Trajectory (GPS) Synthetic Data Generation: A Solution for Enhancing Both Privacy and Utility (8/13)
Table of Contents
Synthetic Data Generation
1. Introduction
In recent years, the advancement of Location-Based Services (LBS) has led to the emergence of various applications utilizing GPS data. GPS data plays a crucial role in fields such as autonomous vehicles, smart cities, logistics optimization, and sports analysis. Additionally, this data is vital for mobility analysis. However, can we freely analyze this data and use it for AI training? Absolutely not. This data includes personal location information and individual trajectories, and using it without consideration for privacy can lead to serious privacy breaches. So, should we abandon this data? As mentioned, trajectory (GPS) data is too valuable and can be used in many beneficial applications.
To address these issues, Synthetic Trajectory data has gained attention. This data maintains the utility and statistical properties of real trajectory data while ensuring that sensitive information is not disclosed.
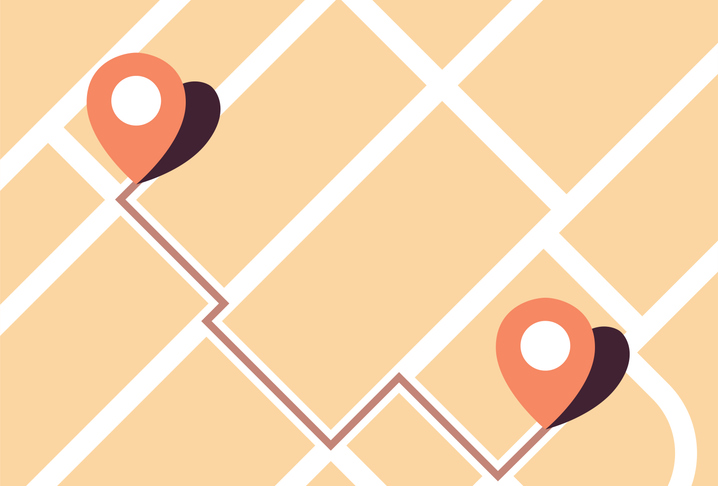
2. What is Synthetic Trajectory Data?
Synthetic GPS data is artificially generated based on real data but does not include actual user location information. This approach protects user privacy while retaining the data’s utility. Synthetic data is typically generated using learning techniques such as Diffusion Models, Generative Adversarial Networks (GANs), and Variational Autoencoders (VAEs).
3. Advantages of Synthetic Data for Privacy Protection
3.1. Privacy Protection
Synthetic data does not include actual user location information, effectively safeguarding individual privacy.
3.2. Maintaining Data Utility
Synthetic data retains similar characteristics to real data, making it useful for data analysis, model training, and various applications.
3.3. Solving Data Scarcity
When it is challenging to collect real data, synthetic data can provide the necessary data for various needs.
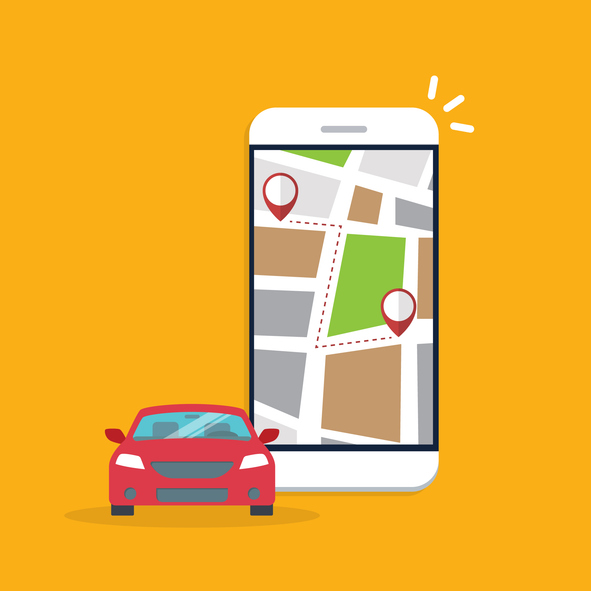
4. Limitations and Solutions of Synthetic Data
Despite synthetic data not directly including user data, it may still contain some properties of the original data. In some cases, this can lead to the possibility of reconstructing the original data. To mitigate this issue, Differential Privacy techniques are applied during the synthetic data generation process.
Differential Privacy involves adding random noise to the data to ensure that individual data points are not identifiable. This technique helps protect sensitive information while maintaining the utility of the synthetic data.
5. Applications of Synthetic Trajectory Data
5.1. Autonomous Vehicles ( Synthetic Data Generation in Autonomous Vehicles)
Autonomous vehicles require large amounts of GPS data for route planning and environmental perception. By using synthetic data, it is possible to obtain sufficient data without compromising privacy.
5.2. Smart Cities
In smart cities, synthetic data can be used for urban traffic analysis and optimizing public transportation systems, protecting residents’ location information while enabling effective city management.
5.3. Healthcare and Fitness
In healthcare and fitness, synthetic data can be used for tracking exercise routes and analyzing activities without exposing individual location information.
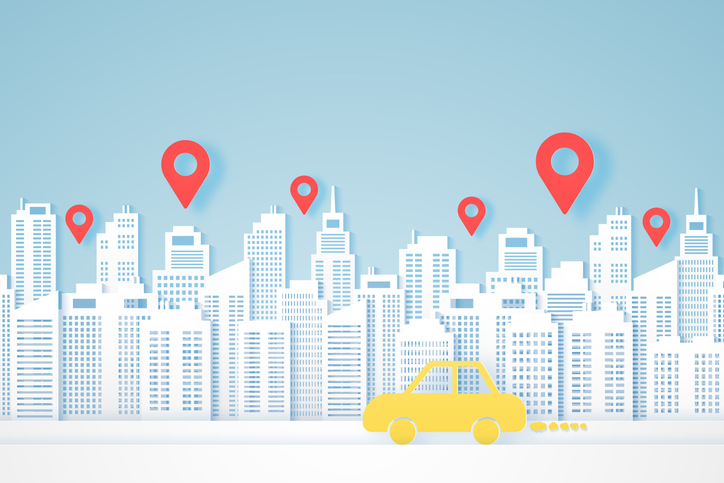
Conclusion
Synthetic GPS data offers an innovative solution that balances privacy protection and data utility. Various machine learning techniques can generate data that closely resembles real data, enabling privacy protection while providing valuable insights in multiple application areas. Techniques such as GANs, VAEs, and Diffusion Models are particularly effective in creating highly realistic synthetic data. Moreover, by applying Differential Privacy, the risk of reconstructing original data is minimized, allowing for safer data usage. The development and application potential of synthetic data are expected to grow further in the future.
Are you curious about creating useful synthetic data securely with differential privacy? Cubic Inc. utilizes differential privacy to generate highly secure synthetic data, achieving up to 99% similarity with the original data. If you’re interested, click the link below:
- CUBIG: https://cubig.ai/
- Platform for creating and trading synthetic data: https://azoo.ai/
- This platform supports the generation of synthetic data in various fields, not just synthetic trajectory data, tailored to your needs.