How Private LLMs are Transforming Enterprise Security and Efficiency (07/26)
Table of Contents
What is a Private LLM?
A Private Large Language Model is an AI language model trained using only an enterpriseโs internal data. These models are developed to address specific business requirements and data security concerns. Private LLMs enable companies to leverage their language models without sharing sensitive information or data with external parties.
Differences from Public LLM
Public Large Language Model is spearheaded by big tech companies like OpenAI and Google. These models are trained on large-scale data collected from the internet or publicly available sources. While Public LLM is widely accessible to various users, they pose risks of data breaches and hallucination issues, where incorrect information is generated. In contrast, Private LLM use only internal data, enhancing security and tailoring learning to the companyโs specific needs, thus minimizing data leakage and hallucination risks.
The Necessity of Private LLM
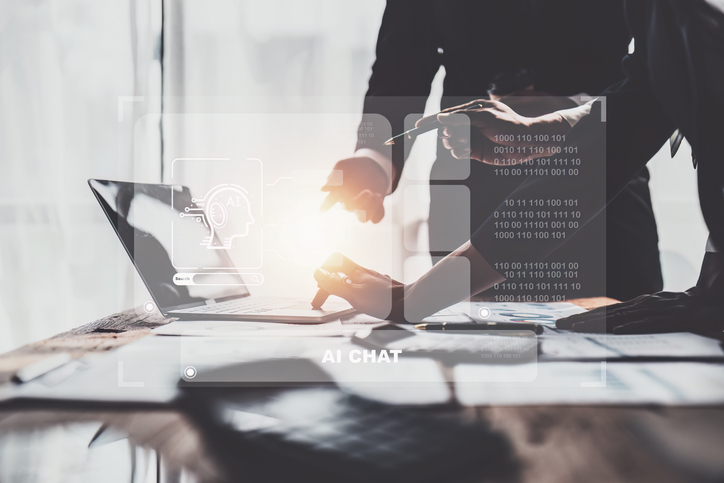
- Enhanced Data Security
- Private LLM is trained using only internal data, significantly reducing the risk of data breaches. This ensures that sensitive or critical information is protected, greatly enhancing the companyโs data security.
- Business Optimization through Customized Learning
- Private LLM is tailored to the specific requirements and characteristics of an enterprise, providing results optimized for the business environment. This allows companies to operate more efficiently and offer personalized services to customers.
- Cost Efficiency
- Private LLM typically have smaller model sizes compared to Public LLMs, resulting in lower operating costs. Smaller models maximize cost-effectiveness and deliver efficient performance tailored to the company’s specific needs.
Market Trends and Scale of Private LLMs
Global Market Trends
The Private LLM market is gaining attention from both global big tech firms and emerging startups. In response to the public LLM market led by companies like OpenAI and Google, Private LLMs are rapidly growing by meeting demands for security and customized learning. Salesforce’s “Einstein GPT” and Upstage’s performance highlight this trend. Notably, Upstageโs 70 billion parameter model outperformed GPT-3.5 on the global machine learning platform Hugging Face.
Domestic Market Trends
In Korea, interest in Private LLMs is also rising. Companies like Samsung SDS, Saltlux, and Upstage are adopting Private LLMs, leveraging them to enhance customer service personalization and business efficiency. CUBIG is aligned with this trend, researching the improvement of Private LLM performance using synthetic data generation technology to enrich learning data and enhance model accuracy and performance.
Future Outlook
Private LLMs are expected to play an increasingly vital role as the need for data security and customized learning grows. Companies can minimize data leakage risks and enhance competitiveness through models optimized for their business environment. Additionally, advancements in hardware, combined with on-device AI, will enable more sophisticated personalization strategies.
Private LLMs will become key solutions impacting business strategies significantly. As technology advances, Private LLMs will find widespread application across various industries, marking a new turning point in AI technology. CUBIG will continue to improve the security and performance of Private LLMs through differential privacy technology and synthetic data generation, supporting enterprises in utilizing AI technology more safely and efficiently.
Related posts: link