Why Density and Coverage Outperform Precision and Recall in Evaluating Synthetic Data Quality
Table of Contents
In the world of synthetic data, evaluating quality is crucial for ensuring data that accurately represents real-world distributions while offering the necessary variety. Traditionally, metrics like precision and recall have been used to assess aspects of fidelity and coverage in generated data. However, as synthetic data generation evolves, these metrics have shown limitations. In contrast, density and coverage are emerging as more reliable indicators for evaluating synthetic data, especially when it comes to measuring the true quality of generated samples.
The Limitations of Precision and Recall
Precision and recall have long been fundamental in assessing classification tasks, but when applied to synthetic data evaluation, these metrics can lead to misleading conclusions. Precision is meant to assess how well generated samples fall within the dense regions of real data, while recall gauges how well real data samples are represented within the generated distribution. However, these metrics struggle with outliers and distribution boundaries, often resulting in inflated scores that don’t necessarily indicate quality. For instance:
- Precision can be artificially boosted if synthetic samples merely cover outliers or sparse regions in the real data, giving the impression of high fidelity without truly capturing the core of the data distribution.
- Recall may increase if generated samples are dispersed broadly, capturing a wide range of the real data but failing to reflect the meaningful density and variation within the primary data.
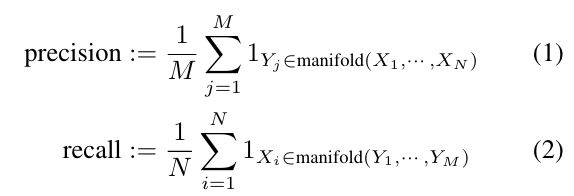
The Power of Density and Coverage
To address these limitations, density and coverage provide a more nuanced evaluation. Density measures how well synthetic samples capture the concentrated regions of real data, effectively assessing the fidelity without succumbing to outlier issues. Coverage, on the other hand, evaluates the range and breadth of synthetic samples, ensuring they represent the underlying variability within the real dataset.
- Density assesses the extent to which synthetic samples are embedded within dense areas of real data, providing a clearer picture of how well the synthetic data captures the essential characteristics of the real dataset.
- Coverage ensures that generated data spans the entire spectrum of the real dataset’s distribution, which is critical for maintaining the utility and representativeness of synthetic data.


Why Density and Coverage are Essential for Synthetic Data Quality
As synthetic data continues to play an integral role in various industries—from healthcare and finance to machine learning research—the need for reliable quality metrics will only grow. Density and coverage offer a way to objectively gauge whether synthetic data can stand in for real data without falling into the pitfalls associated with precision and recall. These metrics not only provide a better understanding of fidelity and coverage but also help in improving synthetic data generation processes by guiding developers toward more representative and useful datasets.
As synthetic data applications expand and technology advances, metrics like density and coverage will be at the forefront, providing the insights needed to ensure high-quality, reliable synthetic data.
At Azoo (https://azoo.ai), we understand that reliable synthetic data isn’t just about generation—it’s about quality. That’s why we provide not only high-quality synthetic datasets but also robust evaluation metrics that ensure data fidelity, density, and diversity, giving you full confidence in your purchase. If you’re looking to enhance your models with synthetic data that meets real-world standards, visit our platform to explore a range of synthetic datasets crafted to meet your specific needs. Join us at Azoo and discover synthetic data solutions with the transparency and quality metrics you can trust.
