De-identification: The 3 Critical Shortcomings
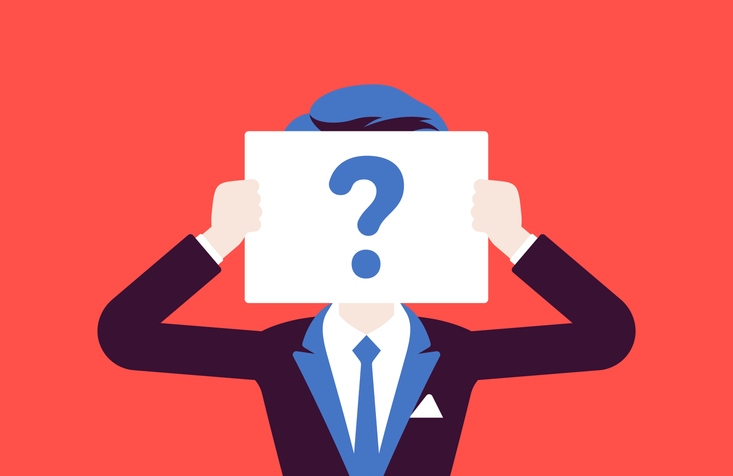
In our data-driven world, the need to protect personal information has become substantially imperative. De-identification has long been touted as the primary means of safeguarding privacy. However, the technique has significant limitations, often failing to provide the robust protection necessary in today’s ever sophisticated AI landscape.
The Illusion of De-identification
De-identification aims to remove or obscure personal identifiers from datasets, theoretically rendering the data safe for use without compromising individual privacy. Nevertheless, the de-identified datasets are still vulnerable to re-identification attacks. It is well demonstrated that by cross-referencing anonymized data with other publicly available datasets, re-identifying individuals is indeed possible with alarming accuracy. This risk undermines the core purpose of the privacy technique, exposing sensitive information and potentially leading to harmful consequences.
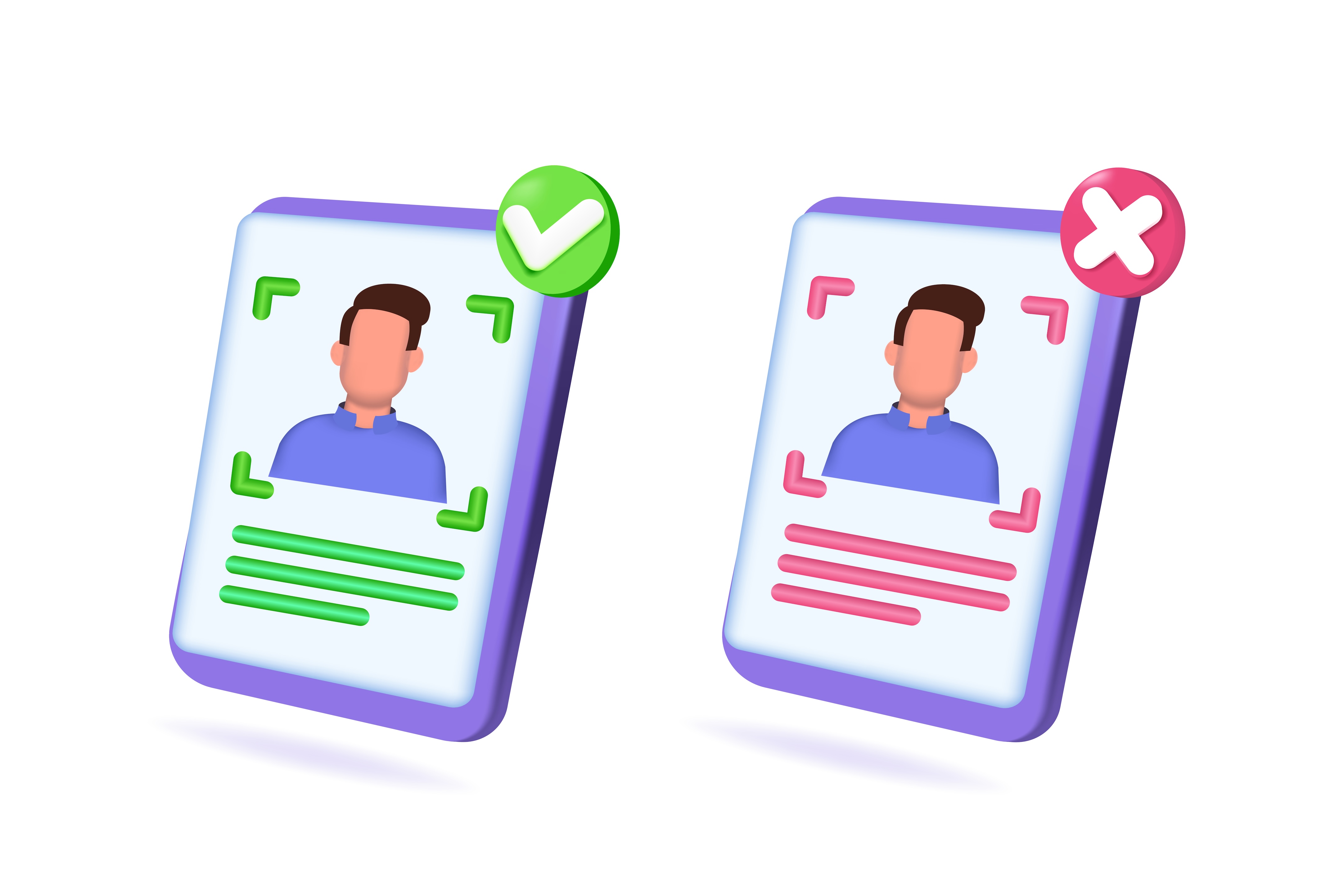
Forbidden to Know, Free to Infer
While de-identification offers weak protection, its scope is also narrow. It usually involves removing obvious identifiers such as names, addresses, and social security numbers. However, even seemingly benign data points can act as quasi-identifiers when combined with other information. Factors like age, gender, and ZIP code, though not inherently unique, can collectively identify specific individuals, especially in small or homogeneous populations.
