How DTS Accelerates AI Adoption in Finance
Issues(AI Adoption)
Financial institutions increasingly adopt artificial intelligence (AI) to enhance their operations and offer better services. Key applications include:

- Fraud Detection: AI-powered systems analyze transactional patterns in real time to identify and prevent fraudulent activities.
- Credit Risk Analysis: Machine learning models assess creditworthiness more accurately, reducing defaults and improving loan allocation.
- Personalized Services: AI tailors product recommendations, creating a more engaging customer experience.
However, the sensitive nature of financial data brings unique challenges:
- Data Access and Privacy Concerns: Institutions must comply with stringent data privacy regulations such as GDPR (General Data Protection Regulation) and CCPA (California Consumer Privacy Act).
- Regulatory Compliance: Cross-border data sharing and third-party collaborations become complex, limiting the usability of valuable datasets.
Example
AI adoption in finance often relies on vast amounts of high-quality data for training machine learning models. However, financial institutions face significant obstacles:
- Regulatory Barriers: Sharing real-world financial data for AI model training can violate privacy laws, especially when dealing with personally identifiable information (PII).
Despite its potential, synthetic data presents challenges:
- Quality Concerns: Ensuring that the generated data is realistic and statistically similar to the original data is critical for effective AI model training.
- Security Risks: Trust in synthetic data diminishes if there’s even a minimal risk of re-identifying sensitive information from the generated datasets.
Data Transform System (DTS) for Finance
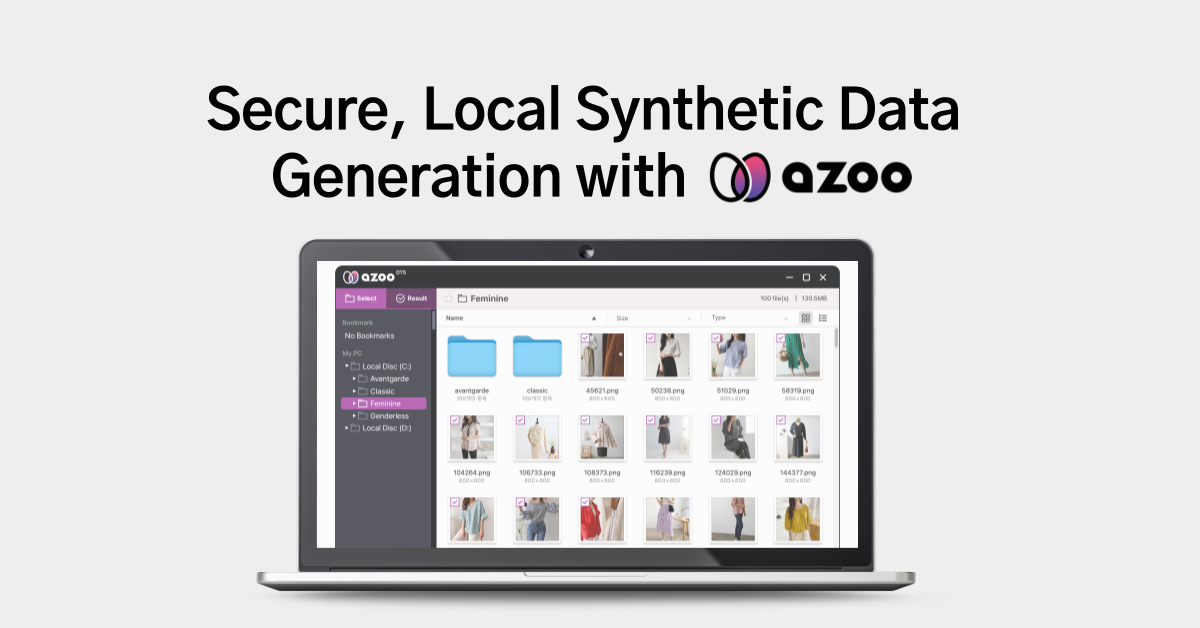
DTS fundamentally addresses the data utilization challenges faced by the financial industry, delivering a variety of positive outcomes.
- Regulatory Compliance: DTS transforms sensitive original data into secure synthetic data, ensuring compliance with data protection regulations such as GDPR and CCPA. This eliminates legal risks associated with data usage and facilitates data sharing and collaboration in global environments.
- Data Quality Assurance: DTS generates synthetic data that is up to 99% similar to the original, ensuring the reliability and accuracy needed for training machine learning models. This overcomes the limitations of traditional synthetic data, which often fail to meet quality standards.
- Enhanced Data Security: By incorporating differential privacy techniques, DTS eliminates the possibility of re-identifying original data from synthetic datasets. This alleviates concerns about customer information leakage, enabling financial institutions to use data securely while maintaining customer trust.
DTS serves as a powerful tool for financial institutions to pursue innovation while balancing customer trust and regulatory compliance. By leveraging DTS, the financial industry can unlock new possibilities in data utilization, maintain competitiveness, and promote sustainable growth.