Insurance Transformed: 5 Powerful Benefits of Synthetic Data
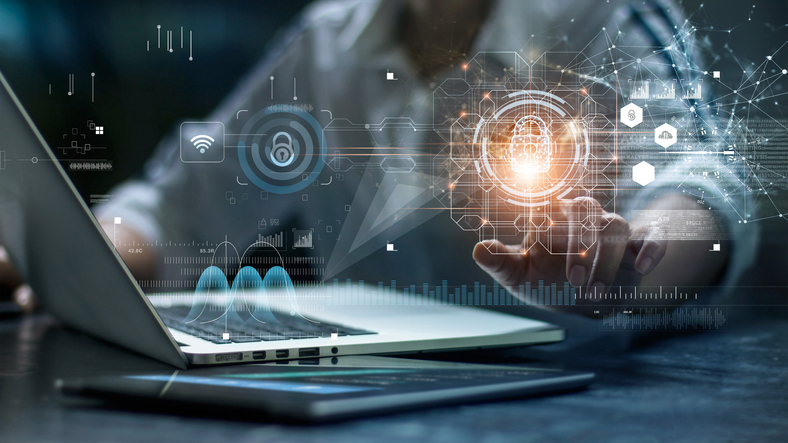
Insurance is undergoing a transformative shift in the data-driven era, with synthetic data emerging as a game-changer. Synthetic data, crafted by algorithms to simulate real-world data accurately, stands poised to revolutionize various features of the insurance industry. From enhancing risk assessment and detecting fraud to refining customer service and driving product innovation, synthetic data offers compelling solutions to contemporary challenges faced by insurers. This blog delves into the applications, benefits, and promising future of synthetic data in reshaping the landscape of insurance.
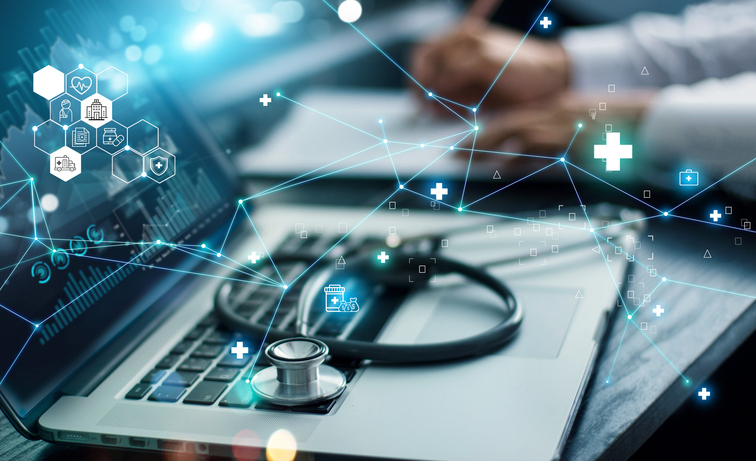
Understanding Synthetic Data
Synthetic data is created using sophisticated algorithms that replicate the statistical properties of real-world data. This process involves techniques such as Generative Adversarial Networks (GANs), Variational Autoencoders (VAEs), and other machine learning methods. The result is a dataset that closely resembles real data in terms of structure and distribution, but without containing any actual personal information.
Applications of Synthetic Data in Insurance
The insurance industry relies heavily on data for various functions, including underwriting, claims processing, risk management, and fraud detection. Hereβs how synthetic data can be applied in these areas:
1. Underwriting and Risk Assessment
Underwriting is a critical process in the insurance industry, where insurers evaluate the risk associated with a potential policyholder. Traditional underwriting relies on historical data, which may not always be sufficient or current. Synthetic data can enhance underwriting by:
- Expanding Data Availability: Synthetic data can augment real-world data, providing a more comprehensive dataset for analysis. This is particularly useful in cases where historical data is limited or outdated.
- Improving Risk Models: With synthetic data, insurers can simulate various scenarios and stress-test their risk models. This leads to more accurate risk assessments and better pricing strategies.
- Ensuring Privacy Compliance: Using synthetic data allows insurers to perform underwriting analysis without exposing sensitive personal information, thereby ensuring compliance with data protection regulations.
2. Fraud Detection
One of the most significant concern in the insurance is fraud, leading to substantial financial losses each year. Synthetic data can enhance fraud detection efforts in several ways:
- Training Machine Learning Models: High-quality synthetic data can be used to train machine learning models for fraud detection. These models can learn to identify patterns and anomalies that indicate fraudulent activities.
- Simulating Fraud Scenarios: Insurers can create synthetic datasets that include various types of fraud scenarios. This helps in developing and testing detection algorithms that are more robust and effective.
- Protecting Real Data: By using synthetic data for training and testing, insurers can safeguard real customer data, reducing the risk of data breaches.
3. Customer Service and Personalization
In todayβs competitive market, providing excellent customer service and personalized experiences is crucial for insurers. Synthetic data can play a vital role in this area by:
- Creating Customer Profiles: Insurers can generate synthetic customer profiles that reflect the diversity of their customer base. This helps in designing personalized insurance products and services that meet specific needs.
