Can You Really Train an AI Model Effectively Without Preprocessing? (03/11)
Table of Contents
In the realms of data science and machine learning, model training is typically conducted based on original data. However, using raw data as-is often requires extensive preprocessing and statistical knowledge, leading to potential loss of valuable information when the data format is mismatched. To address these challenges, utilizing synthetic data proves to be an extremely useful alternative.
Challenges of Original Data Preprocessing
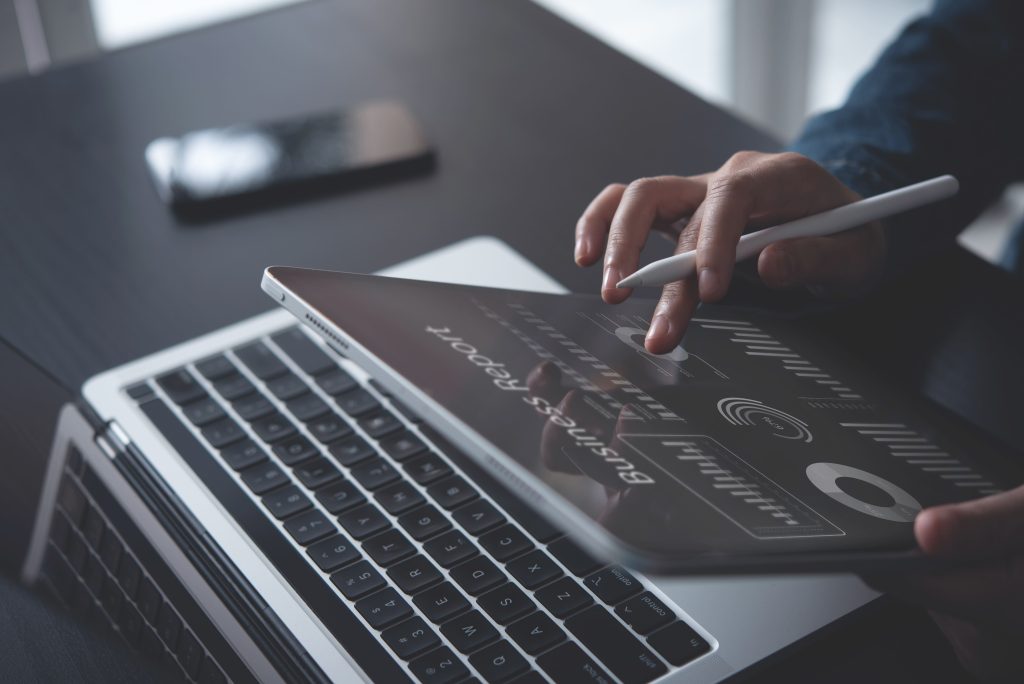
When using original data, one must navigate through various issues such as diverse data formats, missing values, and outliers. Dealing with these complexities demands a significant investment of time and effort in the preprocessing stage to ensure the data is suitable for model training. Actually, more time is spent on collecting and preprocessing data than on developing and improving the performance of AI models.
more about: link
In the manufacturing industry, for instance, when machines measure and record numerical values, there is a possibility of data loss due to malfunctions or stoppages. In such cases, some or all of the data for that time period may not be usable, and data scientists may resort to using post-processed data, augmented with their domain expertise, to fill in the gaps. However, this process can be cumbersome and costly.
In the manufacturing industry, for instance, when machines measure and record numerical values, there is a possibility of data loss due to malfunctions or stoppages. In such cases, some or all of the data for that time period may not be usable, and data scientists may resort to using post-processed data, augmented with their domain expertise, to fill in the gaps. However, this process can be cumbersome and costly.
Advantages of Synthetic Data
Synthetic data serves as a powerful tool, possessing similar characteristics to original data while significantly simplifying the model training process. By incorporating synthetic data, one can bypass intricate preprocessing steps and expedite the model training process. Furthermore, generating synthetic data enhances data diversity, ultimately improving the model’s generalization performance.
Do you also aspire to bring about business innovation?
Join us with Cubig!Β