Diversity Wins: 5 Smart Strategies to Supercharge AI Performance
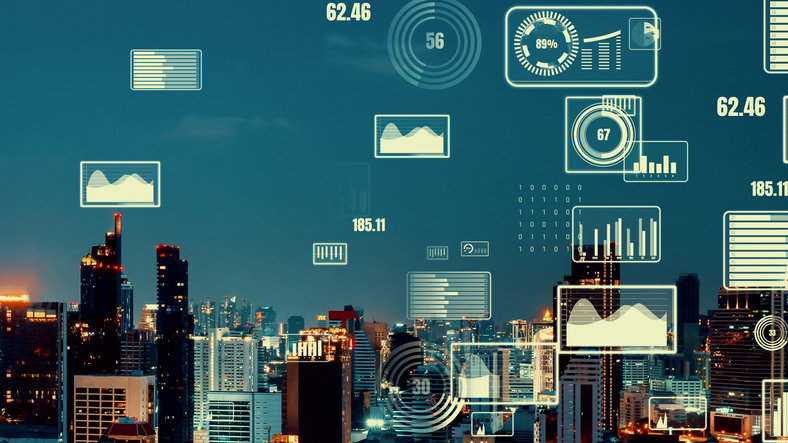
Data diversity is a fundamental aspect of AI development and deployment that is often underscored for its critical importance. As AI systems become drastically embedded in various fields of current society, from healthcare and finance to education and entertainment, the diversity of data used to train these systems is paramount. The diversity is not just a technical requirement but a cornerstone of ethical, effective, and robust AI.
Understanding Data Diversity
You have a high data diversity when you train your AI model on a wide range of data representing various situations, conditions, etc.. For example, when deploying facial recognition AI, including photos of people from different age groups, genders, and ethnicities increases data diversity.
Why Is Diverse Data So Important?
Imagine a person trying to understand a complex topic like climate change. Relying solely on a single source or perspective can lead to a skewed or incomplete understanding. Instead, by exploring multiple sources, including scientific journals, news articles, expert interviews, documentaries, and even different cultural perspectives, the person can gain a more well-rounded and nuanced understanding. This approach helps to identify biases, verify facts, and appreciate the broader context, ultimately leading to more informed and critical thinking.
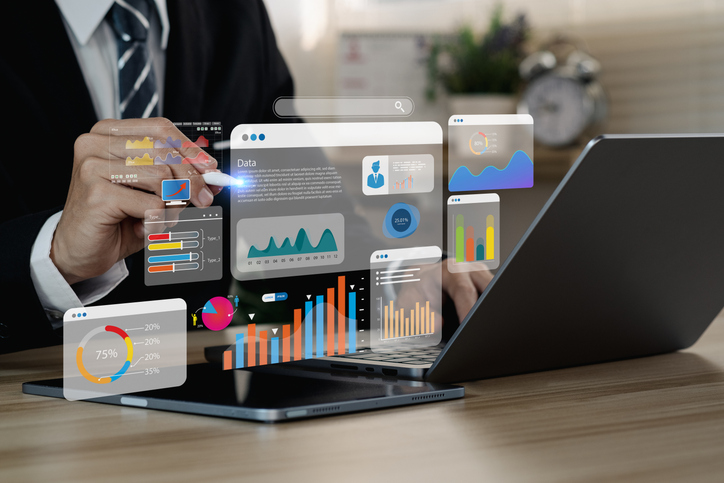
The same principle applies for AI to learn effectively. When training AI models, a diverse and comprehensive dataset is crucial in order to make more accurate predictions in various situations.
If AI is trained on a dataset with a limited variety, it might work well for certain groups but poorly for others. For example, if an AI is trained mostly on male voices, it might struggle to recognize female voices. Data diversity helps prevent AI from being biased towards specific groups.
Diverse Data Not More Data
Gaining many data points is not necessarily equivalent to increasing diversity. While having a large dataset is beneficial for training AI models, the quality and diversity of the data are equally, if not more, important. Here’s why:
Quality Over Quantity
A large dataset with redundant or similar data points might not provide new information to the model. Diversity ensures that each data point contributes unique information, enhancing the learning process.