- Enhancing Chatbots and Virtual Assistants: Synthetic data can be used to train AI-powered chatbots and virtual assistants, enabling them to handle a wide range of customer queries effectively.
- Testing Customer Interaction Scenarios: Insurers can use synthetic data to simulate various customer interaction scenarios, allowing them to optimize their customer service strategies and improve overall customer satisfaction.
4. Product Development and Innovation
The ability to innovate and develop new insurance products is essential for staying competitive. Synthetic data can support product development in the following ways:
- Market Analysis: Synthetic data can be used to conduct market analysis and identify emerging trends. This helps insurers to develop products that cater to evolving customer needs.
- Rapid Prototyping: Insurers can generate synthetic datasets to test new product ideas quickly. This accelerates the development process and reduces time-to-market.
- Scenario Analysis: By simulating different market conditions and customer behaviors, insurers can evaluate the potential success of new products before launching them.
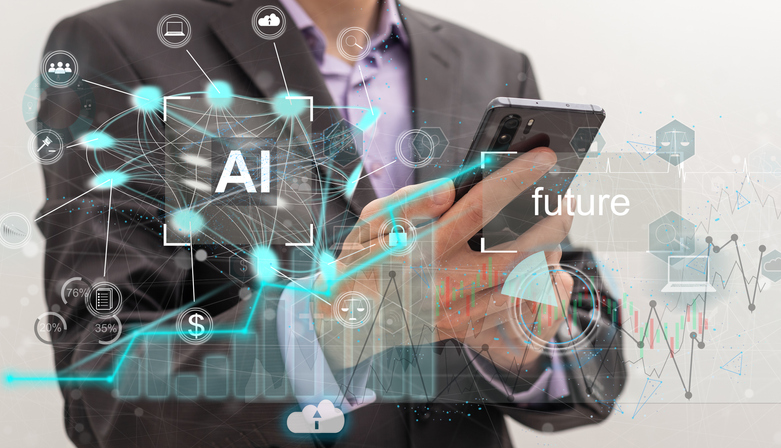
Benefits of Synthetic Data in Insurance
1. Enhanced Data Privacy
Synthetic data plays a pivotal role in safeguarding sensitive information. By generating data that mimics real-world scenarios without containing actual personal data, insurers can mitigate the risk of data breaches and ensure compliance with stringent data protection regulations like GDPR and CCPA.
2. Improved Model Accuracy
By augmenting existing datasets, synthetic data enriches the training of machine learning models. This enhancement translates to higher accuracy and reliability in predictive models crucial for tasks such as underwriting, fraud detection, and customer service.
3. Cost Efficiency
Unlike traditional methods that involve costly and time-consuming data collection and labeling, synthetic data generation can be automated and scaled. This efficiency is particularly beneficial for smaller insurance companies looking to reduce expenses associated with data acquisition.
4. Accelerated Innovation
Synthetic data empowers insurers to innovate swiftly by enabling rapid testing of new ideas and products. Through simulations of diverse scenarios and market conditions, insurers can identify promising opportunities and expedite the launch of innovative products.
5. Flexibility and Scalability
Customizable to meet specific needs and scalable for generating large datasets, synthetic data provides flexibility in adapting to evolving data requirements. This adaptability enables insurers to explore new avenues of analysis and research.
Challenges and Future Prospects
While the benefits of synthetic data are clear, challenges remain:
1. Quality and Realism
Ensuring synthetic data accurately reflects real-world complexities is crucial for maintaining the reliability of models and informed decision-making. Continuous advancements in data generation techniques are essential to enhance the quality and realism of synthetic data.
2. Acceptance and Trust
Building trust in synthetic data among stakeholders is imperative. Effective communication about its benefits and limitations, along with transparent practices in its deployment, is key to gaining acceptance from regulators, customers, and internal teams.
3. Ethical Considerations
Upholding ethical standards in synthetic data usage is paramount. Insurers must ensure fairness and guard against perpetuating biases or discrimination. Establishing clear ethical guidelines and best practices is essential to uphold integrity in synthetic data applications.
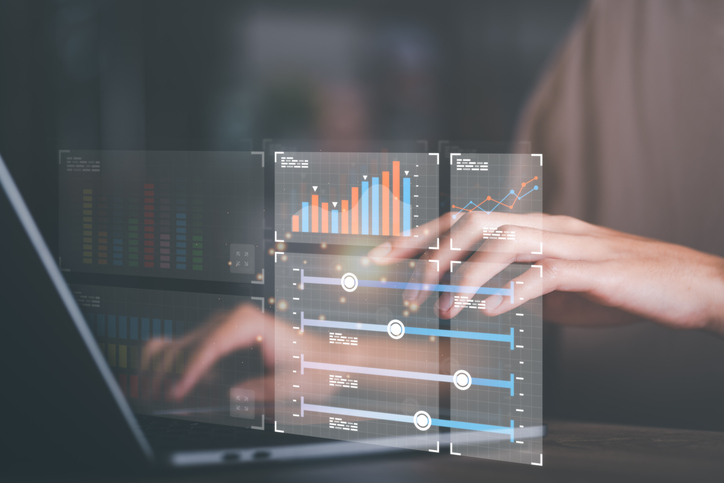