Federated Learning Explained: 3 Powerful Advantages of Decentralized AI
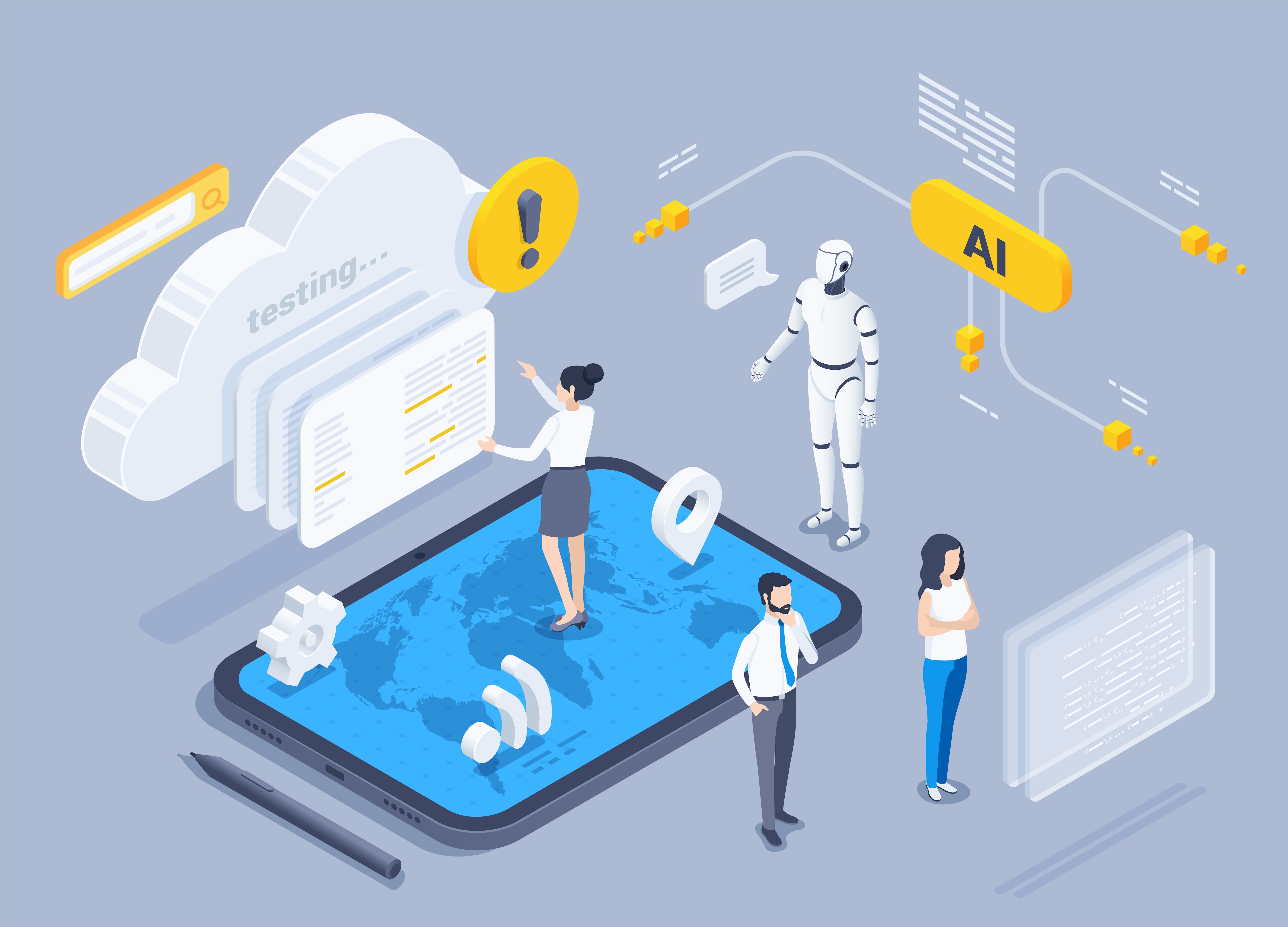
Federated learning (FL) is swiftly becoming a pivotal technology in AI. This cutting-edge method enables the training of machine learning models across various decentralized devices or servers without the need to share the local data samples. By keeping data local, FL significantly mitigates privacy risks and optimizes data efficiency across different networks.
