Pushing the Boundaries with RAG: New Horizons Data Diversity in Synthetic Data (07/10)
Table of Contents
Synthetic data has gained importance in recent years in the fields of machine learning and artificial intelligence. It addresses issues such as the scarcity of real data and concerns about privacy, contributing to enhancing model performance and generalization capabilities. One of the innovative approaches to synthetic data generation is Retrieval Augmented Generation technology, which combines retrieval-based information retrieval with generative models to increase both the diversity and quality of data.
The Encounter of RAG and Synthetic Data
One of the key advantages of RAG is its integration of information retrieval and text generation models, enabling an enhancement in both the diversity and quality of data simultaneously. When applied to the process of synthetic data generation, this technology allows for the creation of data that reflects various real-world contexts through search-based data extraction. This approach provides richer and more realistic datasets compared to traditional prompt-based methods, thereby synergistically improving model performance, especially in scenarios where data scarcity is an issue.
Furthermore, by leveraging filtered important documents during the information retrieval process, the generated data exhibits similarity to real data and helps reduce model biases. This proves advantageous, particularly in situations aiming for performance improvements in domains with limited data availability.
related paper: link
Integration of RAG and Synthetic Data: Applications Across Fields
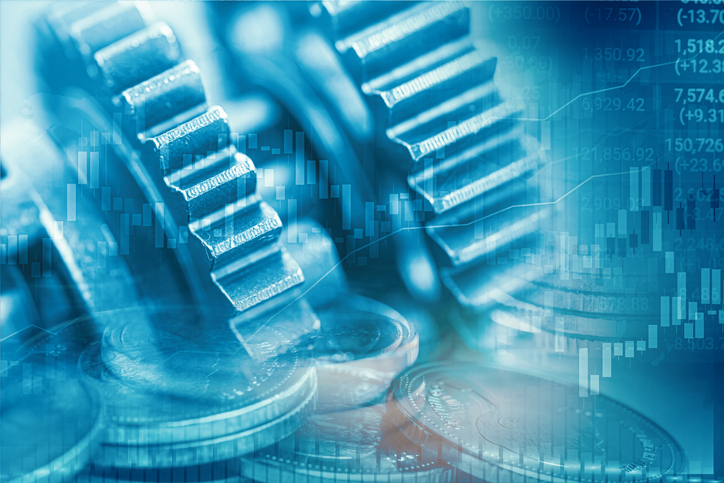
- Financial Data: In the financial sector, diverse and accurate data is essential for market trend analysis, risk management, and investment strategies. Using RAG, simulated financial transaction data can be generated, enabling training of AI models to support investment decision-making. Particularly in finance, where timeliness is crucial, combining RAG allows for the creation of synthetic data that closely resembles current trends.
- Medical Data: Medical data can innovate various critical aspects of medical research and clinical practice. Firstly, through RAG, it is possible to generate synthetic medical images that closely mimic real-world scenarios. By integrating information retrieval techniques, diverse datasets encompassing various modalities such as MRI, CT scans, X-rays, and multiple medical conditions can be created, enhancing the overall quality and representation of the data. Additionally, real-time generation of synthetic medical images allows reflection of current medical trends and advancements.
related post: link