Anomaly Detection Revolutionized: 5 Breakthrough Benefits of Synthetic Data
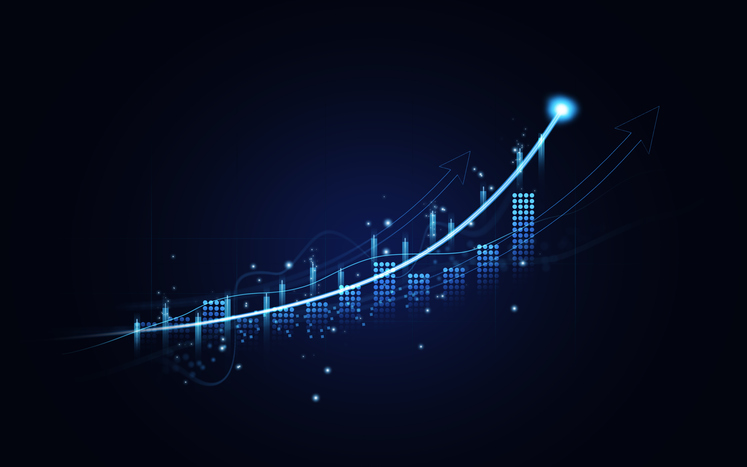
Anomaly detection is essential across industries such as finance and healthcare, where identifying unusual patterns can signal fraud, errors, or other significant issues. Traditional methods for training anomaly detection models often struggle due to the limited availability of high-quality, labeled data. Synthetic data offers an innovative solution, transforming the landscape of anomaly detection.
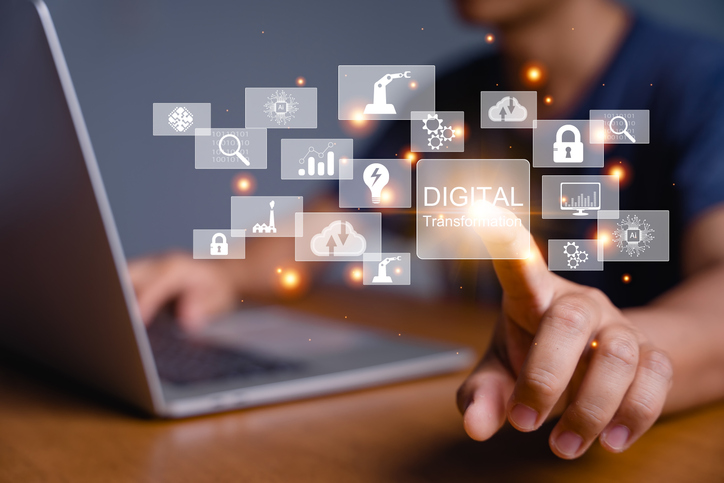
Understanding Anomaly Detection
Anomaly detection is the process of identifying unusual patterns or behaviors within a dataset that do not conform to expected norms. These anomalies can indicate critical issues such as fraud, errors, equipment failures, or other irregularities. Anomaly detection is widely used in monitoring, to enhance security, improve operational efficiency, and ensure quality control. The process involves analyzing data to uncover deviations from standard patterns, which can then be further investigated to determine their cause and significance.
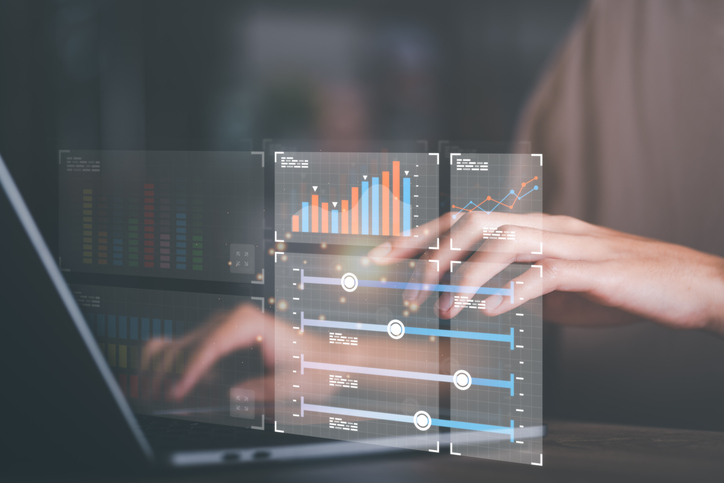
Benefits of Synthetic Data: 1. Abundance of Training Data
One of the most significant advantages of synthetic data is its capacity to generate large volumes of training data. Real-world datasets, particularly those with anomalies, are often limited and imbalanced. Synthetic data can be customized to create a diverse array of scenarios, ensuring machine learning models encounter a wide variety of anomalies. This comprehensive exposure improves the models’ performance in real-world applications, where detecting rare but critical anomalies is essential.
Benefits of Synthetic Data: 2. Enhanced Privacy and Security
Synthetic data significantly enhances privacy and security by eliminating the risks associated with handling sensitive information. Real-world datasets often contain personally identifiable information or other sensitive data that are challenging to anonymize effectively without diminishing their utility. Synthetic data, however, is generated to mimic the statistical properties of real data while containing no actual sensitive information. This crucial characteristic allows organizations to train and test their anomaly detection models comprehensively without compromising data privacy. Additionally, synthetic data can be shared across teams and organizations more freely, fostering collaboration and innovation without legal or ethical concerns.
The use of synthetic data ensures robust model development while maintaining stringent privacy standards, ultimately reducing the risk of data breaches and privacy violations. This approach supports the broader adoption of advanced analytics and machine learning practices in industries where data security and privacy are paramount concerns. By enabling comprehensive testing and development without compromising privacy, synthetic data promotes a more secure and collaborative environment for data-driven innovation.
Benefits of Synthetic Data: 3. Improved Model Generalization
Models trained on synthetic data can generalize better to unseen data. Because synthetic data can be designed to encompass a wide range of possible anomalies, models learn to detect patterns beyond the limited scope of real-world training sets. This capability is crucial in anomaly detection, where anomalies can be highly unpredictable and varied. Improved generalization ensures that the models are more robust and reliable in detecting novel anomalies in operational environments.
Moreover, synthetic data allows for the simulation of rare and extreme scenarios that may not be present in real-world datasets. This aspect is particularly valuable in anomaly detection, where the ability to identify and respond to rare events is critical. By exposing models to these uncommon but significant scenarios, synthetic data helps build resilience and adaptability into the models, preparing them for a broader range of potential issues. Additionally, the use of synthetic data can speed up the training process, as large volumes of high-quality data can be generated quickly and cost-effectively. This leads to more efficient development cycles and ultimately, more effective anomaly detection solutions in real-world applications.
Benefits of Synthetic Data: 4. Cost-Effective Data Generation
Collecting and labeling real-world data for anomaly detection is both time-consuming and expensive. Synthetic data generation offers a cost-effective alternative, minimizing the need for extensive data collection efforts. Advanced techniques like generative adversarial networks (GANs) and variational autoencoders (VAEs) can create high-fidelity synthetic datasets with minimal human intervention. This efficiency enables organizations to allocate resources more effectively while still developing high-performing anomaly detection models.
Benefits of Synthetic Data: 5. Accelerated Development and Testing
The availability of synthetic data accelerates the development and testing cycles of anomaly detection models. With an abundant supply of diverse and representative data, data scientists and engineers can rapidly iterate on model design and parameter tuning. Additionally, synthetic data allows for rigorous testing under controlled conditions, helping to identify and address potential model weaknesses before deployment. This accelerated development process fosters faster innovation and enhances model performance in production.