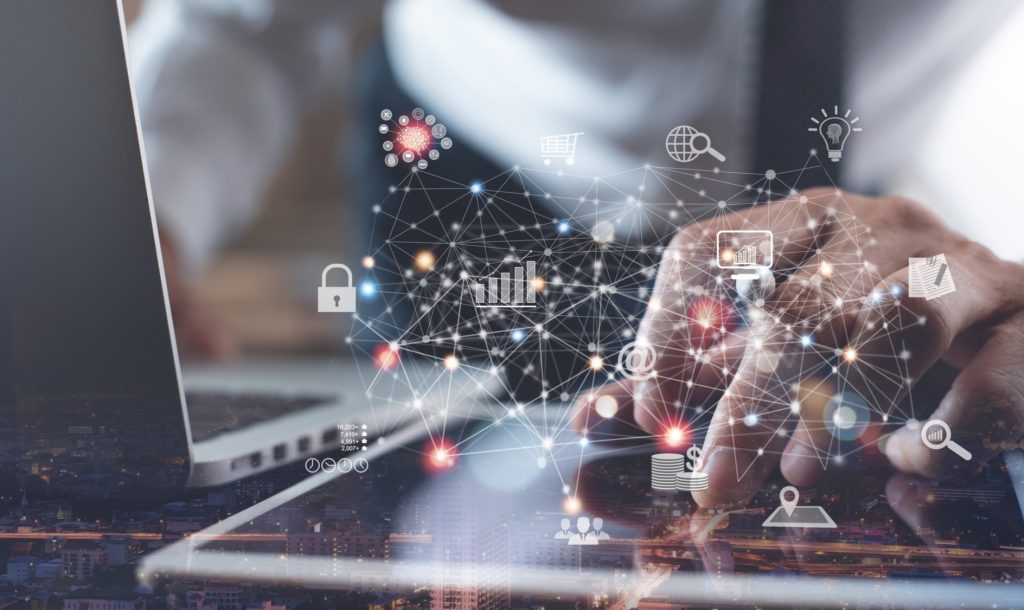
Synthetic Yet Real
The old saying goes, ‘Nothing beats the real thing.’ Well, it is not the case for data. Synthetic data have so many benefits over the real ones.