Federated Learning for a Better Data Privacy
The foremost advantage of FL is its ability to uphold stringent data privacy standards. Traditional machine learning approaches often require data owners to send their data to a centralized server, posing significant risks of data breaches or misuse. Federated learning, however, allows data to remain on local devices, such as smartphones or hospital servers, and only the minimal necessary informationโmodel updates, not the data itselfโis shared to a central server.
This structure significantly mitigates the risk of exposing sensitive information. It is particularly beneficial in sectors like healthcare and finance, where protecting personal data is paramount. By leveraging federated learning, organizations can utilize collective insights from vast datasets while complying with privacy regulations such as the GDPR in Europe and HIPAA in the United States.
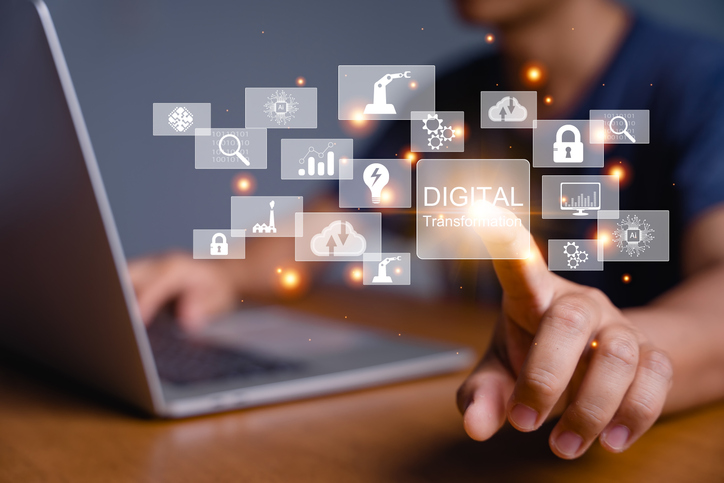
Federated Learning for Better Utilization of Diverse Data Sources
FL excels in environments where data is not only voluminous but also highly varied and distributed across many devices. This would be a challenge to conventional AI models as centralizing those data requires tremendous amount of time and resources. On the other hand, FL allows each participating device in the network to train a local model on its own dataset; these local models are then aggregated into a global model. This method ensures a rich, diverse set of data inputs that contribute to more robust and generalized AI models.
For instance, a smartphone app using FL can improve its predictive text capabilities without ever needing to access specific user data. Similarly, it allows for the development of personalized medical treatments by learning from patient data across numerous hospitals, without ever sharing the individual patients’ data. This ability to tap into a wide array of real-world data can significantly accelerate innovation and improve model accuracy.
