Contrastive Learning Unleashed: Transforming AI with Revolutionary Techniques (6/4)

In the dynamic landscape of AI, contrastive learning stands out as a revolutionary technique poised to redefine the capabilities of AI models in understanding and interacting with complex data sets. Unlike conventional neural network architectures, this self-supervised learning strategy offers a profound transformation in the AI sector, setting a new precedent for technological advancement in 2024 and beyond.
Exploring the Fundamentals of Contrastive Learning
Contrastive learning is a sophisticated machine learning approach that enhances a system’s ability to discern and assimilate the nuances of data by identifying and comparing differences and similarities among data instances. It is rooted in self-supervised learning paradigms where the AI model autonomously generates labels from the data it processes. This method enables AI to develop a nuanced understanding of the attributes that either differentiate or link various data points. By learning from the inherent diversity in data, it facilitates a more natural and efficient acquisition of knowledge, significantly improving learning efficacy and data utilization.

The Significance of Contrastive Learning in Modern AI
The introduction of contrastive learning marks a major paradigm shift in AI methodologies, primarily due to its enhanced efficiency and adaptability. A critical advantage of this approach is its capacity to utilize unlabeled data, which is generally more abundant and less costly than labeled datasets. By reducing reliance on human-annotated labels, this learning paradigm democratizes AI development, enabling quicker, more economical, and universally accessible advancements across multiple sectors.
Another significant attribute of contrastive learning is its ability to efficiently prepare models for a variety of downstream tasks with minimal additional training. This efficiency stems from the generalizable features that the models cultivate, which are adaptable enough for deployment in various contexts and challenges. As models engage in the learning, they acquire an in-depth comprehension of the intrinsic qualities of data, thus equipping them to rapidly and effectively adapt to novel and unforeseen tasks.
These capabilities have far-reaching implications. They foster the development of stronger AI applications in situations where obtaining labeled data is cumbersome or unfeasible, such as in remote sensing or behavioral studies conducted in natural settings. Consequently, contrastive learning is instrumental in developing more sophisticated, efficient, and adaptable AI systems, which is essential for the future scalability of AI technologies. Therefore, contrastive learning represents not just an enhancement in AI capabilities but a fundamental shift towards a more sustainable, expansive, and inclusive approach to AI applications.

Synthetic Data: A Strategic Component in Contrastive Learning
In the realm of contrastive learning, the skillful creation of positive and negative data pairs is crucial. Synthetic data proves invaluable in this context, offering a controlled environment to generate these crucial pairs. This is particularly beneficial when real-world examples of negative pairs are rare or challenging to gather. By precisely replicating these pairs, synthetic data ensures a balanced and diverse dataset, which enhances the model’s generalization abilities during its training phase.
Moreover, synthetic data allows for the customization of features within these pairs, facilitating the fine-tuning of the contrastive learning process to target specific traits or behaviors. This is especially advantageous in sectors like robotics and medical imaging, where actual data may be limited or difficult to obtain in sufficient variety. By simulating complex or rare interactions, synthetic data aids in training models to recognize subtle distinctions or resemblances, significantly boosting their accuracy and operational effectiveness.
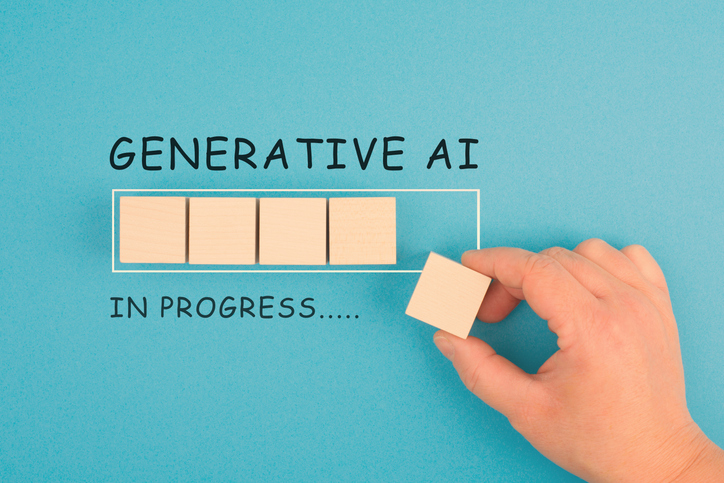
The strategic use of synthetic data not only augments the variety and intricacy of the training pairs but also substantially enhances the overall efficacy of contrastive learning. As a result, models develop a deeper comprehension of crucial data characteristics, which translates into superior performance across a broad spectrum of real-world applications, paving the way for groundbreaking advancements in AI.