AI Dataset Repository: The Backbone of Innovation in Artificial Intelligence (8/23)

In the ever evolving world of artificial intelligence (AI), data is hailed as the new oil, fueling AI development and innovation. The AI dataset repository lies at the heart of this data-driven revolution. This blog explores why AI dataset repositories are so crucial, how they contribute to the progress of AI technologies, and the impact they have on the future of this transformative field.
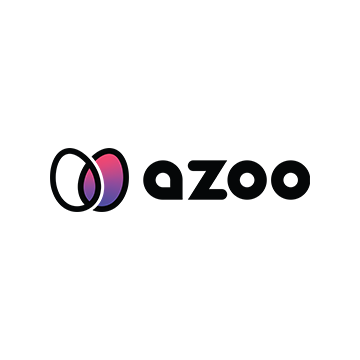
The Role of a Good AI Dataset Repository
AI dataset repositories are centralized platforms where datasets are stored, shared, and managed. There repositories are essential for several reasons.
Centralized Access
They provide a single point of access for researchers, developers, and organizations to obtain diverse datasets. This centralization makes it easier to find and use the data necessary for training and utilizing AI models.
Diversity and Quality
Repositories often include datasets from various sources and domains, ensuring a broad range of data types and quality. This diversity is crucial for developing trustworthy AI models that are generalizable and robust.
Standardization
Many repositories enforce standards for data formats and metadata, which facilitates easier data integration and comparison. This standardization helps streamline the process of preparing data for AI training and evaluation.

How AI Dataset Repositories Drive Innovation
Speeding Up Research and Development
Repositories provide easy access to high-quality datasets, allowing researchers and developers to swiftly test and improve their AI models. This accelerates the pace of innovation and reduces the time required to bring new AI technologies to market.
Facilitating Collaboration
AI dataset repositories often include community features that allow researchers to share datasets, work together on projects, and exchange insights. This collaborative environment fosters innovation and helps overcome challenges by leveraging collective expertise.
Supporting Benchmarking and Evaluation
Repositories provide standardized datasets that are used to benchmark and evaluate AI models. This benchmarking is crucial for comparing different approaches, measuring progress, and setting performance standards in the AI community.

Challenges and Considerations
While a good AI dataset repository is invaluable, there are challenges for repositories to be good:
Data Privacy and Ethics
Ensuring that datasets are collected and shared ethically is a major concern. Repositories must implement policies and practices to protect user privacy and avoid misuse of sensitive data.
Bias and Fairness
Datasets can inadvertently contain biases that may lead to biased AI models, causing unintended impacts. Repositories need to include mechanisms for identifying and mitigating bias to ensure fairness and inclusivity in AI applications.
Data Quality and Maintenance
Maintaining the quality and relevance of datasets is the top challenge of AI datasets. Repositories must regularly update and curate their data to ensure that it remains accurate and useful for current AI research.
Case Studies
Kaggle Datasets
Kaggle is a famous AI dataset repository that hosts a wide range of datasets across different domains. The accessibility of these datasets has facilitated numerous machine learning projects and competitions, driving innovation and providing valuable learning opportunities for data scientists.
However, since datasets on Kaggle are contributed by users, the quality and consistency can vary significantly. Not all datasets are well-documented or curated, which can harm their reliability and usability.
Furthermore, Kaggle’s public datasets are shared openly, which raises concerns about the privacy and ethical use of data. Users need to be cautious about handling sensitive information and ensuring that datasets comply with legal and ethical standards.
AZOO
AZOO is a specialized AI dataset repository where synthetic data can be bought and sold. It offers custom AI data that can be used in various fields, enabling researchers and organizations to easily acquire the data they need, while data providers can sell the data they generate. AZOO plays a key role in both buying and selling synthetic data.
One of the biggest strengths of AZOO is that it provides high-quality synthetic datasets that satisfy differential privacy. This helps users to comply with legal and ethical issues regarding data privacy while obtaining high data analysis results.