Good in Lab, Poor in Reality: How to Overcome Domain Shift (4/1)
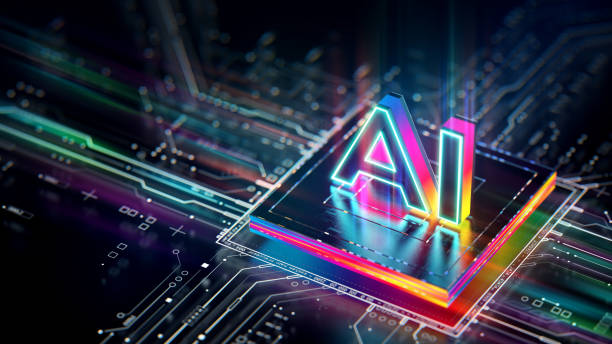
Always Mind the Domain Shift
AI is one of the key techniques in this century that is considered to change our lives utterly. But such a complex technology as AI may not always behave as one might expect. one phenomenon continues to perplex even the most seasoned data scientists and AI practitioners: the challenge of successfully transitioning a well-performing AI model from the training phase to real-world application. Despite leveraging advanced techniques like k-fold cross-validation to ensure model robustness and employing data augmentation methods to enhance dataset variability, there remains a gap in performance when AI models are deployed in actual products. In most cases, this is because of domain shift.
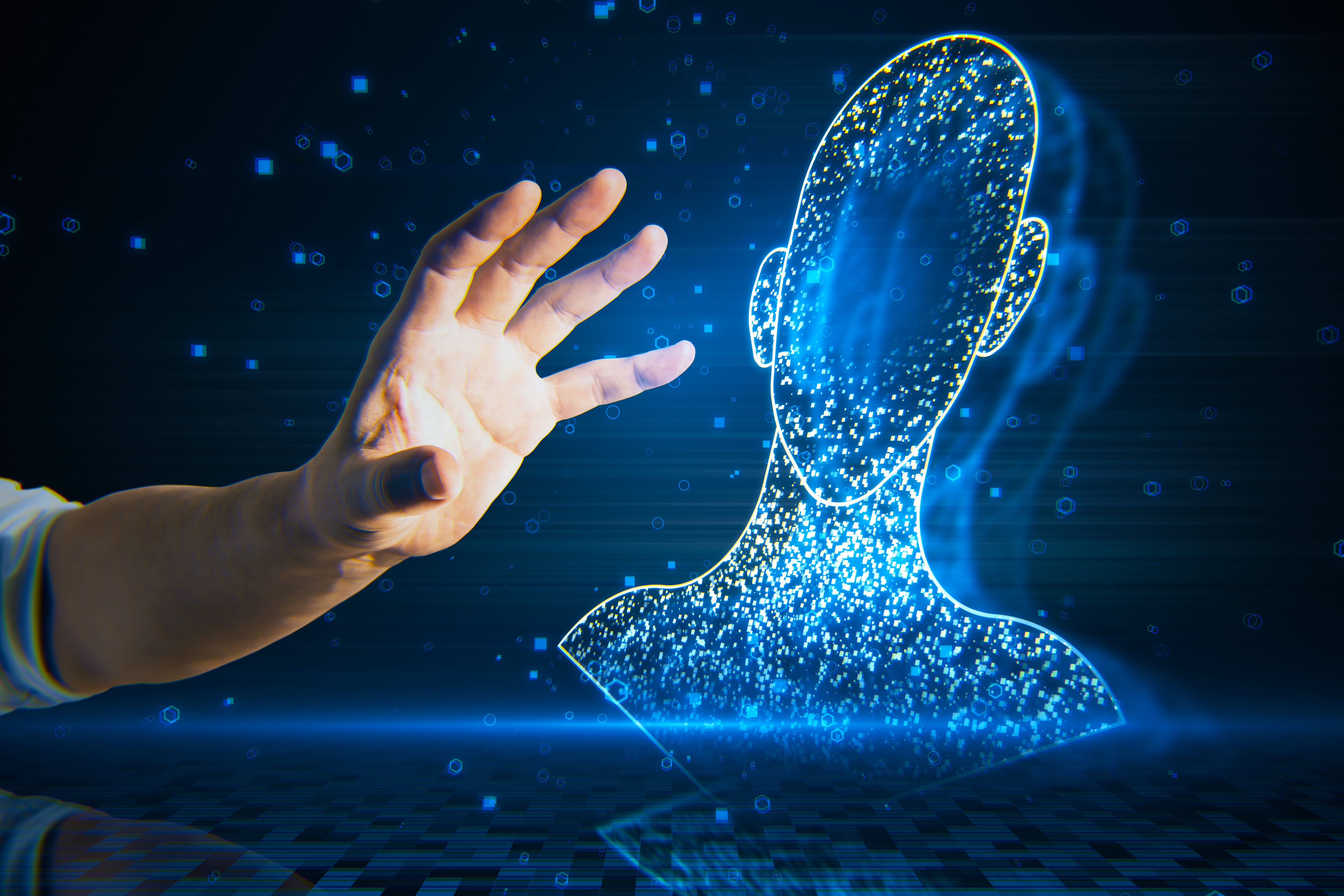
What is Domain Shift?