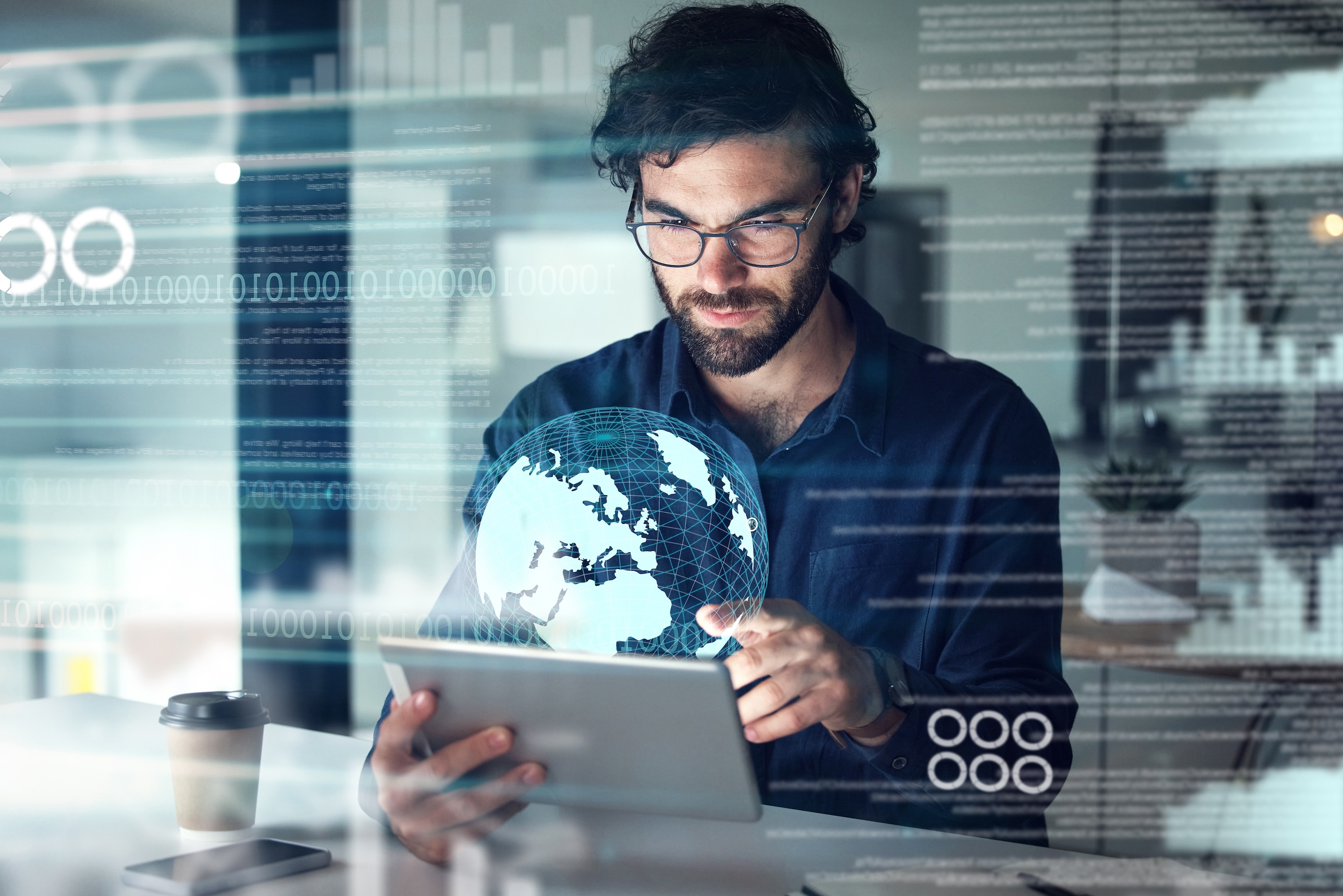
Federated Learning to Learn More and Quicker
By decentralizing the data analysis and processing to local devices, FL reduces the need for data transmission to a central server, thereby decreasing latency. This is crucial for applications requiring real-time processing and decision-making, such as autonomous driving systems or real-time health monitoring.
Furthermore, FL is inherently scalable. Since the central server needs to handle only model updates rather than the entire datasets, it reduces the computational and storage demands on the central system. This scalability makes federated learning an attractive option for growing networks of IoT devices and mobile applications, which are expected to explode in numbers in the coming years.
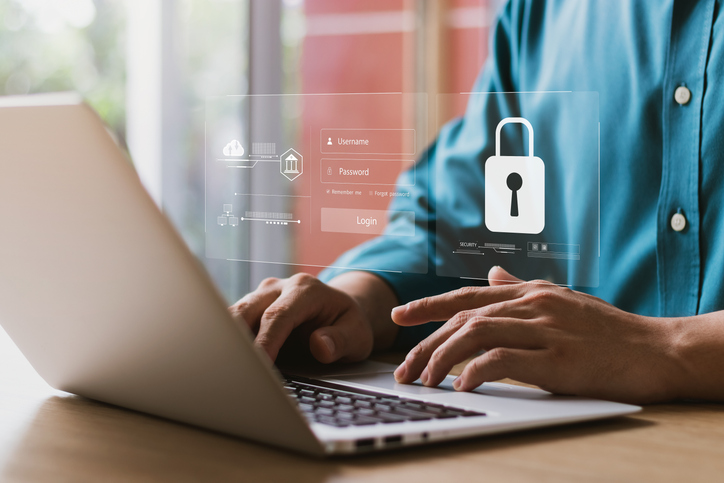
Remaining Privacy Risks in Federated Learning
While FL significantly enhances data privacy, it is not entirely without risks. One of the residual challenges is the potential for what is known as inference attacks. In these scenarios, malicious actors may attempt to reconstruct private data by analyzing the shared model updates. Although the raw data does not leave the local device, insightful details about the data can still be inferred from the patterns within the updates, especially if the attacker has access to auxiliary information.
To mitigate these risks, additional privacy-preserving techniques such as differential privacy, which adds controlled noise to the model updates, are often employed. Secure multi-party computation and homomorphic encryption are other strategies that can be used to further secure the data during the aggregation process. These technologies ensure that even if an attacker could intercept the model updates, the information gleaned would be insufficient to compromise individual data points effectively.