Discover the Performance: Secure and Utility-Driven Synthetic Data for You! (7/9)
Table of Contents
Nowadays, synthetic data is becoming a popular method for sharing data and training AI without legal issues related to privacy and confidential information. To find really meaningful synthetic data for your project, it is crucial to evaluate if synthetic data is truly useful and safe. Today, we will discuss two primary evaluating methods for assessing the performance of synthetic data: utility evaluation and safety evaluation.
1. Utility Performance Evaluation
Utility evaluation confirms how similar the synthetic data is to the original data and whether it can be used effectively for analysis or AI Training. This includes the following assessment methods:
- Column Distribution Histogram Similarity: This method compares the histograms of each column in the synthetic data to those in the original data to ensure similar column distributions.
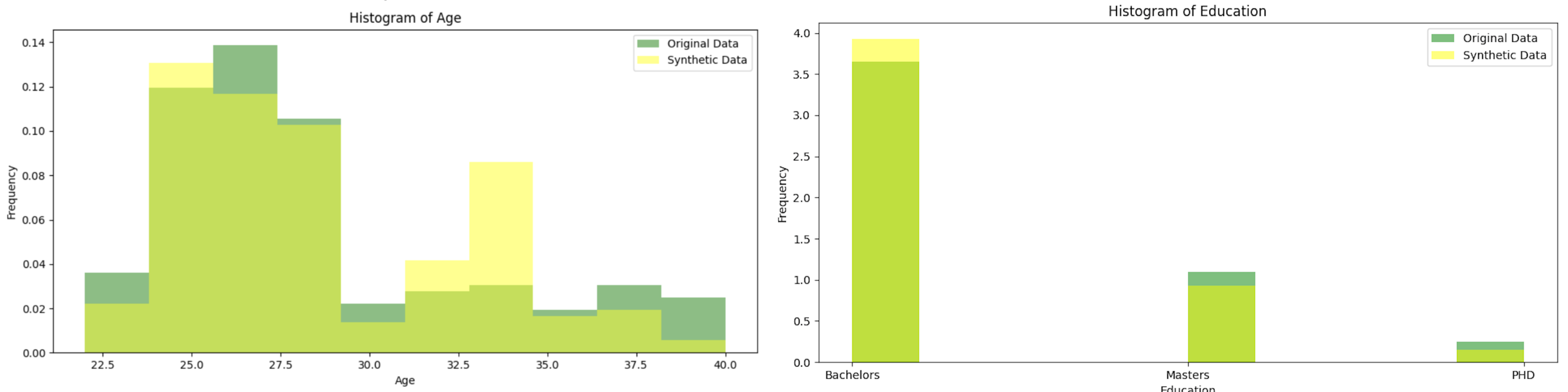
2. Column Correlation Coefficient Similarity: It evaluates how closely the correlations between columns in the synthetic data match those in the original data. (Left figure is for the real data, right figure is for the synthetic data)
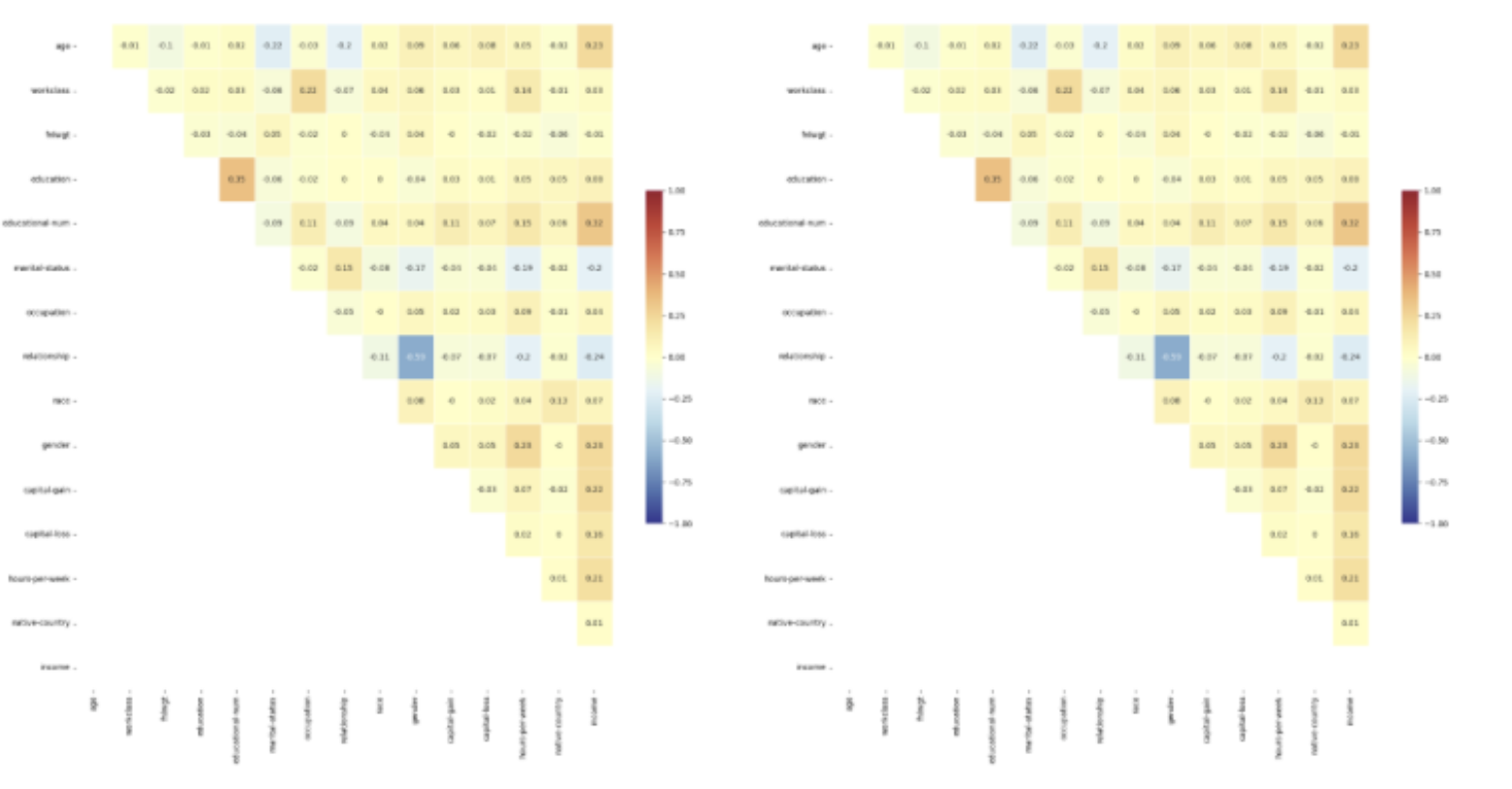
3. Similarity Metrics (KID, Cosine Similarity): Metrics such as Kernel Inception Distance (KID) and cosine similarity measure the overall similarity between the original and synthetic data.
4. Downstream Classification Performance: This assesses the performance of machine learning models trained on synthetic data compared to those trained on original data, using classification tasks.
5. Statistical Value Similarity: This involves comparing basic statistical values (mean, median, standard deviation, etc.) between the original and synthetic data.
6. Data Diversity Evaluation (Entropy based Score): Measures like the entropy based score evaluate the diversity within the synthetic data to ensure it captures the variety present in the original data.
2. Safety Performance Evaluation
Safety evaluation ensures that the synthetic data does not compromise privacy and is indistinguishable from the original data. This includes:
- Non-Identifiability: Ensuring that individual information cannot be identified from the synthetic data.
- Indistinguishability: Evaluating whether it is difficult to distinguish between the original and synthetic data.
At Cubig, we emphasize these evaluation metrics to guarantee the creation of high-quality synthetic data. Our synthetic data meets these standards for both utility and safety, providing users with reliable and secure data for their analytical needs.
Conclusion
Evaluating the performance of synthetic data through utility and safety assessments is essential for ensuring its effectiveness and security. At Cubig, we are dedicated to delivering synthetic data that excels in both areas, enabling users to use data without privacy concerns.
By understanding and applying these evaluation methods, you can ensure that the synthetic data you use or generate maintains high standards of quality and privacy, making it a valuable asset in data analysis, research and AI train!
Azoo