Dynamic Data Challenges
Another significant shortcoming of de-identification is its vulnerability to dynamic datasets. In environments where data is continuously updated or added, maintaining de-identified status becomes drastically complex. Each new data point can potentially compromise the de-identification, allowing adversaries to reassemble and link information over time. This dynamic nature of data makes the de-identification method insufficient for long-term privacy protection.
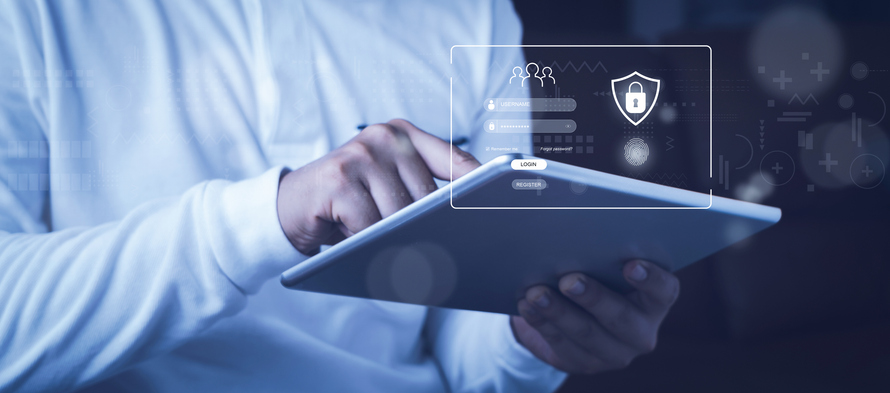
The Promise of Differential Privacy
Given the limitations of the traditional privacy method, differential privacy emerges as a superior alternative. Differential privacy is a mathematical framework that ensures the privacy of individuals within a dataset by introducing a controlled amount of random noise to the data, masking the contribution of any single individual.
Strengths of Differential Privacy
Differential privacy provides a quantifiable measure of privacy protection. Hence, this approach offers much stronger guarantees against re-identification, significantly enhancing the security of personal information. Furthermore, differential privacy is applicable to various types of data and analytics. Whether used on health research, social science studies, or business analytics, differential privacy adapts to different contexts while maintaining robust privacy protections.
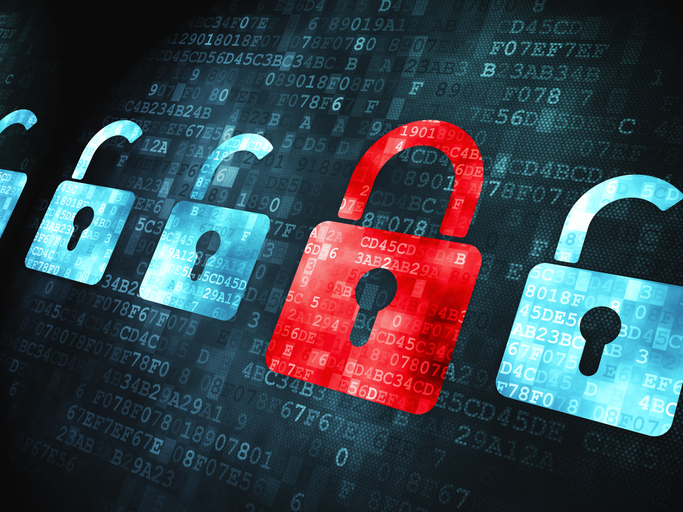
While adding noise to data might seem counterproductive, differential privacy strikes a balance between data utility and privacy. The framework allows for the adjustment of noise levels based on the desired privacy guarantee and the acceptable trade-off in data accuracy. This flexibility enables organizations to derive valuable insights from data without compromising individual privacy.