Excellent Chatbots and Customer Support with LLMs and RAG (06/21)
Table of Contents
The Role of Large Language Models (LLMs) in Interactive AI
Interactive AI systems have rapidly evolved in recent years, thanks to advancements in artificial intelligence (AI) technology. These systems primarily use large language models (LLMs) and retrieval-augmented generation (RAG) techniques to provide more sophisticated and useful conversations. In this article, we’ll explain the roles of LLMs and RAG in interactive AI and explore real-world applications like chatbots and customer support systems.
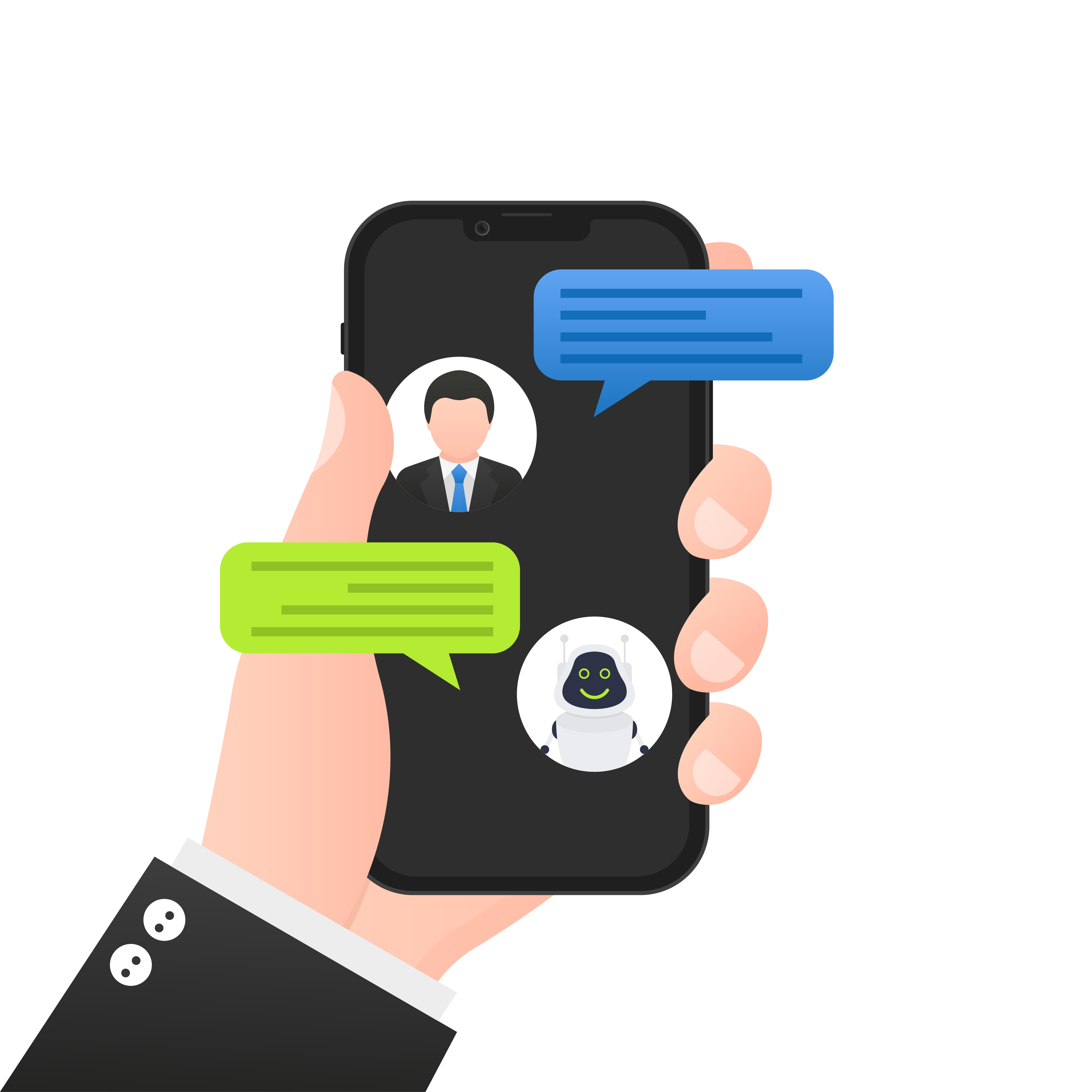
Understanding Retrieval-Augmented Generation (RAG)
Large language models (LLMs) are trained on vast amounts of text data to perform natural language understanding (NLU) and natural language generation (NLG). Examples of LLMs include OpenAI’s GPT series and Google’s BERT model. LLMs play a crucial role in interactive AI systems due to their ability to understand context and generate natural responses. They excel at understanding user questions and providing appropriate answers. However, LLMs alone may have limitations in offering up-to-date information or deep knowledge in specific domains.
