Need a Secret Weapon for LLM Innovation? Synthetic Text Data Unlocks the Door to LLM Revolution (4/7)
Table of Contents
Introduction
A Large Language Model (LLM) refers to an AI model capable of understanding and generating human language. Since the emergence of ChatGPT-3.5 at the end of 2022, LLMs have continued to undergo remarkable advancements. Now, LLMs have diversified in their purposes and utilities, reaching a stage where they can be practically applied in almost every field including healthcare, law, finance, research, customer service, advertising, and marketing.
However, the training of such LLMs requires vast amounts of data. Furthermore, this training data not only needs to be abundant but also of high quality and diversity. Yet, obtaining high-quality data solely from real sources can be challenging in terms of curation, and there may be limitations in the quantity of data that can be obtained legally.
For these reasons, there is a need to create synthetic text data to train LLMs. In this post, we will discuss how synthetic data could become another key driver revolutionizing the advancement of LLMs.
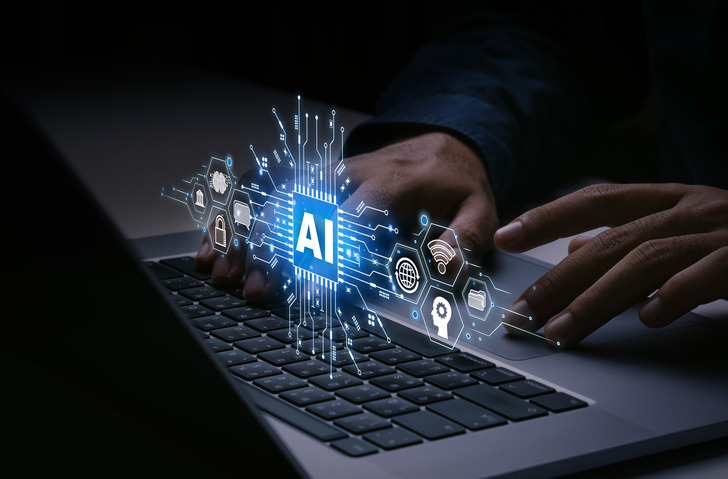
The Secret Weapon to Advance LLMs: Synthetic Text Data
1. Enhancement of Quantity and Diversity of Text Data
The performance of LLMs heavily relies on the quantity and diversity of the data used for training. To model the complex linguistic situations of the real world, a significant amount of data reflecting diverse contexts, topics, and language styles is required.
Synthetic text data artificially generates diversity, allowing models to learn a broader range of linguistic phenomena. Moreover, employing methods to create synthetic text data theoretically enables the generation of an infinite amount of text data, thus facilitating the acquisition of massive datasets. At first glance, you might wonder, “Is it necessary to create diverse text data using synthetic methods?” However, if the diversity of training data is lacking, the resulting LLM may exhibit biases. This not only hinders the model’s ability to generate diverse and creative language but also poses risks of generating language that may raise legal or ethical concerns. Therefore, it is imperative to produce diverse synthetic text data to mitigate such issues and effectively train LLMs.
2. Protection of Privacy through Synthetic Text Data
The process of collecting text data generated by real individuals may raise ethical concerns such as privacy infringements. Synthetic data offers a solution to these issues. Therefore, creating synthetic text data can provide high-quality data necessary for LLM training in a manner that protects personal information.
3. Reduction in Training Costs and Time
The process of collecting, curating, and preparing high-quality real data for LLM training demands significant time and resources. In contrast, synthetic data can be generated in large quantities at relatively lower costs and time, accelerating the training process of language models. In this regard, for developers or researchers involved in LLM development or research, considering the use of synthetic text data is prudent in terms of cost and time efficiency.

Conclusion
Synthetic data is poised to become an essential component in advancing LLMs. By utilizing synthetic text data to enhance the diversity of data for LLM training, while also addressing legal and ethical concerns and reducing training costs and time, we can anticipate significant progress in the development of LLMs.
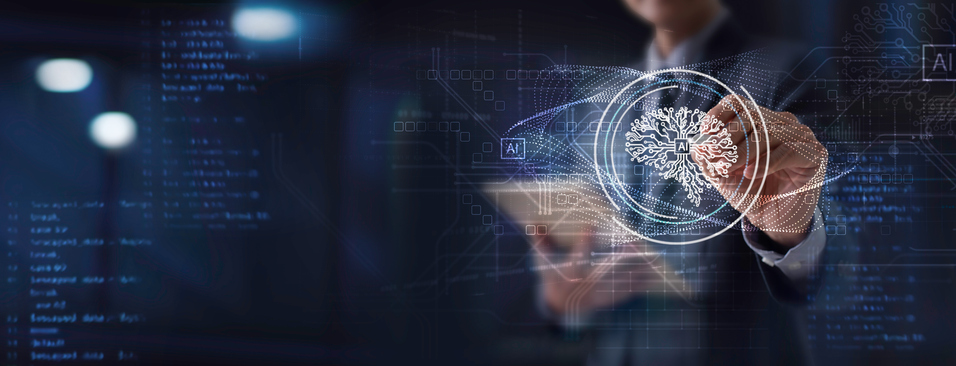
Synthetic data indeed holds great potential beyond fields like LLM. Are you curious about its applications in other diverse domains? Furthermore, are you interested in how synthetic data might impact various industries such as healthcare, finance, autonomous driving, and more in the future? If so, I invite you to explore our blog via the link below for a wealth of articles that delve deeper into these topics! I believe it could provide you with even more valuable insights.