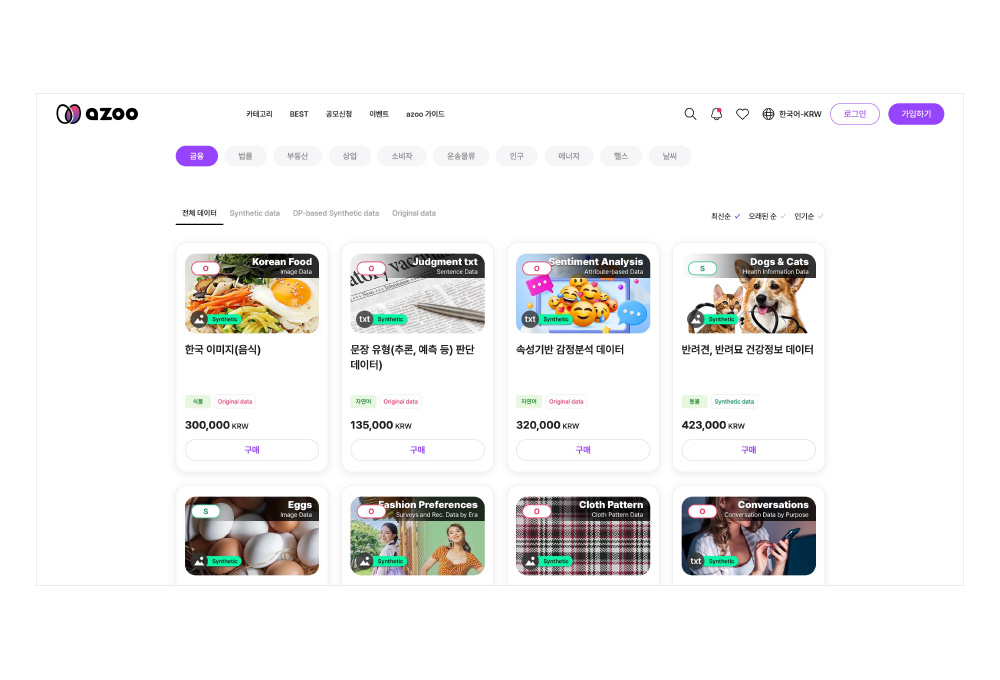
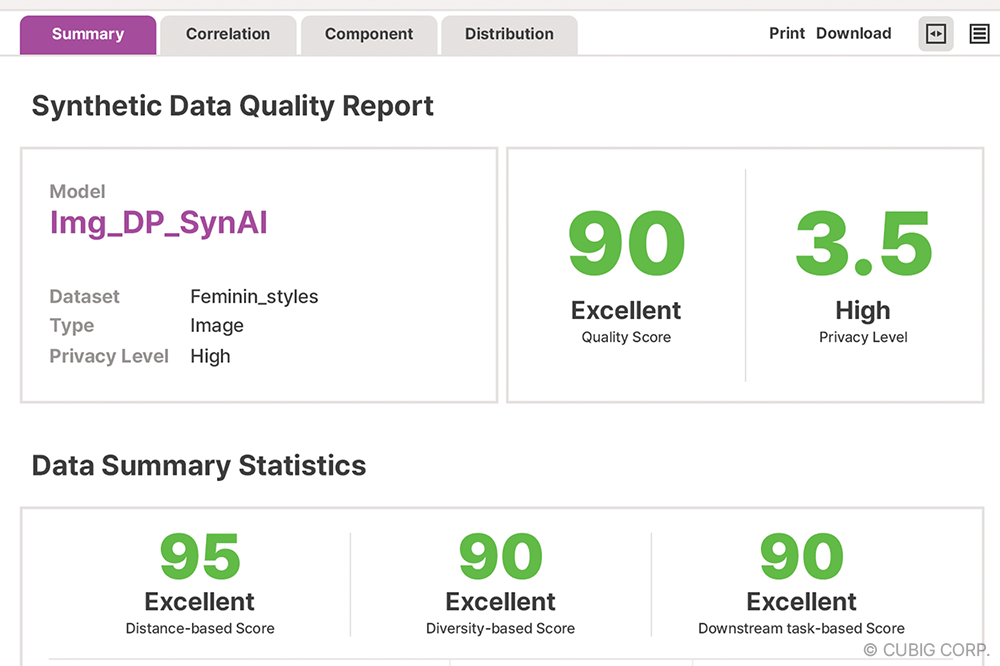