To overcome these limitations, retrieval-augmented generation (RAG) techniques are used. RAG combines LLMs with traditional information retrieval systems, allowing LLMs to provide more accurate and current information. A RAG system analyzes the user’s question, retrieves relevant information from databases or the web, and helps the LLM generate a more precise response. This significantly enhances the accuracy and reliability of LLM responses.
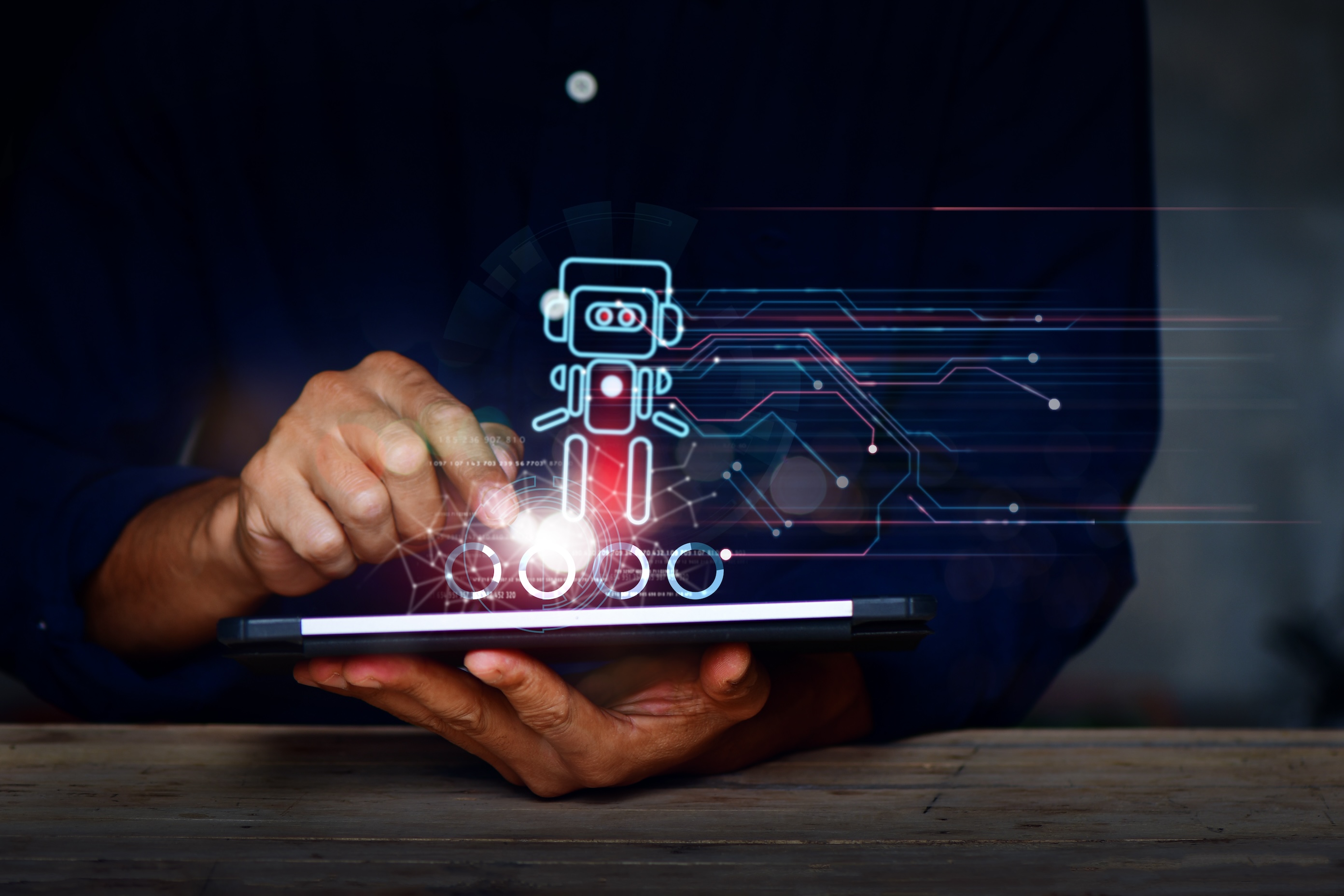
Practical Applications: Chatbots
In practice, interactive AI systems using LLMs and RAG are applied in various fields. One prominent example is chatbots. Chatbots are used for customer support, information provision, booking services, and more. By using LLMs and RAG, chatbots can offer more natural and accurate conversations. For instance, when a customer asks about a specific product, the chatbot understands the question using the LLM and retrieves the latest product information through RAG to provide an accurate response.