Domain shift refers to the discrepancy between the data distribution a model was trained on and the distribution it encounters in real-world applications. Those distributions differ from each other due to various factors, such as environmental conditions, sensor qualities, or simply the unpredictability of human behavior. Therefore the model struggles to adapt to the new, unseen data it faces outside the controlled training environment, leading to a decline in performance.
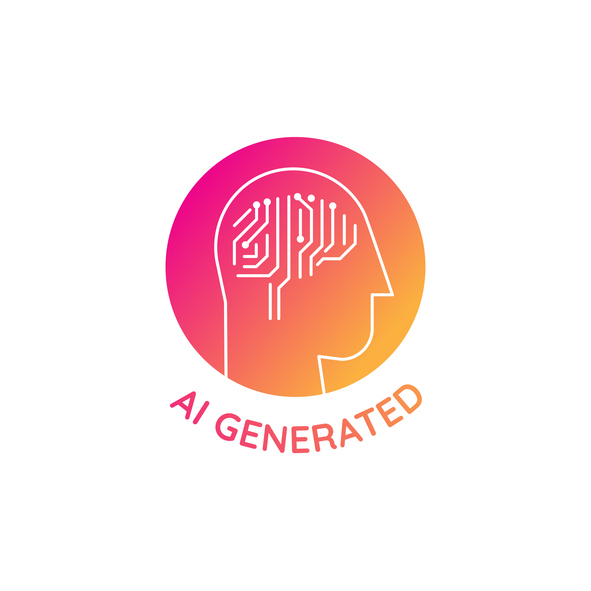
Synthetic Data as Intermediate Domains
This is where synthetic data emerges as a promising solution to bridge the gap between training environments and real-world application. Synthetic data is artificially generated information that simulates the characteristics of real-world data, offering a controlled yet versatile means of expanding the diversity of training datasets. By using synthetic data as a series of intermediate domains that gradually mitigate the gap, AI developers can preemptively expose their models to a wider range of experiences, thereby equipping them with the flexibility needed to adapt to domain shifts.
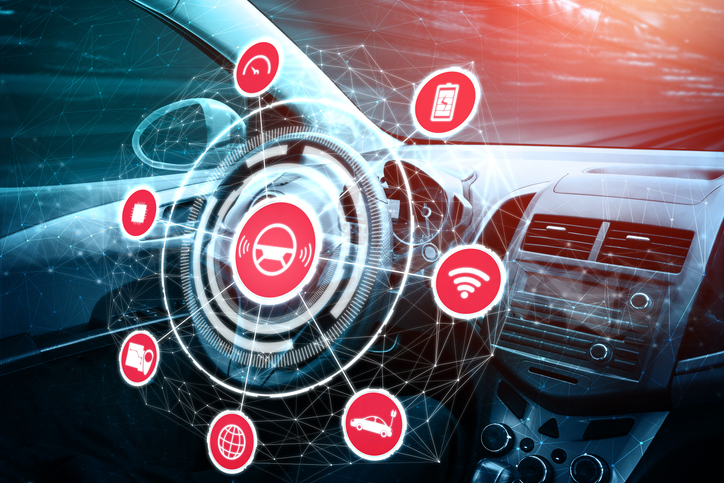
Can We Trust Synthetic Data?
The answer is yes. Synthetic data has various advantages. Developers can not only generate as much data as they want, but also obtain more variety, higher quality, and safer privacy.