Bias Reduction
A large dataset can still be biased if it predominantly represents certain groups, scenarios, or perspectives. Increasing diversity helps mitigate these biases by ensuring that underrepresented groups and scenarios are included.
Generalization
AI models trained on diverse data are better at generalizing to new, unseen data. If the training data covers a wide range of scenarios, the model is more likely to perform well in various real-world situations.
Robustness
Diversity in data helps in building robust models that can handle different conditions and anomalies. For example, a facial recognition system trained on images from various lighting conditions, angles, and ethnic backgrounds will be more robust than one trained on a large dataset of similar images.
Inclusivity and Fairness
Diverse data ensures that the AI system is fair and inclusive, reducing the risk of discrimination against any particular group. For instance, a voice recognition system should include voices of different genders, ages, and accents to be fair and effective for all users.
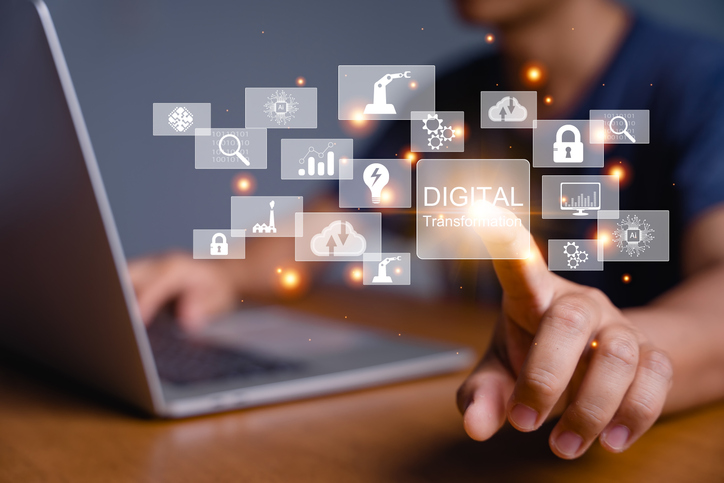
Strategies to Increase Data Diversity
Collect Data from Various Sources
Gather data from multiple sources such as social media, news, blogs, and government databases to include a wide range of content.
Broaden Data Collection Criteria
Ensure that data collection includes all groups and situations by considering factors like age, gender, ethnicity, and geographic location.
Secure Data from Minority Groups
Intentionally gather more data from underrepresented groups or scenarios, such as anomalies, is a crucial strategy for enhancing the diversity and effectiveness of AI models. This improves the model’s robustness and reliability.
Use Data Augmentation
Data augmentation involves modifying existing data to create new samples. For example, rotating, scaling, and changing colors of images can generate diverse data.
Generate Synthetic Data
When it’s hard to collect data from all possible scenarios, synthetic data can be created. Synthetic data is artificially generated rather than collected from real-world events. This data can be created using various techniques, including simulations, computer-generated models, and more advanced algorithms than data augmentation. It generates data entirely without basis in real-world data while derivative data is created from existing real-world data in data augmentation.
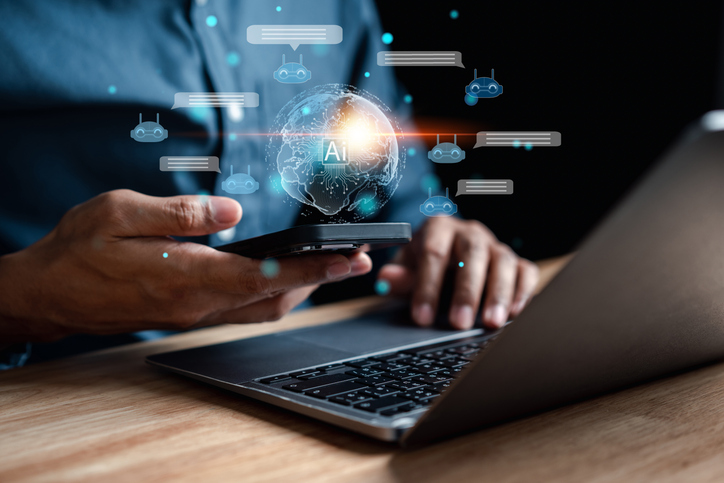