Another application is in customer support systems. Traditional customer support systems mainly provided pre-defined answers, but systems integrating LLMs and RAG can respond to a wide range of customer inquiries in real-time. For example, if a customer asks about a specific problem, the system quickly retrieves relevant information and uses the LLM to suggest an appropriate solution. This greatly improves the efficiency and satisfaction of customer support.
Conclusion: The Future of Interactive AI with LLMs and RAG
In conclusion, the combination of LLMs and RAG significantly enhances the performance of interactive AI systems and demonstrates its usefulness in various real-world applications. As these technologies continue to advance and expand in scope, we can expect to see more AI solutions that revolutionize user experiences.
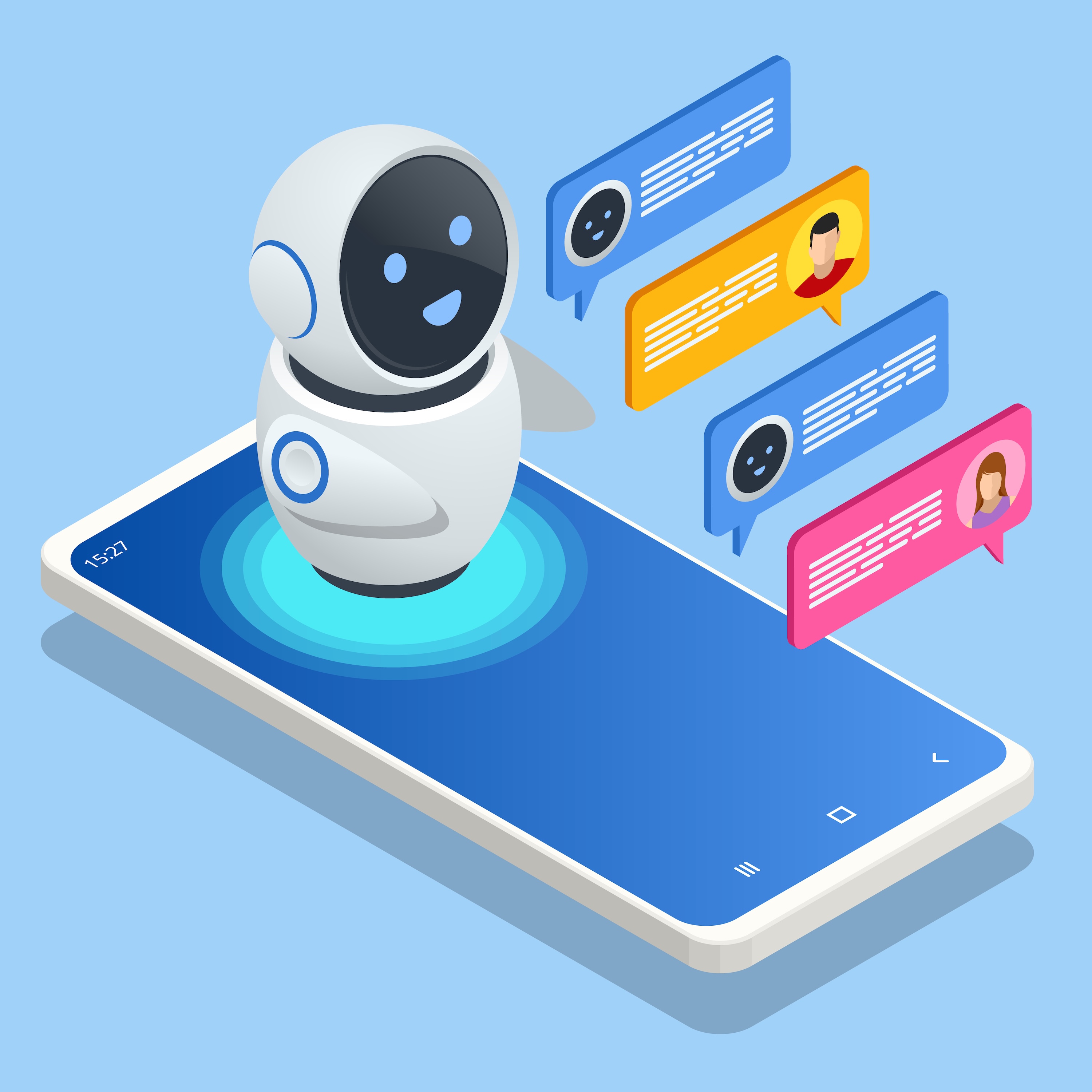
If you want to read more, click the links!: