How to Safely Use Medical Data Thatโs Locked Away Due to Privacy Concerns
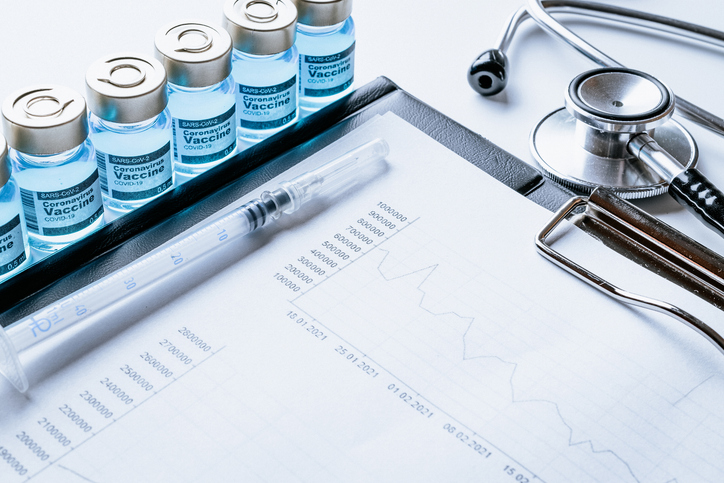
Table of Contents
Introduction
Medical data is often locked behind strict privacy regulations, preventing it from being used to its full potential in research and healthcare innovation. However, thereโs a way to generate data that is remarkably similar to your original dataset while keeping sensitive information private. This is made possible through advanced techniques that do not compromise privacy but still provide highly useful datasets.
Why This Approach Works
The process for generating synthetic data achieves high similarity without risking privacy because:
- State-of-the-Art Generative AI Doesnโt See Your Original Data: The AI model is trained on vast, diverse datasets and creates synthetic data based on patterns it has learned. It doesnโt directly access your original data. Instead, the process involves refining the generated samples by selecting only those that closely align with the statistical properties of your data.
- DP(Differential Privacy) Techniques Protect Individual Information:ย DP ensures that even as the generated data is refined, no individual details are compromised. This is done by adding noise at critical points, making sure privacy remains intact.
- DP is Applied During the Comparison Phase, Not to the Model Itself:ย The original dataset never needs to leave the secure environment. Instead, differential privacy is used when comparing synthetic data to the original, keeping the data evaluation localized and secure.

How the DTS(Data Transformation System) Fits In
The DTS incorporates these methods as part of a broader strategy to enhance data generation capabilities across various domains, including images, tables, and text. It plays a crucial role in:
- Integrating ongoing advancements in generative AI and privacy-preserving techniques to improve the quality of synthetic data.
- Facilitating versatile data handling, enabling the generation of realistic datasets suited for different applications.
- Ensuring adaptability to evolving privacy requirements, providing a continuous solution for data-sensitive fields like healthcare.
Why This Matters for Healthcare
With these techniques integrated into the DTS, healthcare organizations can:
- Develop reliable AI modelsย for detecting diseases such as heart conditions or diabetes using data that include important label features but doesn’t include private information
- Enable safe data sharing and collaboration with datasets that reflect real-world patterns without exposing personal information.
- Simulate clinical trials or patient outcomesย with synthetic data that aligns with actual population trends, supporting predictive studies without involving real patient data.
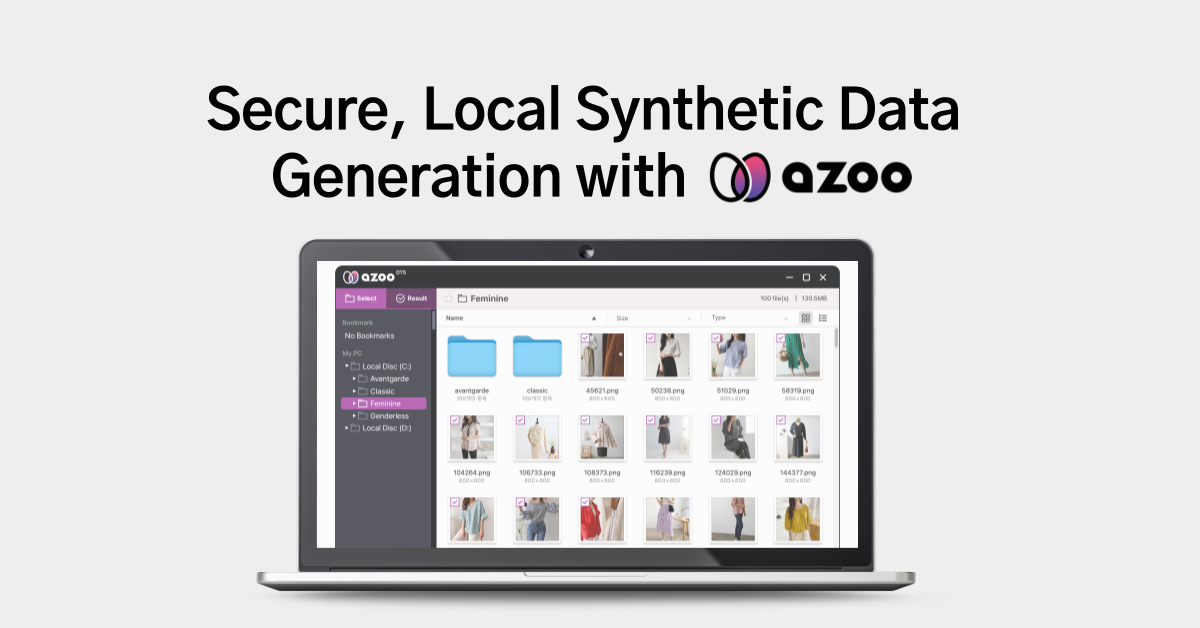
Conclusion
The combination of advanced generative AI and differential privacy, implemented as part of a larger system, ensures that synthetic data remains both secure and highly valuable. The Cubig’s DTS, with its role in integrating these techniques, supports ongoing efforts to make high-quality data accessible for research and AI development across various fields.