GraphRAG: Surpassing RAG’s Limitations and Breaking New Ground (06/16)
Table of Contents
In recent years, advances in artificial intelligence have brought revolutionary changes to the fields of information retrieval and generation. In particular, the Retrieval-Augmented Generation (RAG) model has garnered attention for effectively combining generative and retrieval-based approaches to provide accurate and diverse answers to user queries. Businesses, in particular, are actively pushing for rapid adoption of this technology.
RAG’s Limitations
RAG integrates generative and retrieval models to enhance content generation by having the generative model incorporate additional information retrieved through the retrieval model. However, traditional RAG may underutilize or overly rely on user query information, leading to issues in balancing the relevance of the query. Moreover, it sometimes fails to retrieve documents closely related to user queries due to challenges in indexing and determining the optimal number of documents to retrieve. Addressing these issues requires multiple experiments to find the optimal parameters.
About RAG: link
The Utility and Applicability of GraphRAG
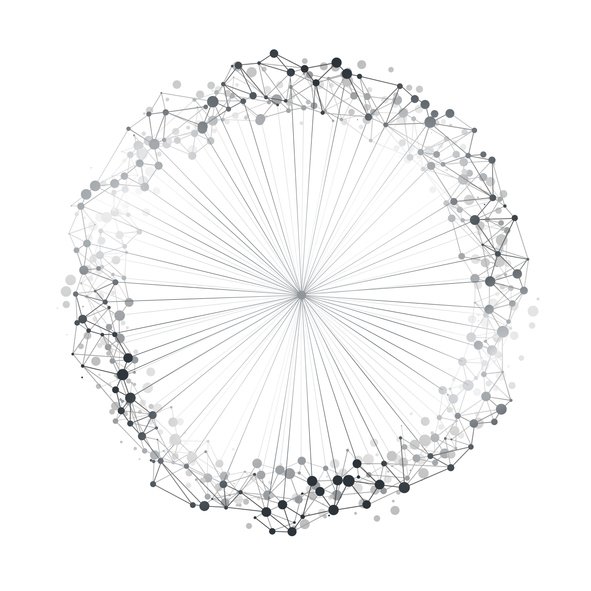
This is an advanced technology that operates as a graph-based version of the RAG model, utilizing knowledge graphs to perform information retrieval and generation. While traditional RAG adjusts a natural language generation model based on retrieved documents to generate answers to queries, GraphRAG further utilizes knowledge graphs to understand context and extract relevant information during this process.
Using GraphRAG offers several advantages:
- Semantic Relationship Analysis: GraphRAG analyzes the semantic relationships between documents and information based on knowledge graphs. This enables deeper information extraction and relationship analysis beyond simple text-based searches.
- Accurate Information Retrieval: Compared to traditional RAG, GraphRAG retrieves more accurate and relevant information. It selects optimal documents and information related to user queries by leveraging the structure and relationships within the knowledge graph.
- Enhanced Contextual Understanding: GraphRAG can understand and apply context more comprehensively. By considering various connections and relationships within the knowledge graph, it provides deeper meanings beyond simple textual information.
- Flexible Information Augmentation: During the information augmentation (generation) process, GraphRAG generates more flexible and accurate answers using diverse relationships and connections. This helps better reflect the information users need.
- Subjective Problem Solving: When retrieving information related to user queries, GraphRAG resolves problems from a broader perspective compared to simple text-based approaches. This enhances user experience and delivers more satisfying results.
Related posts: link